도입
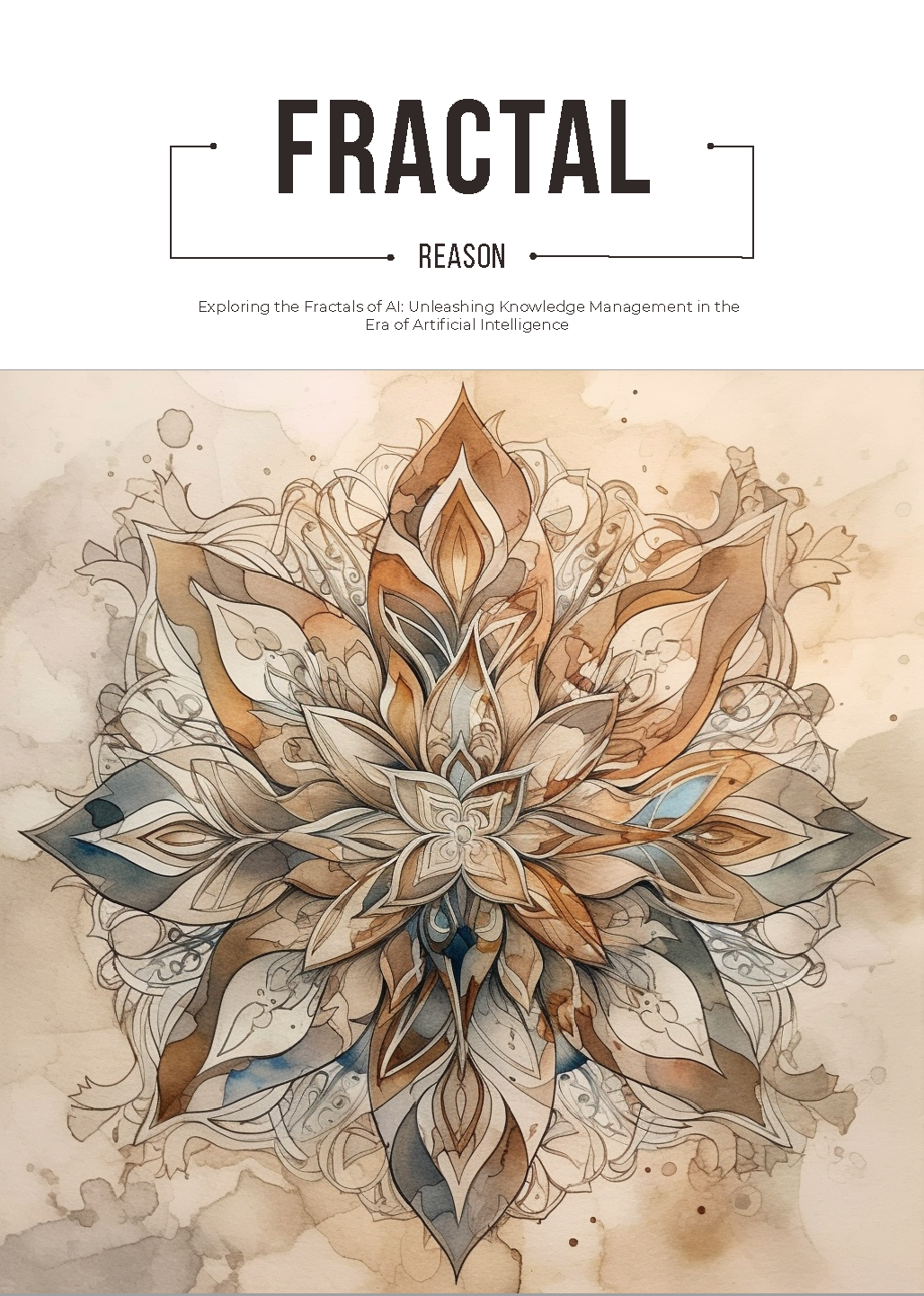
# Exploring the Fractals of AI: Unleashing Knowledge Management in the Era of Artificial Intelligence
## 1. Introduction
In "Exploring the Fractals of AI: Unleashing Knowledge Management in the Era of Artificial Intelligence", this book delves into the fascinating world of fractals and their connection to knowledge management in the era of AI. It explores how the intricate patterns found in fractals can be applied to AI technologies, unlocking the potential for more efficient knowledge management. By harnessing the power of fractals, organizations can better organize, access, and utilize their vast amounts of data and information, leading to enhanced decision-making and innovation. This book serves as a comprehensive guide for individuals and businesses looking to leverage the fractal nature of AI to optimize knowledge management practices and gain a competitive edge.
Throughout the book, readers will embark on a journey that intertwines the concepts of fractals, AI, and knowledge management. The book begins by laying the groundwork of understanding fractals, exploring their characteristics and the mathematics behind them. It then delves into the transformative capabilities of AI and its impact on knowledge management, highlighting the challenges and opportunities that arise in this rapidly evolving landscape. The book provides practical insights, strategies, and case studies, illustrating how organizations can leverage AI-powered knowledge management systems to streamline processes, enhance collaboration, and drive innovation. By embracing the fractals of AI, businesses can unlock the true potential of their knowledge and propel themselves into the future.
"Exploring the Fractals of AI: Unleashing Knowledge Management in the Era of Artificial Intelligence" is a must-read for anyone interested in the intersection of fractals, AI, and knowledge management. Whether you are an AI enthusiast, a knowledge management professional, or a business leader looking to harness the power of data, this book offers a unique perspective on how fractal patterns can revolutionize knowledge management practices. Through engaging explanations, real-world examples, and practical guidance, this book equips readers with the knowledge and tools necessary to navigate the dynamic world of AI-driven knowledge management and unlock its immense potential.
### 1.1 The Concept of Fractals
Fractals are complex geometric shapes or patterns that exhibit self-similarity at various scales. They are characterized by their intricate details, infinite complexity, and the repetition of similar patterns regardless of the level of magnification. The concept of fractals was introduced by the mathematician Benoit Mandelbrot in the 1970s, revolutionizing our understanding of shape and structure in the natural world.
Fractals can be found in many natural phenomena, such as coastlines, clouds, mountains, and even in the branching patterns of trees. These intricate patterns have also captured the attention of artists and scientists alike, inspiring awe and fascination.
In the context of artificial intelligence (AI) and knowledge management, the concept of fractals offers valuable insights into the underlying structure and organization of information. Just as fractals exhibit self-similarity, AI and knowledge management systems aim to capture, organize, and leverage knowledge in a way that enables effective decision-making and problem-solving.
The study of fractals in AI and knowledge management can help us understand the complexity and interconnections within data, uncover hidden patterns, and extract valuable insights. By applying fractal-based techniques, AI systems can navigate through the vast and intricate web of information, identifying relevant patterns and relationships, and providing meaningful knowledge.
Fractals offer a new lens through which we can view knowledge and its organization. Traditional methods of knowledge management often focus on hierarchical structures and linear relationships. However, fractal-based knowledge management takes a different approach, recognizing the inherent complexity and non-linear nature of knowledge.
Just as fractals exhibit self-similarity across scales, knowledge also exhibits similarity and interconnections across different domains, disciplines, and industries. Fractal-based knowledge management approaches enable the identification and utilization of these cross-domain relationships, allowing for a holistic understanding and application of knowledge.
In the era of AI, the need for effective knowledge management becomes even more crucial. The rapid advancement of AI technologies has led to an explosion of data and information. Without proper knowledge management, organizations risk drowning in this sea of data, unable to derive meaningful insights or make informed decisions.
AI systems rely heavily on the availability of high-quality and relevant knowledge. The integration of fractal-based approaches in knowledge management can enhance the efficiency and effectiveness of AI systems by providing a scalable and adaptable framework for knowledge acquisition, organization, sharing, and application.
Fractal-based knowledge management allows for the representation of knowledge in a flexible and interconnected manner, breaking away from rigid hierarchical structures. It enables the identification of key knowledge components, the discovery of hidden relationships, and the adaptation of knowledge to changing contexts. This adaptability is crucial in the dynamic and evolving landscape of AI.
Moreover, fractal-based knowledge management facilitates serendipitous discovery and innovation. Just as fractals exhibit unpredictable and emergent properties, the interconnected nature of knowledge in a fractal-based system can lead to novel insights and unexpected connections. This can drive creativity and spark innovation in AI systems and beyond.
By embracing the principles of fractals in knowledge management, organizations can unleash the full potential of AI. Fractal-driven knowledge management enables the creation of knowledge ecosystems that foster collaboration, information sharing, and interdisciplinary approaches. It breaks down silos and encourages the exchange of ideas, leading to a more holistic and comprehensive understanding of complex problems.
In conclusion, the concept of fractals offers a fresh perspective on knowledge management in the era of AI. Fractal-based approaches provide a powerful framework for capturing, organizing, and leveraging knowledge, enabling AI systems to navigate the complexity of information and uncover valuable insights. By embracing fractals, organizations can unlock the full potential of AI and propel innovation and decision-making to new heights.>)
### 1.2 The Significance of Knowledge Management
In the era of Artificial Intelligence (AI), the significance of knowledge management has never been more crucial. As AI continues to advance and reshape various industries, organizations are faced with the challenge of harnessing and leveraging the vast amount of knowledge and information available to them. This is where knowledge management plays a pivotal role.
Understanding Knowledge Management
Before delving into its significance, let's first define what knowledge management entails. Knowledge management refers to the process of identifying, capturing, organizing, and utilizing an organization's collective knowledge to enhance performance, foster innovation, and facilitate effective decision-making.
In any organization, knowledge is a valuable asset that underpins its success. It encompasses explicit knowledge, which is codified and can be easily documented, and tacit knowledge, which resides in the minds of individuals and is often difficult to articulate. Knowledge management aims to unlock and harness both types of knowledge, ensuring that they are shared, stored, and distributed effectively throughout the organization.
The Role of Knowledge Management in AI
As AI technologies continue to evolve, organizations are increasingly relying on these systems to perform complex tasks, make accurate predictions, and enhance productivity. However, the success of AI systems lies not only in their ability to process and analyze data but also in their access to relevant knowledge.
Knowledge management provides the foundation upon which AI systems can operate effectively. By effectively managing knowledge, organizations can curate high-quality datasets, ensure the accuracy of training data, and establish robust knowledge repositories that serve as the backbone of AI applications.
Knowledge Creation and Acquisition
One of the primary functions of knowledge management is the creation and acquisition of knowledge. With the exponential growth of data, organizations must have mechanisms in place to identify, extract, and validate relevant knowledge from various sources. This process involves data collection, synthesis, and transformation into actionable insights that can be utilized by AI systems.
Organizations can leverage AI techniques such as natural language processing (NLP), machine learning, and data mining to automate knowledge creation and acquisition processes. These AI-driven tools enable the extraction of valuable information from unstructured data sources and assist in the identification of patterns and trends that may not be easily discernible to human operators.
Knowledge Storage and Retrieval
Once knowledge is created, it needs to be appropriately stored and easily accessible for future use. This is where knowledge management systems play a vital role. By implementing robust storage structures, organizations can ensure that valuable knowledge is preserved and readily available to AI systems and human users alike.
AI techniques such as knowledge graphs and semantic technologies can enhance knowledge storage and retrieval processes by creating meaningful associations between different pieces of information. By organizing knowledge in a structured and interconnected manner, organizations can optimize search and retrieval operations, enabling more efficient access to relevant knowledge.
Knowledge Sharing and Collaboration
In the dynamic and interconnected world of AI, knowledge sharing and collaboration are essential for both human operators and AI systems. By facilitating knowledge sharing, organizations can foster a culture of collaboration and innovation, allowing individuals and AI systems to learn from each other's experiences and expertise.
Knowledge management systems can provide collaborative platforms that enable real-time sharing of information, insights, and best practices. Through these platforms, human operators and AI systems can engage in a symbiotic relationship, continuously learning from and contributing to the organization's collective knowledge.
Knowledge Transfer and Application
For organizations to fully reap the benefits of AI, effective knowledge transfer and application are critical. Knowledge management systems play a crucial role in facilitating the transfer of knowledge from AI systems to human operators and vice versa. This ensures that the knowledge generated by AI is translated into practical insights that can be applied to decision-making and problem-solving processes.
Furthermore, knowledge management systems enable the application of acquired knowledge across different contexts and domains. By leveraging the insights gained from AI systems, organizations can enhance their operations, improve customer experiences, and drive innovation in their respective industries.
Conclusion
In the era of AI, knowledge management is indispensable for effectively leveraging the power of artificial intelligence. It provides the infrastructure and processes necessary to create, store, retrieve, share, transfer, and apply knowledge, ensuring that organizations maximize the potential of their AI systems. By embracing knowledge management practices, organizations can unlock the true value of their knowledge assets, drive innovation, and gain a competitive edge in the rapidly evolving AI landscape.>)
### 1.3 Fractals and Knowledge Management in the AI Era
In the realm of artificial intelligence (AI), the utilization of fractals has opened new avenues for exploring and enhancing knowledge management processes. Fractals, with their inherent self-similar patterns and complexity, have found relevance in various fields, including mathematics, science, and aesthetics. Now, their application in AI has the potential to revolutionize knowledge management by providing a deeper understanding of complex systems, facilitating data analysis, and improving decision-making processes.
Fractal Geometry and its Significance
Fractal geometry, pioneered by mathematician Benoit Mandelbrot in the late 1970s, offers a unique framework for understanding complex and irregular structures that cannot be described by traditional Euclidean geometry. Fractals are self-replicating patterns that exhibit similar characteristics at different scales, unveiling intricate details and hidden patterns within complex systems. From the branching patterns of trees to the jagged coastline of a shoreline, fractals are ubiquitous in nature and hold valuable insights into the structure and behavior of various entities.
The Power of Fractals in Artificial Intelligence
Fractals provide a powerful lens through which AI systems can gain a better understanding of the data they work with. By incorporating fractal analysis techniques into AI algorithms, researchers and developers can extract meaningful patterns and make sense of the vast and often chaotic information available. Fractal-based approaches enable AI to identify hidden relationships, classify data more accurately, and generate valuable insights, thus enhancing knowledge management capabilities in the AI era.
Fractals in Data Analysis and Visualization
AI-powered systems deal with vast amounts of complex and multidimensional data. Fractal analysis techniques allow for efficient data analysis, helping to uncover underlying structures and patterns that traditional statistical methods may overlook. By visualizing fractal patterns, AI systems can present data in a more intuitive and interpretable manner, enabling users to gain a deeper understanding of the information at hand. This aids in knowledge discovery and facilitates effective decision-making.
Fractals in Pattern Recognition and Prediction
One of the key aspects of knowledge management is understanding patterns and predicting future trends. Fractals offer a unique advantage in this regard, as their self-similarity allows AI algorithms to detect recurring patterns at various scales. This ability lends itself to pattern recognition tasks and predictive analytics, enabling AI systems to identify complex trends and potential anomalies. By leveraging fractal insights, knowledge management systems can enhance their accuracy, efficiency, and ability to make informed recommendations.
Fractals in Simulating Complexity
Complex systems often exhibit fractal patterns that reflect the interplay between their various components. AI systems can harness this property by using fractal-based simulations to model and understand intricate phenomena. Whether it's simulating the behavior of financial markets, the spread of diseases, or the dynamics of social networks, fractal-based simulations provide a dynamic and adaptive framework for exploring complex systems. This aids in knowledge acquisition, allowing AI systems to capture the underlying dynamics of real-world phenomena and support decision-making processes.
Knowledge Management in the AI Era
Knowledge management plays a pivotal role in harnessing and leveraging organizational knowledge for decision-making and innovation. The advent of AI has brought about a paradigm shift in knowledge management practices, enabling more efficient and effective management of vast amounts of data and information.
AI-driven knowledge management systems leverage advanced techniques such as machine learning, natural language processing, and computer vision to organize, retrieve, and apply knowledge in novel ways. These systems have the potential to revolutionize knowledge management by automating knowledge creation, discovery, and application processes.
Automation and Acceleration of Knowledge Processes
AI technology can automate and accelerate various knowledge management processes. Through machine learning algorithms, AI systems can automatically mine large datasets, extract relevant information, and categorize it according to predefined taxonomies. This automation saves time and enables organizations to efficiently leverage their knowledge assets for decision-making and problem-solving.
Enhancing Knowledge Discovery and Collaboration
With the increasing complexity and volume of data, traditional manual methods of knowledge discovery and collaboration are becoming inadequate. AI-powered knowledge management systems, incorporating fractal-derived insights, enable more advanced and efficient methods of knowledge discovery. By automatically identifying patterns, relationships, and trends, AI systems facilitate the identification of relevant knowledge sources, recommend experts for collaboration, and support the creation of new knowledge.
Enabling Adaptive Knowledge Repositories
In the AI era, knowledge management systems are evolving from static repositories to adaptive and intelligent knowledge systems. By leveraging fractal insights, these systems can adaptively organize and update knowledge repositories based on dynamic patterns and emerging trends. They can identify and fill knowledge gaps, alert users to relevant information, and provide personalized recommendations based on individual user preferences and needs. This adaptability enhances the usability and effectiveness of knowledge repositories, enabling organizations to harness their knowledge for innovation and competitive advantage.
Preserving Explicit and Tacit Knowledge
AI-driven knowledge management systems not only automate the management of explicit knowledge (i.e., information that can be codified and stored), but they also provide mechanisms for capturing and preserving tacit knowledge (i.e., knowledge embedded in personal experiences and expertise). Through natural language processing and sentiment analysis techniques, AI systems can extract valuable tacit knowledge from unstructured data sources such as documents, emails, and chat logs. This ensures that critical knowledge is not lost when employees retire or leave the organization, thus facilitating seamless knowledge transfer and continuity.
In the AI era, the fusion of fractals and knowledge management holds immense promise for organizations seeking to effectively utilize and manage their vast knowledge resources. By leveraging the self-similar and complex nature of fractals, AI systems can enhance knowledge discovery, analysis, and dissemination processes. With AI-driven knowledge management platforms becoming increasingly intelligent and adaptive, organizations are poised to unlock new insights, make better decisions, and leverage their knowledge for innovation and competitiveness.
### 1.4 Structure of the Book
In this section, we will discuss the structure of the book, "Exploring the Fractals of AI: Unleashing Knowledge Management in the Era of Artificial Intelligence." The book aims to provide a comprehensive understanding of the intersection between fractals, artificial intelligence (AI), and knowledge management. By exploring the concepts of fractals and their applications in AI, the book delves into how knowledge management can leverage AI capabilities to enhance various aspects of organizational knowledge.
The book is divided into twelve chapters, each focusing on a specific aspect of the subject matter. Let's take a closer look at the contents of each chapter:
Chapter 1: Introduction
The first chapter serves as an introduction to the book, providing an overview of the key concepts and themes. The chapter establishes the foundation for understanding the subsequent chapters by introducing the concept of fractals, the significance of knowledge management, and the role of fractals and knowledge management in the AI era. Additionally, this chapter presents the structure of the book, giving readers a roadmap of what to expect.
Chapter 2: Foundations of Artificial Intelligence
Chapter 2 serves as a primer on the foundations of artificial intelligence. It covers a broad range of topics such as an overview of AI, machine learning algorithms, deep learning and neural networks, natural language processing, computer vision, and AI ethics and bias. These foundational concepts are crucial for understanding the capabilities and limitations of AI, which are integral to the discussions on fractals and knowledge management.
Chapter 3: Fractals
Chapter 3 is dedicated to exploring the world of fractals. It starts with an introduction to fractal geometry, explaining the underlying principles and properties of fractals. The chapter then focuses on fractal dimension and self-similarity, discussing how these concepts contribute to the unique characteristics of fractals. Furthermore, it explores common fractal patterns and their applications in mathematics and science. Lastly, the chapter delves into the ways fractals are utilized in artificial intelligence.
Chapter 4: Knowledge Management
Chapter 4 delves into the fundamental concepts of knowledge management. It begins by defining knowledge management and its significance in contemporary organizations. The chapter explores various aspects of knowledge management, such as knowledge creation and acquisition, storage and retrieval, sharing and collaboration, as well as knowledge transfer and application. By providing a comprehensive understanding of knowledge management, this chapter sets the stage for the exploration of AI and its relationship with knowledge management.
Chapter 5: AI and Knowledge Management
Chapter 5 focuses on the intersection between AI and knowledge management. It examines how AI technologies can drive knowledge discovery, capture, organization, sharing, collaboration, transfer, and application. Additionally, it highlights the ethical considerations that arise when AI is employed in knowledge management processes. This chapter demonstrates how AI can enhance various aspects of knowledge management, making it more efficient and effective.
Chapter 6: Fractal Patterns in AI Applications
Chapter 6 analyzes the role of fractals in different AI applications. It explores the ways in which fractal patterns are utilized in machine learning, natural language processing, computer vision, and other AI domains. The chapter also examines specific fractal-based AI algorithms and how they contribute to innovation within the field. By showcasing the relationship between fractals and AI applications, this chapter illustrates the potential of fractals to enhance AI capabilities.
Chapter 7: Knowledge Management in AI Systems
Chapter 7 dives into the architecture and design of knowledge management systems in the context of AI. It discusses the integration of cognitive computing and knowledge management, as well as the utilization of knowledge graphs and semantic technologies. Additionally, this chapter explores the emergence of AI-driven knowledge management platforms and their potential to transform organizational knowledge processes.
Chapter 8: Building AI-Powered Knowledge Repositories
Chapter 8 focuses on the practical aspects of building AI-powered knowledge repositories. It covers topics such as data collection and preprocessing, knowledge extraction and representation, and the design of AI-enabled knowledge repositories. Furthermore, the chapter explores the crucial tasks of knowledge validation and maintenance. Real-world examples of AI-powered knowledge repositories are also provided to illustrate the practical application of the concepts discussed.
Chapter 9: Fractal Visualization and Analysis in AI
Chapter 9 delves into the visualization techniques and analysis of fractal data in the realm of AI. It explores the different ways in which fractal data can be visualized and the insights derived from such visualizations. The chapter also discusses how AI algorithms can be employed to analyze and interpret fractal patterns, contributing to fields such as anomaly detection and predictive analytics.
Chapter 10: Challenges and Future Directions
Chapter 10 addresses the challenges and future directions in the field of AI-driven knowledge management. It explores ethical challenges, privacy and security concerns, data bias and fairness, as well as enhancing human-AI collaboration. The chapter concludes by highlighting emerging trends and providing insights into potential future directions in AI-driven knowledge management.
Chapter 11: Case Studies in Fractal-Based Knowledge Management
Chapter 11 presents real-world case studies that exemplify the application of fractal-based knowledge management in various domains. It explores the role of fractals in robotics and AI, medical knowledge management, financial knowledge management, social media analytics, and manufacturing. These case studies provide practical illustrations of how fractal-based knowledge management can be implemented and its potential impact.
Chapter 12: Conclusion
The final chapter concludes the book by summarizing the key takeaways from each chapter. It highlights the implications of fractal-based knowledge management and its future prospects in the field of AI. The chapter also offers final thoughts on the subject matter, encouraging readers to explore further and contribute to the advancement of knowledge management in the era of artificial intelligence.
Through this comprehensive structure, "Exploring the Fractals of AI: Unleashing Knowledge Management in the Era of Artificial Intelligence" provides readers with a deep understanding of the concepts, applications, and implications of fractals, AI, and knowledge management. It equips readers with the knowledge necessary to leverage the power of fractals and AI to enhance knowledge management practices and drive innovation in their organizations.
## 2. Foundations of Artificial Intelligence
### 2.1 Overview of Artificial Intelligence
Artificial Intelligence (AI) has become a transformative force across various industries, revolutionizing the way we live and work. It encompasses a wide range of technologies and techniques that enable machines to perform tasks that traditionally require human intelligence. AI systems can perceive their environment, reason, learn from experience, and make decisions autonomously. In this section, we will provide an overview of AI, its subfields, and its significance in the era of knowledge management.
AI is a multidisciplinary field that draws upon various disciplines such as computer science, mathematics, psychology, and linguistics. It aims to develop intelligent machines that can replicate, simulate, or enhance human cognitive abilities. These machines are designed to understand, learn, and apply knowledge to solve complex problems effectively. AI systems are capable of processing large volumes of data, extracting patterns, and making decisions based on algorithms and models.
The field of AI encompasses several subfields, each focusing on specific aspects of intelligent systems. Machine learning, a prominent subfield of AI, involves the development of algorithms that enable systems to learn from data and improve their performance over time without explicitly being programmed. Machine learning techniques, such as supervised learning, unsupervised learning, and reinforcement learning, underpin many AI applications.
Deep learning and neural networks are subfields of AI that aim to emulate the structure and functioning of the human brain. Deep learning algorithms, inspired by the neural networks of the brain, are capable of learning patterns and features from vast amounts of data, leading to breakthroughs in areas such as image and speech recognition. Neural networks, composed of interconnected artificial neurons, process and transmit information to make complex decisions.
Natural Language Processing (NLP) is an AI subfield that focuses on enabling machines to understand, interpret, and generate human language. NLP techniques allow AI systems to analyze and comprehend textual data, enabling tasks such as sentiment analysis, language translation, and chatbot interactions. This subfield plays a crucial role in knowledge management as it allows organizations to extract meaningful insights from unstructured text documents and facilitate efficient information retrieval.
Computer vision is another subfield of AI that deals with enabling machines to interpret and understand visual information from images or videos. Computer vision algorithms enable AI systems to perceive their environment, recognize objects or patterns, and make decisions accordingly. It has a wide range of applications, including autonomous vehicles, surveillance systems, and medical imaging analysis. By analyzing visual data, organizations can gather valuable knowledge and make informed decisions.
Ethical considerations and bias in AI have gained significant attention in recent years. As AI systems increasingly influence decision-making processes, concerns regarding fairness, transparency, and accountability have emerged. It is crucial to ensure that AI applications do not perpetuate biases or discriminate against certain individuals or groups. Organizations must deploy AI ethically, considering the potential impact on society and addressing inherent biases in data and algorithms.
In the era of knowledge management, AI plays a pivotal role in revolutionizing how organizations acquire, store, retrieve, share, transfer, and apply knowledge. AI-enabled knowledge management systems offer the potential to unlock vast amounts of information and generate actionable insights. By leveraging AI techniques such as machine learning and NLP, organizations can automate knowledge discovery, extract valuable insights from unstructured data, and enable efficient collaboration and knowledge sharing.
AI-driven knowledge management systems can automate the process of acquiring and organizing knowledge, reducing manual effort and improving efficiency. These systems can analyze vast amounts of data to identify relevant information, categorize it, and store it in structured repositories. AI techniques such as knowledge graphs and semantic technologies help organizations uncover hidden relationships and connections among different pieces of knowledge, enabling better decision-making.
Furthermore, AI-powered knowledge management systems can facilitate efficient knowledge sharing and collaboration. By leveraging NLP and machine learning techniques, these systems can provide personalized recommendations, identify subject matter experts, and foster a culture of knowledge sharing within organizations. This enables employees to access the right information at the right time, enhancing productivity and innovation.
AI also plays a crucial role in knowledge transfer and application. By capturing tacit knowledge, which resides in the minds of individuals and is difficult to articulate, AI systems can preserve and transfer it to future generations of employees, ensuring the continuity of critical knowledge within organizations. Additionally, AI techniques such as predictive analytics and anomaly detection can leverage fractal patterns in data to identify trends, predict future outcomes, and detect deviations from normal behavior.
However, integrating AI into knowledge management systems also presents ethical challenges that must be addressed. Organizations need to ensure the responsible and ethical use of AI, safeguarding user privacy, and upholding fairness and transparency. In the era of AI-driven knowledge management, it is essential to establish guidelines, standards, and regulations that govern the collection, storage, and use of data to preserve the integrity and value of knowledge.
In conclusion, AI has transformed the way organizations manage knowledge in the digital era. The subfields of AI, such as machine learning, natural language processing, computer vision, and deep learning, provide the foundation for developing intelligent systems that can process and leverage knowledge effectively. By embracing AI-driven knowledge management, organizations can unlock new opportunities, enhance decision-making processes, and drive innovation in the era of artificial intelligence.
### 2.2 Machine Learning Algorithms
Machine learning is a fundamental component of artificial intelligence (AI) technology, and it plays a significant role in harnessing the power of knowledge management in the AI era. Machine learning algorithms are the backbone of AI systems, enabling them to learn from data, make predictions, and automate intelligent decision-making processes. In this section, we will explore the different types of machine learning algorithms and their applications in knowledge management.
Supervised Learning Algorithms
Supervised learning algorithms are designed to learn patterns and relationships from labeled training data. They are fed with input data and their corresponding output or target values, allowing the algorithm to learn the mapping function between the input and output. The goal of supervised learning is to make accurate predictions or classifications based on new, unseen inputs.
Classification Algorithms
Classification algorithms are one type of supervised learning algorithm that is widely used in knowledge management applications. These algorithms aim to categorize data into predefined classes or categories based on their features. For example, in a knowledge management system, a classification algorithm can be used to automatically categorize documents or articles into relevant topics or subjects. This can aid in organizing and retrieving knowledge efficiently.
Regression Algorithms
Regression algorithms, another type of supervised learning algorithm, are used to predict continuous output variables based on input features. Unlike classification algorithms that deal with categorical variables, regression algorithms focus on numeric or continuous data. In knowledge management, regression algorithms can be utilized to predict factors such as customer satisfaction ratings or sales forecasts based on historical data.
Unsupervised Learning Algorithms
Unsupervised learning algorithms, as the name suggests, do not require labeled data for training. Instead, they aim to discover patterns or structures in the data on their own. Unsupervised learning algorithms are crucial in knowledge management as they enable organizations to uncover hidden insights, identify clusters, and perform data exploration without any prior knowledge or guidance.
Clustering Algorithms
Clustering algorithms are widely used in knowledge management to discover groups or clusters of similar data points based on their features or characteristics. This can be useful for knowledge discovery and organization, allowing organizations to group similar documents, customers, or products together. Clustering algorithms can also help identify anomalies or outliers in data, which may indicate potential opportunities or risks.
Dimensionality Reduction Algorithms
Dimensionality reduction algorithms are employed to reduce the number of features or variables in a dataset while retaining essential information. By eliminating redundant or less informative features, these algorithms enable better data visualization and analysis. In the context of knowledge management, dimensionality reduction algorithms can assist in summarizing and visualizing complex knowledge datasets, facilitating effective decision-making and interpretation.
Reinforcement Learning Algorithms
Reinforcement learning algorithms operate based on a reward system, where an agent learns through trial and error to maximize its rewards in a given environment. These algorithms continuously interact with the environment, taking actions and receiving feedback in the form of rewards or penalties. In the context of knowledge management, reinforcement learning algorithms can be employed to optimize knowledge retrieval, recommend relevant content to users, and personalize knowledge delivery based on user preferences and feedback.
Transfer Learning
Transfer learning is a machine learning technique that leverages pre-trained models or knowledge learned from one task or domain to improve performance in a different but related task or domain. This approach enables the transfer of knowledge between tasks, reducing the need for extensive training on new datasets. Transfer learning can be valuable in knowledge management, as it facilitates the reuse of knowledge models and accelerates the development of AI-powered knowledge systems.
Neural Networks
Neural networks are a class of machine learning algorithms that mimic the functioning of the human brain. These algorithms consist of interconnected layers of artificial neurons, known as artificial neural networks (ANNs). Neural networks are particularly effective in processing complex, high-dimensional data such as images or natural language. In knowledge management, neural networks have been successfully employed in various applications, including text classification, sentiment analysis, image recognition, and recommendation systems.
Machine learning algorithms form the core of AI systems, enabling knowledge management platforms to extract insights, automate knowledge processes, and optimize decision-making. By leveraging these algorithms, organizations can effectively harness the power of AI to unlock the full potential of their knowledge assets.
In the next section, we will delve into deep learning and neural networks, which have revolutionized many domains of AI and knowledge management in particular.>)
### 2.3 Deep Learning and Neural Networks
Deep learning and neural networks are two fundamental components of artificial intelligence (AI) that have revolutionized various industries. In this section, we will explore the concepts and applications of deep learning and neural networks, with a specific focus on their role in knowledge management in the era of AI.
Understanding Deep Learning
Deep learning is a subset of machine learning that utilizes artificial neural networks to model and understand complex patterns in data. Unlike traditional machine learning algorithms, deep learning algorithms are designed to automatically learn hierarchical representations of data by stacking multiple layers of artificial neurons, known as neurons, together. This allows deep learning algorithms to extract higher-level features and representations from raw data, leading to more accurate predictions and intelligent decision-making.
The key advantage of deep learning is its ability to perform end-to-end learning, meaning that the algorithm learns directly from the raw input data without relying on handcrafted feature engineering. Instead, deep learning algorithms automatically learn the most relevant features and patterns directly from the data through a process called backpropagation, which adjusts the weights of the neurons to minimize the difference between the predicted and actual outputs.
Neural Networks and Their Components
Neural networks are the building blocks of deep learning algorithms. They are computational models inspired by the structure and function of biological neural networks in the human brain. A neural network consists of interconnected layers of neurons, where each neuron performs a simple mathematical operation on its inputs and produces an output.
The basic components of a neural network include:
Input Layer: The input layer is responsible for receiving the raw input data and passing it on to subsequent layers for processing.
Hidden Layers: Hidden layers are located between the input and output layers of the neural network. These layers perform the crucial task of learning and extracting meaningful representations from the input data.
Output Layer: The output layer produces the final prediction or output based on the learned representations from the hidden layers.
Each neuron within a neural network is connected to the neurons in the previous and subsequent layers through weighted connections. These connections allow information to flow through the network and facilitate the learning process.
Applications of Deep Learning and Neural Networks in Knowledge Management
Deep learning and neural networks have transformed various aspects of knowledge management in the AI era. Here are some notable applications:
Knowledge Representation and Extraction
Deep learning algorithms can be leveraged to automatically extract knowledge and meaningful patterns from unstructured data, such as text documents, images, and videos. Natural language processing (NLP) techniques, combined with deep learning models like recurrent neural networks (RNNs) and transformers, enable efficient knowledge extraction from textual data. This capability is particularly useful for knowledge management systems that deal with large amounts of textual information, such as digital libraries, document management systems, and chatbots.
Recommendation Systems
Neural networks are extensively employed in recommendation systems, which play a significant role in knowledge management. These systems analyze user preferences and historical behavior to provide personalized recommendations and suggestions. Deep learning models such as deep belief networks (DBNs) and convolutional neural networks (CNNs) have demonstrated exceptional performance in capturing complex patterns and improving the accuracy of recommendations. By leveraging user data, content metadata, and contextual information, recommendation systems enable knowledge managers to deliver relevant and tailored content to users, enhancing the overall knowledge sharing experience.
Knowledge Discovery and Data Mining
Deep learning algorithms, such as deep autoencoders and generative adversarial networks (GANs), are capable of uncovering hidden patterns and insights from large datasets. This ability is particularly useful for knowledge discovery and data mining tasks in knowledge management. By analyzing structured and unstructured data, deep learning techniques can assist in identifying trends, clusters, and anomalies to facilitate decision-making, knowledge acquisition, and innovation.
Content Classification and Tagging
Deep learning models excel at classification tasks, making them invaluable in knowledge management systems that rely on accurate content classification and tagging. Convolutional neural networks (CNNs) are commonly employed for image and video classification, while recurrent neural networks (RNNs) and transformers are used for text classification. By automatically assigning relevant tags and metadata to knowledge assets, deep learning algorithms enhance the discoverability and organization of knowledge, making it more accessible to users.
Knowledge Visualization
Neural networks can also be utilized for knowledge visualization, allowing users to explore and perceive complex knowledge structures effectively. By mapping high-dimensional knowledge data onto lower-dimensional representations, such as graphs or interactive visualizations, deep learning techniques enable users to gain insights and make informed decisions based on the visualized knowledge. This assists in simplifying complex knowledge architectures, improving knowledge understanding, and facilitating knowledge sharing and collaboration.
In summary, deep learning and neural networks play a pivotal role in knowledge management in the AI era. They enable knowledge acquisition, extraction, representation, recommendation, discovery, classification, tagging, and visualization, among other functions. By harnessing the power of deep learning algorithms, organizations can optimize their knowledge management processes, unlock hidden insights, and foster a culture of innovation and collaboration within their knowledge ecosystems.
### 2.4 Natural Language Processing
Natural Language Processing (NLP) is a field of artificial intelligence that focuses on enabling computers to understand, interpret, and generate human language. It plays a crucial role in knowledge management systems, as it empowers machines to process and analyze vast amounts of textual data, extracting valuable insights and facilitating effective knowledge management strategies.
In the era of AI, where data is abundant and constantly expanding, NLP serves as a vital tool for knowledge management by enabling machines to interact with human language in a meaningful way. Through advanced algorithms and techniques, NLP allows computers to comprehend the nuances and complexities of human communication, leading to improved knowledge acquisition, organization, retrieval, and collaboration.
One of the primary applications of NLP in knowledge management is information extraction. With the capability to automatically extract relevant information from unstructured data sources such as articles, documents, and social media, NLP enables organizations to efficiently gather valuable knowledge from various textual sources. By leveraging techniques like named entity recognition, part-of-speech tagging, and sentiment analysis, NLP helps identify key entities, relationships, and sentiments within text, which can be used to populate knowledge management systems with up-to-date and relevant information.
Additionally, NLP plays a crucial role in knowledge organization and retrieval. One effective way of managing knowledge is through the use of chatbots and virtual assistants that employ NLP techniques. By understanding natural language queries, these intelligent systems can provide users with accurate and relevant information from knowledge repositories, fostering efficient knowledge retrieval. NLP-powered search algorithms can understand the context and intent behind user queries, ensuring more precise and targeted results. This capability significantly enhances the user experience and increases the accessibility and usability of knowledge management systems.
Another area where NLP shines is in knowledge collaboration and sharing. Traditional knowledge management systems often rely on structured data and predefined taxonomies, which can hinder flexible collaboration and inhibit knowledge sharing. However, with NLP, organizations can implement intelligent systems that analyze textual content to identify common themes, topics, and expertise among employees. This fosters the creation of communities of practice, where individuals with similar interests or expertise can connect and collaborate effectively. NLP-powered recommendation systems can suggest relevant documents, articles, or experts, promoting knowledge sharing and collaboration between employees.
Furthermore, NLP can enhance knowledge transfer and application within organizations. By automatically extracting knowledge from unstructured textual data, NLP algorithms can convert it into structured and semantically rich representations, such as knowledge graphs. These knowledge representations unlock the potential of machine reasoning and enable intelligent systems to draw insights, make inferences, and provide context-aware recommendations. With NLP, organizations can build intelligent systems that can automatically generate reports, summaries, or recommendations based on a deep understanding of the underlying knowledge.
However, employing NLP in knowledge management systems is not without challenges. One significant hurdle is the inherent ambiguity and complexity of natural language. Humans frequently use sarcasm, idioms, and metaphors, which can be difficult for machines to interpret accurately. Ensuring that NLP algorithms can handle these linguistic nuances and provide accurate results remains an ongoing challenge. Additionally, languages with a lack of resources or complex grammatical structures can pose difficulties in developing accurate NLP models.
Another challenge is the ethical considerations associated with NLP in knowledge management systems. NLP algorithms need to ensure fairness and avoid biases in their analysis and decision-making processes. It is crucial to consider issues of privacy, security, and data protection when implementing NLP techniques in real-world applications. Ensuring transparency and accountability in the use of NLP algorithms is necessary to promote trust and ethical practices.
In conclusion, Natural Language Processing plays a pivotal role in transforming knowledge management in the era of AI. Through its ability to understand, process, and generate human language, NLP enables machines to interact with textual data in a meaningful way, leading to improved knowledge acquisition, organization, retrieval, and collaboration. While challenges persist, the potential of NLP in revolutionizing knowledge management practices is vast, offering organizations efficient and intelligent methods of managing and leveraging their knowledge assets in the AI era.>)
### 2.5 Computer Vision
Computer Vision is a field of artificial intelligence that focuses on enabling computers to understand and interpret visual information from images or videos. It involves developing algorithms and models to extract meaningful insights and make sense of visual data. Computer Vision plays a crucial role in various applications, including object recognition, image classification, image segmentation, and motion detection. In this section, we will explore the intersection of Computer Vision with fractals and knowledge management in the AI era.
Fractals in Computer Vision
Fractals, with their self-similar and recursive patterns, have found significant relevance in the field of Computer Vision. Fractal-based algorithms and techniques have been employed to tackle complex visual recognition and analysis tasks. One area where fractals have been particularly influential is in image compression. Fractal image compression exploits the self-similarity properties of fractals to efficiently store and transmit image data. By using a fractal-based approach, it becomes possible to represent an image using a small amount of data while preserving its visual quality.
Moreover, fractals have also contributed to the development of texture analysis in Computer Vision. Textures are patterns that repeat at small scales within an image. Fractal analysis allows for the characterization and modeling of textures, which is essential for tasks such as texture synthesis, texture segmentation, and texture classification. Fractals provide a powerful framework for describing and understanding the complex patterns and structures present in natural and synthetic textures.
Fractal-Based Feature Extraction
Feature extraction is a fundamental step in Computer Vision, where relevant information is extracted from images or videos to be used for subsequent analysis and decision-making. Fractal geometry offers a unique way of extracting features from visual data. Fractal-based feature extraction techniques focus on capturing the self-similar patterns and structures within an image that may not be easily captured using traditional methods.
By analyzing the fractal dimension and other fractal properties of an image, it becomes possible to extract meaningful features that can be used for various tasks, such as object recognition, image retrieval, and image classification. Fractal-based features provide a rich representation of visual content and can capture intricate details that are not apparent to the human eye or conventional algorithms.
Fractal Analysis for Image Segmentation
Image segmentation is a critical task in Computer Vision that involves partitioning an image into distinct regions to simplify analysis and enable higher-level understanding. Fractal analysis techniques have been employed to aid in the accurate and efficient segmentation of images. By exploiting the self-similarity and geometric properties present in an image, fractal-based segmentation methods can effectively identify boundaries or regions with similar attributes.
Fractal-based segmentation algorithms analyze the variations in fractal dimensions or other fractal measures within an image to determine regions of interest. These algorithms can handle complex images with irregular shapes, fuzzy boundaries, and overlapping objects. Fractal-based image segmentation provides a powerful method to extract meaningful regions and objects from digital imagery, enabling more accurate analysis and understanding.
Knowledge Management in Computer Vision
The increasing complexity of Computer Vision algorithms and models has led to the need for effective knowledge management practices. As Computer Vision systems become more intelligent and have access to vast amounts of visual data, it is crucial to manage and organize this knowledge to ensure efficient and effective decision-making.
Knowledge management in Computer Vision involves capturing, storing, organizing, and retrieving visual knowledge to enhance the performance of computer vision algorithms. It focuses on leveraging the abundance of visual data to improve the accuracy and reliability of vision systems. Effective knowledge management practices enable the transfer and reuse of knowledge across different vision tasks and applications.
AI-Driven Knowledge Management in Computer Vision
Artificial intelligence, particularly machine learning and deep learning techniques, has played a transformative role in knowledge management within Computer Vision. AI-driven knowledge management systems leverage these techniques to automatically extract, organize, and annotate visual knowledge. By training models on large amounts of labeled visual data, AI systems can learn to recognize and understand visual content, enabling efficient knowledge extraction and capture.
AI-powered knowledge management systems can automatically categorize and tag visual data, making it easier to search and retrieve relevant information. These systems can also identify and highlight important patterns and features within images or videos, aiding in better understanding and analysis. Furthermore, AI techniques can enable automated knowledge transfer and integration across different Computer Vision tasks, allowing for the creation of more comprehensive and robust vision systems.
Ethical Considerations in Computer Vision and Knowledge Management
As Computer Vision becomes more pervasive and influential in various domains, ethical considerations surrounding its applications and knowledge management practices are of paramount importance. Issues such as privacy, fairness, and bias need to be carefully addressed to ensure that the development and deployment of Computer Vision systems do not result in negative consequences.
For example, in knowledge management, the data used to train AI models may contain biases or inaccuracies that can perpetuate unfairness or discrimination. It is essential to have robust mechanisms in place to manage and mitigate these biases while ensuring the responsible and ethical use of visual knowledge.
Additionally, privacy concerns arise when dealing with visual data, as it can contain sensitive and personal information. Knowledge management practices must incorporate privacy-preserving techniques to protect individuals' privacy rights and adhere to legal frameworks.
Conclusion
Computer Vision, with its ability to understand and interpret visual data, has greatly benefited from the application of fractals. Fractals have proven to be valuable in various aspects, such as feature extraction, image segmentation, and knowledge management. Their self-similar and recursive patterns provide unique insights and enable more efficient and accurate analysis of visual content.
Moreover, knowledge management in the context of Computer Vision becomes increasingly critical as AI systems become more intelligent and have access to vast amounts of visual data. AI-powered knowledge management systems leverage machine learning and deep learning techniques to facilitate the capture, organization, and retrieval of visual knowledge, contributing to enhanced decision-making and performance.
However, as with any emerging technology, ethical considerations must be at the forefront of computer vision and knowledge management practices. It is necessary to address issues such as privacy, fairness, and bias to ensure the responsible and ethical use of visual knowledge. By incorporating ethical guidelines and frameworks, we can create a future where Computer Vision and fractal-driven knowledge management systems are utilized responsibly and for the benefit of society.
### 2.6 AI Ethics and Bias
In the era of artificial intelligence, it is imperative to consider the ethical implications and potential biases that can arise from the use of AI in knowledge management systems. As AI systems become more prevalent and sophisticated, it is crucial to understand and address the ethical challenges and biases that may arise in order to ensure fairness, transparency, and accountability in AI-driven knowledge management.
Ethical Considerations in AI
Ethics in AI refers to the principles and guidelines that govern the development, deployment, and use of AI systems. While AI has the potential to greatly enhance knowledge management processes, it also brings forth ethical considerations that must be carefully addressed.
Fairness and Transparency
One key ethical concern in AI is ensuring fairness in algorithmic decision-making. AI systems often make decisions based on patterns and correlations in data, which can lead to biased outcomes. Bias can occur when the training data used reflects existing societal biases or when the algorithms themselves inadvertently reinforce or amplify biases. For example, if an AI-driven knowledge management system is trained on biased data, it may perpetuate discriminatory practices or favor certain groups over others when recommending or prioritizing knowledge resources.
To mitigate bias, it is crucial to ensure diverse and representative training data and implement unbiased algorithms. Furthermore, transparency and explainability are essential in AI systems. It is important to understand and communicate how AI-driven knowledge management systems make decisions, enabling users to assess the reliability and fairness of the system's outputs.
Privacy and Security
Privacy and security are significant ethical concerns in AI-driven knowledge management. AI systems often require access to large amounts of data, including sensitive information. It is essential to implement robust privacy measures to protect individuals' personal data from unauthorized access, misuse, or breaches. Organizations must comply with data protection regulations and ensure proper consent, anonymization, and data encryption methods are in place.
AI systems should also be designed with security in mind, employing measures to safeguard against cyber threats and attacks. An AI-driven knowledge management system should prioritize the protection and security of both user data and the knowledge repository itself.
Accountability and Responsibility
When AI systems are involved in knowledge management, accountability and responsibility become critical ethical considerations. It is essential to clearly define roles and responsibilities in the development, deployment, and monitoring of AI systems. Organizations must ensure that individuals are accountable for the decisions and actions of AI-driven systems.
Moreover, in situations where an AI system makes a mistake or produces an undesirable outcome, it is crucial to establish mechanisms for addressing and rectifying such occurrences. Transparency and clear communication channels should be in place to handle user inquiries, complaints, or potential biases detected in the system.
Bias in AI Systems
Bias in AI refers to the unequal treatment or favoritism towards certain groups of people or ideas due to the inherent biases in training data or design choices. Bias can occur in various ways in AI-driven knowledge management systems and can have significant implications for decision-making and knowledge dissemination.
Data Bias
One of the primary sources of bias in AI systems is data bias. AI algorithms learn patterns and correlations from the data they are trained on. If the training data is biased or incomplete, the resulting AI system may perpetuate or amplify those biases. For example, if historical data used for training an AI-driven knowledge management system predominantly includes resources from specific demographics or regions, the system may fail to adequately represent a diverse range of perspectives, resulting in biased recommendations or information retrieval.
To address data bias, organizations need to ensure diverse and representative training datasets that include contributions from a variety of sources and perspectives. Data preprocessing techniques should be implemented to identify and mitigate biases, and ongoing monitoring is necessary to detect and rectify any biases that emerge during the system's operation.
Algorithmic Bias
Another form of bias in AI systems is algorithmic bias, which occurs when the design or implementation of an algorithm leads to unfair or discriminatory outcomes. Bias can stem from various factors, such as the choice of features, the weighting of those features, or the underlying assumptions of the algorithm. Algorithmic bias can unintentionally discriminate against certain individuals or groups by favoring or disadvantaging them in decision-making processes.
To mitigate algorithmic bias, it is essential to carefully design and test algorithms, ensuring that they do not unfairly discriminate against any individuals or groups. Regular audits and reviews of AI systems should be conducted to identify and address any biases that may arise over time.
User Bias
User bias refers to the biases that users may bring to the interaction with AI-driven knowledge management systems. Users' biases and preferences can influence their behavior, decisions, and interactions with the system. User bias can impact the effectiveness and fairness of AI systems, potentially reinforcing existing biases or filtering knowledge based on preconceived notions or prejudices.
To address user bias, it is important to design AI systems that are transparent and adaptable, allowing users to understand and modify the system's behavior based on their preferences. User feedback and diverse user testing must be incorporated to identify and rectify biases that may arise from user interactions.
Mitigating Bias and Ethical Concerns
To mitigate bias and address ethical concerns in AI-driven knowledge management, a multi-faceted approach must be adopted. This approach includes:
Diverse and Representative Data: Ensuring that the training data used for AI systems is diverse, representative, and thoroughly vetted for biases.
Unbiased Algorithm Design: Implementing algorithms that are designed to be unbiased and regularly audited to identify and rectify any emergent biases.
Transparency and Explainability: Providing transparent explanations of how AI systems make decisions, enabling users to understand the basis for the system's outputs and assess their fairness and reliability.
Ongoing Monitoring and Evaluation: Regularly monitoring and evaluating AI systems to detect, assess, and rectify biases or ethical concerns that may emerge over time.
User Input and Participation: Incorporating user feedback and involving users in the design and evaluation processes to ensure that the system reflects their needs and values.
Collaborative Efforts: Engaging in collaborative efforts within the AI community and across industries to share best practices, establish standards, and collectively address ethical challenges and biases.
By adopting these measures, organizations can strive to develop and deploy AI-driven knowledge management systems that are fair, transparent, and accountable, while minimizing biases and maximizing the benefits and potential of AI technology in knowledge management.
## 3. Fractals
### 3.1 Introduction to Fractal Geometry
Fractal geometry is a mathematical concept that has gained significant attention in various fields, including art, science, and technology. It is based on the idea of self-similarity, where a geometric pattern exhibits the same intricate details regardless of the scale at which it is observed. The study of fractals involves understanding the properties, dimensions, and behavior of these complex and recursive patterns.
In the era of Artificial Intelligence (AI), where immense amounts of data are being generated and utilized, the concept of fractals holds great significance. Fractals provide a unique perspective for understanding and analyzing complex systems, allowing us to uncover hidden patterns, structures, and relationships. This section explores the fundamentals of fractal geometry and its relevance to knowledge management in the AI era.
Fractal Dimension and Self-Similarity:
One of the key aspects of fractal geometry is the concept of fractal dimension. Unlike traditional geometric objects, fractals can have non-integer dimensions, indicating the intricate and infinitely detailed nature of their patterns. Fractal dimensions provide a measure of complexity and self-similarity within a fractal structure.
Self-similarity is a fundamental property of fractals, where smaller components within a fractal pattern resemble the overall pattern. This characteristic allows for the recursive nature of fractals, with similar patterns repeating themselves at different scales. Self-similarity provides a powerful tool for understanding complex systems, as it allows us to zoom in and out of a fractal pattern to analyze its properties in different contexts.
Common Fractal Patterns:
Fractals can take various forms, each with its unique properties and characteristics. Some of the commonly known fractal patterns include the Mandelbrot set, Julia sets, Koch curve, Sierpinski triangle, and the Cantor set. These patterns exhibit self-similarity and intricate details at different levels of magnification, making them ideal for studying complex phenomena.
Applications of Fractals in Mathematics and Science:
Fractal geometry has found numerous applications in various branches of mathematics and science. In physics, fractals have been used to describe the behavior of natural phenomena such as coastlines, cloud formations, and patterns of turbulence. Fractal modeling has also been employed in ecological studies to understand the distribution and growth patterns of biological populations.
In mathematics, fractals have been utilized to solve complex equations, analyze chaotic systems, and understand the behavior of dynamical systems. Fractal geometry has also found applications in computer graphics, where it is used to generate visually stunning images, simulate natural phenomena, and create realistic landscapes.
Fractals in Artificial Intelligence:
As AI continues to permeate various aspects of our lives, the concept of fractals offers valuable insights for knowledge management in this era. The vast amount of data generated by AI systems requires effective methods for understanding, organizing, and exploiting knowledge. Fractal geometry provides a powerful framework for dealing with complex and interconnected data.
By applying fractal concepts to AI systems, we can identify patterns, relationships, and structures within massive datasets. Fractal analysis can help in knowledge discovery, enabling AI algorithms to uncover hidden insights and extract relevant information from seemingly chaotic data.
Moreover, self-similarity and recursive properties of fractals can be utilized to develop AI algorithms that adapt to different scales and levels of complexity. These algorithms can effectively handle increasing data volumes and make accurate predictions or classifications based on patterns observed at different resolutions.
In summary, fractal geometry offers a unique and powerful framework for understanding complex systems and analyzing large datasets in the AI era. The self-similarity and recursive nature of fractals allow for the discovery of hidden patterns, structures, and relationships within data. By incorporating fractal concepts into AI systems, we can enhance knowledge management processes, improve decision-making, and drive innovation in various domains. The subsequent sections will explore specific applications of fractals in AI algorithms, knowledge management architectures, and real-world case studies.
### 3.2 Fractal Dimension and Self-Similarity
Fractal geometry is a fascinating field that explores complex, intricate patterns and structures. One of the fundamental concepts in fractal geometry is the concept of fractal dimension and self-similarity. This concept not only has profound implications in the field of mathematics and science but also in the realm of artificial intelligence (AI) and knowledge management.
Fractal Dimension
Traditional geometry deals with objects that have integer dimensions: a line has one dimension, a plane has two dimensions, and a solid has three dimensions. However, fractal geometry introduced the notion of fractional dimensions, allowing for the exploration of intricate, non-integer dimensional structures.
The concept of fractal dimension measures how a fractal object fills up space. Unlike traditional dimensions, which are whole numbers, fractal dimensions can be any real number. A fractal with a dimension between 1 and 2, for example, partially fills up space like a coastline or a mountain range. Fractals with dimensions greater than 2 fill up space completely or even exceed the dimensions of the space they inhabit.
The fractal dimension is a crucial parameter that characterizes the complexity and self-similarity of a fractal. Through the fractal dimension, we can gain insights into the intricate patterns and repeating motifs that exist within a fractal object.
Self-Similarity
Self-similarity is another key characteristic of fractals. It refers to the property of a fractal object where smaller parts or substructures resemble the whole or larger parts of the fractal. In other words, a fractal exhibits self-replication at different scales.
The self-similarity property of a fractal enables infinite detail and intricate patterns to emerge as we zoom in on different areas of the object. Regardless of the magnification level, the underlying structure remains the same, and the same patterns repeat themselves.
Self-similarity allows us to capture a vast amount of information within a relatively compact representation. This property has profound implications in various scientific domains, such as physics, biology, and finance. It also has significant implications in the field of AI and knowledge management.
Fractals in AI and Knowledge Management
The principles of fractal dimension and self-similarity have found applications in AI and knowledge management. By leveraging these principles, we can unlock new possibilities in data analysis, pattern recognition, and knowledge representation.
Data Analysis and Pattern Recognition
Fractal dimension provides a powerful tool for analyzing complex datasets. By calculating the fractal dimension of a dataset, we can gain insights into its underlying structure and complexity. In AI applications, this can be particularly useful in understanding and categorizing different types of data.
For example, in image processing and computer vision, fractal dimension analysis can be employed to quantify the complexity of images or identify specific patterns within them. This approach has been successfully applied in various fields, including medical imaging, satellite imagery analysis, and object detection.
In natural language processing (NLP), self-similarity properties can be utilized to identify syntactic and semantic patterns within textual data. By analyzing the fractal nature of language, AI algorithms can extract meaningful insights, improve sentiment analysis, and enhance language modeling techniques.
Knowledge Representation
Fractals offer a unique way to represent complex knowledge structures in AI systems. The self-similarity property allows for a hierarchical representation of knowledge, where smaller components mimic larger components. This hierarchical structure enables efficient storage and retrieval of knowledge in AI-driven knowledge management systems.
By representing knowledge using fractal-based models, AI systems can capture both the local and global patterns within a knowledge domain. This approach enables a more comprehensive understanding of the relationships and dependencies between different knowledge elements.
Furthermore, fractal-based representation allows for adaptive knowledge retrieval and personalized knowledge recommendations. AI systems can leverage the self-similarity property to identify relevant knowledge pieces for a specific user based on their preferences and past interactions.
Knowledge Discovery and Innovation
The application of fractal concepts in knowledge management can also facilitate knowledge discovery and innovation. The self-similarity property of fractals enables the identification of recurring patterns and relationships within a vast amount of knowledge.
By analyzing the fractal nature of knowledge, AI algorithms can uncover hidden connections and similarities between seemingly unrelated pieces of information. This can lead to the discovery of new insights, the development of innovative solutions, and the generation of novel ideas.
Moreover, fractal-based approaches can foster serendipity, as the self-similar nature of fractals allows unexpected connections to emerge. These unexpected links can spark creative thinking and lead to innovative breakthroughs in knowledge management and AI applications.
Conclusion
The concepts of fractal dimension and self-similarity provide a powerful framework for exploring complex patterns and structures in the field of AI and knowledge management. By leveraging these concepts, we can enhance data analysis, pattern recognition, knowledge representation, and knowledge discovery in AI-driven systems.
Fractals open up new possibilities for understanding and harnessing the immense complexity of data and knowledge. They enable us to capture and represent intricate patterns, maximize information storage and retrieval, and drive innovation in AI applications.
As we delve deeper into the era of AI, incorporating fractal-based approaches in knowledge management will become increasingly essential. Fractals offer a new lens through which we can navigate the vast amounts of data, uncover hidden relationships, and unleash the true potential of AI-powered knowledge management systems.
### 3.3 Common Fractal Patterns
Fractals, as we explored in the previous sections, are complex geometric patterns that exhibit self-similarity at different scales. They are found abundantly in nature, art, and mathematics. These patterns have also found their way into the field of Artificial Intelligence (AI), where they play a crucial role in various applications and algorithms. In this section, we will delve into some common fractal patterns frequently encountered in AI and their significance in the era of Knowledge Management.
3.3.1 Mandelbrot Set
One of the most famous and iconic fractal patterns is the Mandelbrot Set. Discovered by the mathematician Benoit Mandelbrot, the Mandelbrot Set is a set of complex numbers that, when iterated through a simple equation, reveal a breathtakingly intricate and infinitely detailed pattern. Its characteristic feature is the self-similarity exhibited at different levels of magnification. The Mandelbrot Set has captured the imagination of scientists, mathematicians, and artists alike due to its beauty and complexity.
The Mandelbrot Set finds application in AI algorithms, particularly in the field of image compression and rendering. Its self-similarity properties allow for efficient encoding and decoding of images, resulting in reduced file sizes without significant loss of quality. Additionally, algorithms inspired by the principles of the Mandelbrot Set have also been employed in AI-driven image enhancement and restoration techniques, improving the visual quality of degraded images.
3.3.2 Sierpinski Triangle
The Sierpinski Triangle is another widely recognized fractal pattern. It is formed by recursively subdividing an equilateral triangle into smaller triangles and removing the central triangle in each iteration. The resulting pattern exhibits self-similarity, with each smaller triangle being a copy of the entire structure. The Sierpinski Triangle is a fascinating example of a simple geometric shape giving rise to a complex and visually appealing fractal pattern.
In AI, the concept of the Sierpinski Triangle has been applied in areas such as spatial indexing and efficient data retrieval. By utilizing the self-similarity properties of this fractal pattern, AI algorithms can organize and store spatial data in a hierarchical manner, allowing for quick and accurate search and retrieval operations. This approach finds applications in various domains, including geographic information systems, robotics, and computer vision.
3.3.3 Koch Snowflake
The Koch Snowflake is a fractal curve created by iteratively replacing each line segment of a triangle with a specific pattern. At each iteration, the line segment is divided into three equal parts, and an equilateral triangle is constructed on the middle segment. This process is then repeated for each new line segment, resulting in a complex and infinitely detailed snowflake-like pattern.
In the field of AI, the Koch Snowflake pattern has found application in the generation of realistic and diverse synthetic data. By leveraging the self-similarity and intricate details of this fractal pattern, AI algorithms can generate large volumes of data with varying degrees of complexity. This synthetic data can be used to train machine learning models, augment existing datasets, or simulate real-world scenarios where limited data is available. The ability to generate realistic and diverse data is crucial in building robust and generalizable AI systems.
3.3.4 Dragon Curve
The Dragon Curve is an iterated fractal curve that can be constructed by repeatedly folding a strip of paper in half and adding a 90-degree turn after each fold. The resulting curve exhibits intricate patterns and self-similarity. It has been of great interest in AI due to its applications in image compression, path planning, and data analysis.
Within the realm of AI, the Dragon Curve has been utilized in image compression algorithms to reduce the size of images while maintaining their quality. By exploiting the self-similar properties of the curve, AI algorithms can efficiently encode and decode images, allowing for more efficient storage and transmission. The Dragon Curve has also been employed in path planning algorithms, as it provides a compact representation of complex and winding paths, improving navigation and optimization in AI-driven systems.
3.3.5 Cantor Set
The Cantor Set is a fractal entity formed by repeatedly removing the middle third of a line segment. This iterative process generates a set of points that exhibit self-similarity and lack any intervals. The Cantor Set holds unique properties and has been extensively studied in mathematics.
In the AI domain, the Cantor Set finds application in the field of data compression and time series analysis. By using the self-similar properties of this fractal pattern, AI algorithms can effectively represent and compress time-dependent data, such as financial market data, sensor readings, or physiological signals. The Cantor Set's ability to retain essential features while discarding redundant information makes it a valuable tool in managing and analyzing large and complex datasets.
These are just a few of the common fractal patterns encountered in the field of AI. Each of these patterns embodies the essence of fractal geometry, with self-similarity being a central characteristic. The utilization of fractals in AI applications enables efficient data representation, compression, synthesis, and manipulation. By harnessing the power of fractals, AI-driven Knowledge Management systems can benefit from enhanced storage, retrieval, visualization, and analysis of data in the era of Artificial Intelligence.>)
### 3.4 Applications of Fractals in Mathematics and Science
Fractals, with their intricate patterns and self-repeating properties, have found numerous applications in the fields of mathematics and science. These unique geometric objects have proven to be valuable tools for understanding complex phenomena, modeling natural processes, and solving mathematical equations. In this section, we will explore some of the fascinating applications of fractals in these domains.
#### 3.4.1 Fractal Geometry in Mathematical Analysis
Fractal geometry has revolutionized the field of mathematical analysis by providing a new lens through which to explore complex mathematical concepts. One of the most notable contributions of fractals to mathematics is the concept of fractal dimension. Unlike the familiar integer dimensions such as one, two, or three, fractal dimension allows for fractional or non-integer values. Fractal dimension provides a measure of how intricately a fractal object fills space.
Fractal dimension has proven to be a powerful tool in the study of chaotic systems and nonlinear dynamics. Fractal-based techniques have been used to analyze and predict the behavior of complex systems, such as the weather, population growth, and financial markets. By quantifying the complexity of these systems using fractal dimension, mathematicians can gain insights into their underlying dynamics and make more accurate predictions.
#### 3.4.2 Fractals in Physics and Natural Sciences
Fractals have also made significant contributions to various branches of physics and natural sciences. For example, fractal patterns have been observed in natural phenomena such as coastlines, clouds, mountains, and snowflakes. These naturally occurring fractals exhibit self-similarity at different scales, allowing scientists to use fractal geometry to study and describe their intricate structures.
In physics, fractal analysis has been used to study the behavior of complex physical systems. Fractal-based models have been applied in areas such as fluid dynamics, turbulence, and the growth of biological organisms. Fractal dimension is particularly useful in characterizing the roughness of surfaces and the irregularities present in natural structures.
Furthermore, fractals have found applications in the field of medical imaging. Fractal analysis techniques have been employed to examine various biological structures such as the branching patterns of blood vessels and the complexity of neuronal networks. By quantifying fractal dimensions in these structures, researchers can gain insights into their functionality, development, and potential abnormalities.
#### 3.4.3 Fractals in Computer Science and Data Visualization
Fractals play a vital role in computer science and data visualization, enabling the representation and analysis of complex data sets. Fractal-based algorithms and techniques have contributed to advancements in computer graphics, image processing, and pattern recognition.
In computer graphics, fractals have been used to generate realistic natural scenes, terrain landscapes, and virtual textures. The self-repeating patterns of fractals allow for the efficient generation of complex visuals, offering great potential for creating realistic and visually appealing virtual worlds.
Fractals are also valuable in data visualization, where they provide a means to represent vast amounts of complex data in a visually meaningful manner. Through techniques such as fractal compression and fractal dimension analysis, researchers can simplify the representation of data without losing important information. Fractal-based visualization techniques offer effective ways to explore and interpret complex patterns in scientific and multidimensional data sets.
#### 3.4.4 Fractals in Cryptography and Data Security
Fractals have even found applications in the field of cryptography and data security. Fractal-based encryption algorithms provide a higher level of security by exploiting the complex and self-repeating properties of fractal patterns. These algorithms use fractal transformations to encrypt sensitive information, making it more challenging for adversaries to decipher.
Additionally, fractal-based techniques have been employed in the field of steganography, which involves hiding information within digital media. By embedding secret data using fractal patterns, steganographic algorithms can effectively conceal sensitive information within images or audio files, further enhancing data security and privacy.
#### 3.4.5 Fractals and Chaos Theory
Fractals have also played a vital role in the development of chaos theory, a branch of mathematics that studies complex and unpredictable systems. Chaos theory investigates the behavior of dynamical systems that are highly sensitive to initial conditions, leading to seemingly random or chaotic outcomes.
Fractals provide a visual representation of chaotic behavior, revealing the underlying order within apparent randomness. By analyzing the fractal properties of chaotic systems, scientists can gain insight into their characteristics, attractors, and long-term behavior.
In conclusion, fractals have found diverse applications in mathematics and science, ranging from mathematical analysis and physics to computer science and data visualization. These intricate geometric objects provide valuable insights into complex phenomena, offer efficient means of data representation, and contribute to advancements in various fields of study. Fractal-based techniques continue to foster innovation in scientific research, enabling us to unravel the mysteries of the natural world and harness the power of complexity.
### 3.5 Fractals in Artificial Intelligence
Fractals, with their intricate and infinitely complex patterns, have found their way into various scientific fields, including mathematics, physics, and computer science. As we step into the era of Artificial Intelligence (AI), the integration of fractals and AI opens up new possibilities for knowledge management. In this section, we will explore the applications of fractals in the field of AI and how they can contribute to effective knowledge management.
The Fractal Nature of Data
In the realm of AI, data is the lifeblood. It fuels the training of machine learning models and enables the creation of sophisticated algorithms. However, data comes in diverse forms, often containing hidden patterns and complexities. Fractals provide a unique lens through which we can understand and analyze this data.
One fundamental characteristic of fractals is their self-similarity, meaning that they exhibit similar patterns at different levels of magnification. Similarly, data in the AI era often follows a fractal-like structure. For instance, consider a dataset of customer interactions in an e-commerce platform. At a macro level, we can observe patterns in customer behavior, such as seasonal fluctuations in purchasing habits. Zooming in, we might discover patterns at a micro level, such as individual preferences for certain products or browsing patterns. Fractal analysis techniques can help us uncover these patterns and gain deeper insights into the data.
Fractals in Machine Learning
Machine learning algorithms lie at the heart of AI applications. They enable computers to learn from data and make predictions or decisions without explicit programming. Fractals bring valuable contributions to the field of machine learning by providing innovative approaches to pattern recognition, classification, and prediction tasks.
One way fractals are employed in machine learning is through fractal-based feature extraction. Fractal analysis techniques can capture the underlying structural complexity of data, allowing the extraction of meaningful features for classification algorithms. These features can encode intricate patterns that conventional techniques might overlook, leading to more accurate and robust models.
Moreover, fractal-based algorithms can improve the performance of machine learning models by optimizing their structure. By harnessing the self-similarity property of fractals, researchers have developed algorithms that dynamically adapt their architecture based on the complexity and organization of the input data. These self-adaptive algorithms can enhance the efficiency and performance of AI systems, enabling them to handle diverse datasets and adapt to changing environments effectively.
Fractals in Natural Language Processing (NLP)
Natural Language Processing (NLP) is a branch of AI that focuses on enabling computers to understand and process human language. Fractals have also found their way into this field, offering novel techniques and insights for NLP tasks such as text analysis, sentiment analysis, and language generation.
Fractal-based text analysis techniques allow for a deeper understanding of textual data by exploring the structural complexity within texts. By employing fractal dimension analysis, researchers can quantify the complexity of texts and reveal hidden patterns. This enables more accurate classification of sentiments, identification of key topics, and extraction of meaningful information from large text corpora.
Additionally, fractals can be leveraged to generate natural language. By applying fractal-based algorithms, researchers have developed methods to generate coherent and contextually relevant sentences with a human-like fluency. Fractal-based language generation models can capture the self-similarity and organization present in natural language, leading to more realistic and engaging outputs.
Fractals in Computer Vision
Computer Vision is the field of AI that focuses on enabling machines to interpret and understand visual information, such as images and videos. Fractal analysis techniques offer valuable methods for extracting meaningful features and understanding the structural complexity of visual data.
Fractals can enhance object recognition algorithms by capturing the intrinsic self-similarity present in visual patterns. By analyzing and quantifying the fractal dimension, computer vision systems can identify and classify objects with improved accuracy. Fractal-based approaches also enable the identification of complex hierarchical structures in images, allowing for more detailed analysis and understanding of visual data.
Furthermore, fractal-based algorithms can aid in image synthesis and reconstruction tasks. By leveraging the self-similarity property of fractals, researchers have developed techniques to generate realistic and high-resolution images from low-quality or incomplete data. Fractal-based image reconstruction methods have applications in a wide range of fields, including medical imaging, satellite imagery, and digital art.
Fractal-Driven AI Innovation
The integration of fractals and AI not only enhances existing applications but also opens doors to new possibilities and innovations. The self-similarity and complexity inherent in fractals inspire the development of novel algorithms and approaches in AI research.
Researchers are exploring fractal-based optimization algorithms that can address challenging optimization problems, such as training deep neural networks or tuning hyperparameters. These algorithms draw inspiration from the evolutionary mechanisms observed in fractal structures, leading to more efficient and effective optimization techniques.
Furthermore, the concept of fractal dimensionality has motivated the development of innovative deep learning architectures. Fractal-based convolutional neural networks (CNNs) and recurrent neural networks (RNNs) have been proposed to handle the complex and hierarchical patterns present in diverse datasets. These fractal-driven architectures aim to improve the generalization and robustness of AI models, making them more adaptable to real-world scenarios.
In conclusion, fractals play a significant role in the field of AI by providing unique insights, techniques, and algorithms for knowledge management. They enable the understanding of the intricate patterns and complexities within data, improving the performance of machine learning models, natural language processing systems, and computer vision algorithms. Moreover, the integration of fractals in AI drives innovation and opens up new avenues for research and development, making AI systems more powerful and adaptable in the era of Artificial Intelligence.
## 4. Knowledge Management
### 4.1 What is Knowledge Management?
Knowledge management refers to the process of capturing, organizing, storing, retrieving, and sharing knowledge within an organization to enhance decision-making, innovation, and learning. It involves the systematic management of both explicit and tacit knowledge assets, including data, documents, expertise, experiences, and insights, to facilitate knowledge creation, acquisition, transfer, and application.
In the era of artificial intelligence (AI), knowledge management plays a crucial role in maximizing the potential of AI systems. AI technologies have the ability to process massive amounts of data, identify patterns, and generate insights, making them essential tools for knowledge management. By leveraging AI, organizations can enhance their knowledge management practices and effectively harness their knowledge assets to drive innovation and competitive advantage.
Knowledge management in the AI era goes beyond the traditional approaches of managing knowledge. It encompasses the use of advanced AI techniques, such as machine learning, natural language processing, and computer vision, to automate knowledge processes, extract insights from unstructured data, and facilitate collaboration and decision-making.
One of the key aspects of knowledge management in the AI era is the integration of AI algorithms and tools into the knowledge management workflow. This integration enables organizations to leverage AI capabilities to analyze, interpret, and extract knowledge from diverse data sources, such as documents, emails, social media, and sensor data. AI-driven knowledge management systems can automatically categorize, tag, and index knowledge assets, making them easier to discover and retrieve. This not only saves time but also enhances the overall efficiency and effectiveness of knowledge management processes.
Moreover, AI-powered knowledge management systems can provide personalized recommendations and suggestions based on user preferences and historical usage patterns. Through advanced data analytics and machine learning algorithms, these systems can identify relevant knowledge assets for specific tasks, improve search results, and enable users to discover new insights and connections between different pieces of knowledge.
Another significant aspect of knowledge management in the AI era is the emphasis on knowledge creation and acquisition. AI technologies can help in automating the process of knowledge creation by analyzing and synthesizing large volumes of data to generate new knowledge and insights. For example, AI algorithms can mine customer feedback data to identify emerging trends and preferences, which can then be used to develop innovative products or services.
In addition, AI-powered knowledge management systems can enable organizations to acquire knowledge from external sources, such as research papers, industry reports, and online forums. AI algorithms can automatically extract and summarize relevant information from these sources, providing organizations with valuable insights and knowledge that can inform decision-making and strategy development.
Furthermore, AI-enabled knowledge management systems facilitate knowledge storage and retrieval by providing intelligent search capabilities. Unlike traditional knowledge repositories, which rely on manually assigned metadata and keywords, AI can analyze the content of documents and apply natural language processing techniques to understand their context and meaning. This allows users to search for knowledge using natural language queries, making the retrieval process more intuitive and efficient.
Knowledge sharing and collaboration are also essential components of knowledge management in the AI era. AI technologies can enable organizations to foster a culture of collaboration by providing platforms and tools for employees to share their knowledge, insights, and experiences. AI-driven chatbots and virtual assistants can facilitate real-time knowledge sharing and provide instant access to relevant information and expertise.
Moreover, AI-powered knowledge management systems can support collaborative decision-making by providing intelligent recommendations and insights based on the collective knowledge and expertise of employees. By capturing and analyzing the interactions and contributions of users, these systems can identify patterns, relationships, and expertise networks, leading to more informed and collaborative decision-making processes.
Lastly, knowledge transfer and application are critical aspects of knowledge management in the AI era. AI technologies can be used to develop intelligent tools and applications that facilitate the transfer of knowledge from experts to novices. For example, AI-driven virtual mentors can guide employees through complex tasks, providing step-by-step instructions and personalized feedback.
Furthermore, AI-powered knowledge management systems can enable the seamless integration of knowledge into business processes and workflows. By embedding AI algorithms into operational systems, organizations can automate decision-making, enhance productivity, and drive innovation. For example, AI-driven recommendation systems can assist customer service agents in providing personalized and timely solutions to customer queries.
In conclusion, knowledge management in the AI era leverages AI technologies to enhance the various facets of knowledge management, including knowledge creation and acquisition, storage and retrieval, sharing and collaboration, and transfer and application. AI-powered knowledge management systems enable organizations to harness their knowledge assets and unlock valuable insights and opportunities for innovation and growth. By embracing the fractal nature of AI and knowledge management, organizations can navigate the complex and ever-changing landscape of the AI era and unleash the true potential of knowledge for sustainable success.
### 4.2 The Importance of Knowledge Management
Knowledge is a valuable asset for organizations, and managing it effectively is crucial for success in the era of Artificial Intelligence (AI). With the rapid advancements in AI technologies, organizations have access to vast amounts of data and information. However, without an effective knowledge management strategy, this wealth of information may go untapped and fail to realize its true potential.
The Role of Knowledge Management
Knowledge management (KM) involves the systematic capture, organization, and utilization of an organization's knowledge assets. It aims to create value by harnessing the collective knowledge and expertise of individuals within the organization. KM provides a framework for capturing, storing, and disseminating knowledge across different parts of the organization, ensuring that valuable information is accessible to those who need it.
In the context of AI, knowledge management becomes even more critical. AI algorithms rely on vast amounts of data and information to make accurate predictions and decisions. Effective knowledge management allows organizations to leverage this data by providing the infrastructure and processes needed to organize, analyze, and apply it in AI systems.
Enhancing Decision-Making and Problem-Solving
One of the key benefits of knowledge management in the AI era is the enhancement of decision-making and problem-solving processes. By systematically capturing and organizing knowledge, organizations can create repositories of valuable insights and best practices. These repositories serve as a knowledge base that AI systems can access to provide data-driven recommendations and support decision-making.
For example, in a customer service setting, AI-powered chatbots can utilize knowledge management systems to understand and respond to customer queries more effectively. By tapping into a repository of previous customer interactions, these chatbots can provide accurate and relevant responses, improving the overall customer experience.
Similarly, in the healthcare industry, AI systems can leverage knowledge management platforms to access medical literature, research findings, and patient records. This enables healthcare professionals to make more informed decisions regarding diagnoses and treatment options, improving patient outcomes.
Enabling Continuous Learning and Innovation
Knowledge management also plays a crucial role in enabling continuous learning and fostering innovation within organizations. By capturing and sharing knowledge, organizations can create a culture of learning and collaboration, where employees can benefit from each other's experiences and expertise.
In the era of AI, knowledge management can support the development of AI models by providing the necessary data and insights for training and refining algorithms. Through the systematic documentation of experiments and findings, organizations can build on previous work and avoid unnecessary duplication of efforts.
Furthermore, knowledge management allows organizations to identify trends, patterns, and gaps in their knowledge, leading to innovation and the development of new ideas. By analyzing data and information stored in knowledge management systems, organizations can uncover hidden connections and opportunities for improvement, driving innovation and competitive advantage.
Facilitating Knowledge Transfer and Retention
Effective knowledge management is also vital for facilitating knowledge transfer and retention within organizations. As organizations face an increasing rate of employee turnover and an aging workforce, preserving institutional knowledge becomes critical.
By capturing and organizing knowledge in AI-powered systems, organizations can ensure that valuable knowledge is not lost when employees leave the organization. AI can support the transfer of knowledge by providing recommendations and guidance based on previous experiences and best practices.
Additionally, knowledge management can facilitate knowledge sharing and collaboration among distributed teams. AI-powered collaboration platforms can connect employees with relevant expertise and allow for seamless sharing of knowledge and ideas.
Mitigating Risks and Ensuring Compliance
With the rapid advancement of AI technologies, organizations also face various risks and challenges related to data privacy, security, and ethical concerns. Knowledge management can help mitigate these risks by providing a structured approach to data handling and ensuring compliance with regulations.
By implementing robust knowledge management practices, organizations can establish data governance frameworks, ensuring that sensitive information is appropriately handled and protected. Furthermore, knowledge management can support the identification and mitigation of biases and ethical concerns in AI systems, ensuring fair and responsible use of AI technologies.
Conclusion
In the era of Artificial Intelligence, effective knowledge management is crucial for organizations looking to leverage the power of AI to gain a competitive edge. By systematically capturing, organizing, and utilizing knowledge, organizations can enhance decision-making, foster innovation, facilitate knowledge transfer, and mitigate risks. Knowledge management provides the foundation for AI systems to access and utilize relevant information, driving improved performance and delivering value across various industries. In the next section, we will explore the processes of knowledge creation and acquisition in the context of AI-powered systems.
### 4.3 Knowledge Creation and Acquisition
In the era of artificial intelligence, knowledge creation and acquisition are critical components of effective knowledge management systems. The intersection of AI and knowledge management presents new opportunities for organizations to enhance their ability to create, acquire, and leverage knowledge for decision-making and problem-solving. This section will explore the role of AI in knowledge creation and acquisition, highlighting the potential benefits and challenges that arise in this context.
The Role of AI in Knowledge Creation
Knowledge creation is the process of generating new insights, ideas, and information that can be used to solve problems or make informed decisions. AI technologies play a significant role in facilitating knowledge creation by augmenting human capabilities and automating complex tasks.
Data-driven Knowledge Creation
One of the primary ways AI facilitates knowledge creation is through data-driven approaches. AI algorithms can analyze vast amounts of data, identify patterns, and extract insights that humans may not have been able to discover on their own. Machine learning algorithms, for example, can process large datasets and identify correlations, trends, and anomalies that form the basis of new knowledge.
By leveraging AI techniques such as deep learning and natural language processing, organizations can acquire knowledge from a variety of sources, including text documents, social media feeds, sensor data, and more. These technologies enable automated data extraction, interpretation, and analysis, allowing organizations to make sense of large volumes of information and generate valuable insights.
Cognitive Assistance
In addition to data-driven approaches, AI can also provide cognitive assistance to humans during the knowledge creation process. For example, AI-powered chatbots or virtual assistants can interact with users, answer questions, and provide recommendations based on their understanding of the available knowledge. These cognitive assistants can help users explore and uncover relevant information, contributing to the creation of new knowledge.
Furthermore, AI techniques such as natural language generation (NLG) can automatically generate written content, summaries, or reports based on the available knowledge. This capability not only saves time but also enhances the efficiency and effectiveness of knowledge creation by synthesizing complex information into easily understandable formats.
Challenges in Knowledge Creation with AI
While AI offers significant potential in knowledge creation, there are several challenges that organizations must address to fully capitalize on its benefits.
Data Quality and Bias
One key challenge is ensuring the quality and integrity of the data used for knowledge creation. AI systems heavily rely on data inputs, and if the data is incomplete, inaccurate, or biased, it can lead to erroneous insights or skewed knowledge. Organizations must implement robust data quality control measures and conduct regular audits to minimize the risk of biased or unreliable knowledge creation.
Creativity and Innovation
Another challenge lies in the limitations of AI systems when it comes to creative and innovative thinking. While AI can assist in generating knowledge based on existing patterns and data, it may struggle to propose truly novel and groundbreaking ideas. Human creativity and intuition play a vital role in driving innovation, and organizations must strike a balance between AI-driven knowledge creation and human-led ideation to foster breakthroughs.
Knowledge Acquisition with AI
Knowledge acquisition is the process of acquiring external and internal knowledge sources to enrich an organization's knowledge base. AI technologies can greatly benefit this process by automating the acquisition and organization of knowledge from various sources.
Automated Information Retrieval
AI-powered information retrieval systems can automatically gather information from both structured and unstructured sources. For example, web scraping and text mining techniques can extract relevant information from websites, research papers, or social media platforms. These automated processes save time and effort, enabling organizations to acquire knowledge from a wide range of sources efficiently.
Semantic Technologies
Semantic technologies, such as semantic search or ontology-based approaches, enhance knowledge acquisition by understanding the meaning and context of information. By leveraging semantic technologies, AI systems can better categorize, organize, and link knowledge, making it easier to acquire and integrate new information.
Expert Systems
AI can also facilitate knowledge acquisition through expert systems. These systems capture the expertise of domain experts and make it accessible to a broader audience. By combining structured knowledge representations and AI reasoning techniques, expert systems can provide recommendations, answer queries, and support decision-making processes.
Challenges in Knowledge Acquisition with AI
While AI offers significant advantages in knowledge acquisition, some challenges must be addressed to ensure the effectiveness and reliability of the acquired knowledge.
Information Overload
The abundance of information available in the digital age can lead to information overload, where organizations struggle to filter and process the relevant knowledge effectively. AI can help address this challenge by leveraging techniques such as natural language processing and machine learning to filter, organize, and prioritize information based on relevance and importance.
Trust and Validation
Another challenge is ensuring the trustworthiness and accuracy of acquired knowledge. AI systems may encounter misleading or false information, and organizations must implement mechanisms to validate the acquired knowledge before integrating it into their knowledge management systems. Incorporating human judgment and expertise is crucial to validating knowledge and detecting any potential biases or inaccuracies.
In conclusion, AI plays a crucial role in knowledge creation and acquisition in the era of artificial intelligence. By leveraging data-driven approaches and cognitive assistance, AI can enhance the generation of new knowledge and facilitate the acquisition of external and internal knowledge sources. However, organizations must address challenges related to data quality, bias, creativity, information overload, and trust to fully benefit from AI-driven knowledge creation and acquisition.
### 4.4 Knowledge Storage and Retrieval
Knowledge storage and retrieval play a crucial role in the field of knowledge management, especially in the era of artificial intelligence (AI). As AI technologies continue to advance, the need to effectively store and retrieve knowledge becomes paramount for organizations seeking to maximize the potential of their data and information. In this section, we will explore the relationship between fractals and knowledge storage and retrieval, highlighting the ways in which fractal-inspired approaches can enhance these processes in AI-driven knowledge management systems.
Understanding Knowledge Storage
Before delving into the application of fractals in knowledge storage, it is important to grasp the concept itself. Knowledge storage refers to the systematic management and organization of information and knowledge assets within an organization. It involves the collection, storage, and categorization of data, documents, and other sources of knowledge to enable easy access and retrieval when needed.
In the context of AI, knowledge storage has expanded beyond traditional databases and file systems. With the advent of big data and the proliferation of unstructured information, organizations are faced with the challenge of capturing and organizing vast amounts of heterogeneous data, including text, images, audio, and video. This is where fractal-based approaches can offer unique solutions.
Fractals and Knowledge Storage
Fractals, with their self-repeating patterns and infinite complexity, provide a powerful framework for storing and organizing knowledge in AI systems. By leveraging the principles of self-similarity and recursive decomposition, fractal-based knowledge storage techniques can effectively handle large and diverse datasets, ensuring that every piece of information is captured and preserved.
One way in which fractals can be applied to knowledge storage is through the use of recursive indexing. Traditional indexing methods rely on hierarchical or relational structures, which can be limiting when dealing with complex and interconnected knowledge. Fractal-based indexing, on the other hand, allows for a more flexible and scalable approach.
By recursively partitioning knowledge into smaller and more manageable components, fractal-based indexing enables efficient storage and retrieval of information at various levels of granularity. This approach not only enables faster search and retrieval but also facilitates the exploration and discovery of interconnected knowledge, uncovering hidden relationships and patterns that may have otherwise been overlooked.
Fractal-Based Knowledge Retrieval
Once knowledge is stored using fractal-inspired techniques, the process of retrieval becomes significantly enhanced. Fractals enable the creation of knowledge retrieval systems that are more intuitive, adaptive, and capable of capturing the inherent complexity of real-world knowledge.
Traditional keyword-based search methods often fall short when it comes to capturing the nuance and context of knowledge. Fractal-based retrieval, however, can overcome these limitations by considering the spatial relationships and structural characteristics of knowledge. By capturing the fractal dimension and self-similarity of knowledge, retrieval algorithms can prioritize relevant and meaningful information, providing users with a more accurate and comprehensive search experience.
Moreover, fractal-inspired retrieval systems can adapt to user preferences and behavior, learning from past interactions to refine search results over time. This adaptive approach allows for personalized knowledge retrieval, tailoring the information presented to the specific needs and interests of each user.
Enhancing Knowledge Storage and Retrieval with AI
While fractals offer valuable insights into knowledge storage and retrieval, the integration of AI further enhances these processes. AI techniques, such as machine learning and natural language processing, can be employed to automate the organization and classification of knowledge, improving the accuracy and efficiency of storage systems.
Through the application of machine learning algorithms, AI systems can automatically categorize and tag knowledge, assigning metadata that facilitates efficient retrieval. This intelligent tagging enables users to perform more nuanced searches, filtering information based on specific criteria such as topic, date, author, or relevance.
Additionally, AI-powered natural language processing techniques enable knowledge retrieval systems to understand and process complex search queries. This allows users to interact with the system using natural language, making the retrieval process more intuitive and user-friendly.
Conclusion
In the era of artificial intelligence, knowledge storage and retrieval are essential components of effective knowledge management. Fractal-inspired approaches, with their emphasis on self-similarity and recursive decomposition, provide valuable insights into the organization and retrieval of complex knowledge. By leveraging fractal concepts and combining them with AI techniques, organizations can build robust and adaptive knowledge storage and retrieval systems that empower users to access, explore, and apply knowledge with unprecedented efficiency and accuracy.
### 4.5 Knowledge Sharing and Collaboration
In the era of Artificial Intelligence (AI), knowledge sharing and collaboration have become crucial components of effective knowledge management systems. With the exponential growth of data and the complexity of AI algorithms, harnessing the power of collective intelligence is essential for organizations to fully leverage their knowledge assets. Fractals, with their self-similar and scalable nature, can provide valuable insights and innovative approaches to enhance knowledge sharing and collaboration in the AI era.
The Role of Fractals in Knowledge Sharing
Fractals offer a unique perspective on knowledge sharing by highlighting the patterns and structures that exist within information. Just as fractals exhibit self-similarity, where the same patterns are repeated on different scales, knowledge is often interlinked and can be organized in hierarchies, networks, and clusters. By visualizing and understanding these patterns, organizations can better communicate, disseminate, and transfer knowledge among individuals and teams.
Fractal-based approaches can aid in the identification of both explicit and tacit knowledge, allowing organizations to capture and share knowledge more effectively. Explicit knowledge refers to the codified and easily transferable knowledge that can be documented, while tacit knowledge is personal knowledge based on individuals' experiences, skills, and insights. Fractal-based analysis can help uncover hidden patterns and connections within tacit knowledge, enabling organizations to leverage it for decision-making and problem-solving.
Furthermore, fractal-inspired algorithms and techniques can enhance knowledge sharing platforms by providing personalized and context-aware recommendations. By analyzing the patterns of users' interactions and interests, AI systems can suggest relevant content, experts, and communities to foster collaboration and knowledge exchange. These recommendations can help individuals discover new insights, learn from their peers, and contribute their expertise to the organization.
Leveraging Fractals for Collaborative Knowledge Creation
Collaborative knowledge creation is a cornerstone of successful knowledge management systems. Fractals can play a vital role in facilitating collaboration by enabling individuals to contribute their unique perspectives while aligning with the larger organizational goals. Fractal-based visualization tools can help teams visualize complex problems and identify the interdependencies between various components. This visual representation enhances communication and fosters a common understanding, driving innovation and creativity.
Fractals can also support collaborative sensemaking, allowing teams to make sense of complex and ambiguous information collectively. By identifying recurring patterns and underlying structures, teams can solve problems and make decisions more effectively. Fractal analysis can reveal patterns of thinking, reasoning, and problem-solving, enabling teams to develop shared mental models and enhance their collective intelligence.
Additionally, fractal-inspired techniques such as swarm intelligence and collective intelligence can be applied to foster collaboration in AI systems. Swarm intelligence, inspired by the collective behavior of social insects, can be employed to facilitate knowledge sharing and decision-making among AI agents. By leveraging the strengths of multiple AI algorithms and models, swarm intelligence enables AI systems to collaborate in solving complex problems and generating innovative solutions.
Overcoming Challenges in Knowledge Sharing and Collaboration
While the integration of fractals in knowledge sharing and collaboration brings numerous benefits, it also presents challenges and considerations. One of the primary challenges is ensuring the security and privacy of shared knowledge. Organizations must implement robust security measures to protect sensitive information and prevent unauthorized access. Additionally, privacy concerns may arise when collecting and analyzing data for personalized recommendations. Striking a balance between personalized recommendations and users' privacy is crucial to build trust and encourage active participation.
Another challenge is addressing cognitive biases and ensuring diversity in knowledge sharing and collaboration processes. Fractal-based approaches should consider the potential biases in the data and algorithms used, as they can inadvertently reinforce existing biases or neglect valuable perspectives. Organizations must adopt inclusive practices and embrace diversity to promote a wide range of ideas and avoid groupthink. By incorporating fractal-based methods, organizations can analyze patterns and identify biases, enabling a more holistic and diverse knowledge sharing environment.
Future Directions and Opportunities
As technology advances and AI continues to evolve, the integration of fractals in knowledge sharing and collaboration holds tremendous potential. Future research can explore the application of fractal-based algorithms in real-time collaboration, enabling teams to work synchronously despite geographical and time constraints. Additionally, the use of augmented and virtual reality technologies can enhance the visualization and interaction in collaborative knowledge sharing, allowing users to manipulate and explore knowledge in immersive environments.
Another exciting avenue of research is the combination of fractals with blockchain technology to facilitate secure and decentralized knowledge sharing. Blockchain can provide a transparent and tamper-proof platform for recording and verifying knowledge contributions, enhancing trust and incentivizing participation. By leveraging the self-similar nature of fractals, blockchain-based knowledge sharing systems can create a scalable and resilient infrastructure for collaborative knowledge creation.
In conclusion, knowledge sharing and collaboration are vital in the AI era, and fractal-based approaches offer innovative solutions to enhance these processes. By visualizing patterns, enabling personalized recommendations, facilitating collaborative sensemaking, and addressing challenges, fractals can unlock the full potential of knowledge management in the era of Artificial Intelligence. As organizations embrace the power of fractals, they can leverage collective intelligence, drive innovation, and make informed decisions to navigate the complex and rapidly evolving AI landscape.
### 4.6 Knowledge Transfer and Application
Knowledge transfer and application play a crucial role in knowledge management, especially in the era of Artificial Intelligence (AI). In this section, we will explore how fractals and AI can enhance the transfer and application of knowledge, leading to more effective decision-making processes and innovative solutions.
4.6.1 Knowledge Transfer
Knowledge transfer involves the dissemination of knowledge from one entity to another. Traditionally, knowledge transfer has been a manual process, which often leads to inefficiencies and the loss of valuable information. However, with the advancements in AI, the transfer of knowledge has become more streamlined and efficient.
Fractals, known for their self-similar patterns, can be utilized to enhance knowledge transfer processes in AI systems. By leveraging the self-replicating properties of fractals, AI algorithms can identify patterns and extract crucial knowledge from vast amounts of data. These extracted patterns can then be transferred and shared with other AI systems or human users, enabling faster and more accurate decision-making.
Fractal-based algorithms can also assist in the transfer of tacit knowledge, which is often challenging to articulate and share. Tacit knowledge, such as expertise or intuition, can be embedded within the fractal patterns and transferred to other systems or individuals. This fosters the exchange of valuable insights and enhances the overall knowledge transfer process.
Additionally, AI-driven knowledge transfer can benefit from fractal algorithms that enhance the contextuality of information. Fractals can capture the interconnections and relationships within complex datasets, enabling a more comprehensive understanding of the knowledge being transferred. This contextual information facilitates the efficient dissemination of knowledge by providing relevant insights and associations, leading to more informed decision-making.
4.6.2 Knowledge Application
Knowledge application refers to the utilization of knowledge in real-world scenarios to solve problems, drive innovation, and make informed decisions. In the AI era, knowledge application can be greatly enhanced by integrating fractal-based approaches.
Fractals can contribute to knowledge application in AI by enabling accurate prediction and forecasting. Fractal patterns possess inherent predictive capabilities due to their self-similarity and iterative nature. By analyzing and understanding these patterns, AI systems can generate insightful predictions about future trends and events. This aids in proactive decision-making and fosters innovation by identifying emerging patterns and opportunities.
Furthermore, the application of fractals in AI can enhance anomaly detection and pattern recognition. Fractals excel in capturing irregular and complex patterns that may be difficult to identify using traditional methods. AI algorithms leveraging fractal analysis can detect anomalies and patterns in large datasets, enabling prompt identification of outliers or potential issues. This enhances the efficiency and effectiveness of decision-making processes by enabling early intervention and mitigation strategies.
Fractal-based AI algorithms can also contribute to the development of personalized recommendations and insights. By analyzing the individual fractal patterns in user data, AI systems can gain a deep understanding of user preferences and behaviors. This allows for the delivery of highly tailored recommendations and insights, leading to improved user experiences and increased engagement.
Moreover, fractals can play a significant role in knowledge application by facilitating the integration of multidisciplinary knowledge. Fractal analysis enables the identification and understanding of cross-domain patterns and relationships. This enables AI systems to leverage insights from various knowledge domains, leading to more comprehensive solutions and novel approaches to problem-solving.
4.6.3 Challenges in Knowledge Transfer and Application
While the integration of fractals and AI in knowledge transfer and application offers numerous benefits, there are several challenges that need to be addressed.
One significant challenge is the interpretability of fractal-based AI algorithms. Fractal patterns can be highly complex, and understanding the underlying meaning and implications of these patterns can be challenging. Ensuring the transparency and interpretability of AI systems utilizing fractal analysis is crucial to build trust and confidence in the knowledge transfer and application processes.
Another challenge is the availability and accessibility of high-quality data. Fractal-based AI algorithms heavily rely on vast amounts of data to identify meaningful patterns. Ensuring the availability of accurate and diverse datasets is essential to maximize the effectiveness of knowledge transfer and application in AI systems.
The ethical considerations surrounding AI-powered knowledge transfer and application should also be taken into account. The use of sensitive or personal data in AI algorithms raises concerns about privacy, security, and potential biases. It is crucial to establish robust ethical frameworks and regulations to ensure responsible and fair knowledge transfer and application practices.
Conclusion
Knowledge transfer and application are vital components of knowledge management in the era of AI. By leveraging the self-similar and iterative properties of fractals, AI systems can enhance the efficiency and effectiveness of these processes. Fractal-based algorithms contribute to the transfer of knowledge by identifying patterns, capturing tacit knowledge, and providing contextual information. In knowledge application, fractals aid in prediction, anomaly detection, personalized recommendations, and integration of multidisciplinary knowledge. However, challenges such as interpretability, data availability, and ethical considerations need to be addressed to maximize the potential of fractal-based knowledge transfer and application in AI systems.
## 5. AI and Knowledge Management
### 5.1 The Intersection of AI and Knowledge Management
Artificial Intelligence (AI) has revolutionized various sectors and industries, with its capabilities to process and analyze vast amounts of data, identify patterns, and make intelligent decisions. One area where AI has made a significant impact is in knowledge management. As organizations strive to leverage their knowledge assets for competitive advantage, AI offers unique opportunities to enhance knowledge creation, storage, retrieval, sharing, transfer, and application. This section explores the intersection of AI and knowledge management, highlighting the synergies between these two fields and the potential benefits they offer.
Enhancing Knowledge Discovery
One of the key challenges in knowledge management is efficiently discovering relevant and valuable knowledge from vast and diverse data sources. Traditional methods rely on human efforts to search, filter, and extract knowledge, which can be time-consuming and prone to errors. AI-powered techniques, particularly natural language processing (NLP) and machine learning (ML), enable automated knowledge discovery by extracting insights, patterns, and trends from unstructured and structured data sources.
NLP leverages AI algorithms to analyze and understand the meaning and context of human language, enabling the extraction of knowledge from text documents, social media feeds, emails, and other forms of textual data. By employing techniques such as named entity recognition, sentiment analysis, topic modeling, and document clustering, AI can efficiently identify domain-specific knowledge and highlight important relationships between concepts and entities.
ML techniques, such as clustering, classification, and recommendation algorithms, can further enhance knowledge discovery by analyzing patterns and relationships in large datasets. These algorithms can uncover hidden connections, identify similar knowledge items, and provide personalized recommendations, thereby facilitating more effective knowledge acquisition and exploration.
Facilitating Knowledge Capture and Organization
In the era of AI, organizations generate vast amounts of data and knowledge. However, capturing, organizing, and structuring this knowledge remains a challenge. AI technologies can automate and streamline these processes, making knowledge management more efficient and effective.
AI-enabled knowledge capture tools, such as chatbots and virtual assistants, enable real-time interactions with users, allowing them to share their knowledge and experiences. These tools use AI techniques, such as natural language understanding and dialogue management, to capture and store knowledge in a well-structured format.
Knowledge organization, on the other hand, involves categorizing, classifying, and structuring knowledge assets to enable efficient storage and retrieval. AI techniques, such as semantic analysis and knowledge graph construction, can automatically organize knowledge based on concepts, relationships, and hierarchical structures. This not only simplifies the process of searching for relevant information but also enables users to explore related knowledge and uncover hidden insights.
Fostering Knowledge Sharing and Collaboration
The true value of knowledge lies in its dissemination and application across the organization. AI can play a pivotal role in facilitating knowledge sharing and collaboration by providing platforms and tools that encourage active participation and knowledge exchange.
AI-enabled collaboration platforms leverage technologies such as natural language understanding, machine translation, and sentiment analysis to facilitate real-time communication and knowledge sharing among individuals and teams. These platforms can intelligently connect individuals based on their areas of expertise, suggest relevant knowledge resources, and provide personalized recommendations, thereby fostering a culture of collaboration and collective learning.
Additionally, AI-powered knowledge sharing platforms can automatically capture and codify explicit and tacit knowledge, turning unstructured information into valuable knowledge assets. This enables organizations to preserve critical knowledge and make it accessible to employees across different locations and time zones, overcoming the limitations of physical boundaries.
Supporting Knowledge Transfer and Application
Knowledge transfer and application are vital processes for organizations that seek to capitalize on their knowledge assets. AI can support these processes by automating knowledge transfer, augmenting human decision-making, and enabling the application of knowledge in real-time scenarios.
AI-powered chatbots and virtual assistants can act as virtual mentors, providing personalized guidance and support to employees during knowledge transfer processes. These virtual agents can leverage AI techniques such as natural language processing and machine learning to understand the knowledge requirements of individuals and provide them with relevant and context-specific information.
Moreover, AI can enhance decision-making by leveraging knowledge resources and historical data to provide intelligent recommendations and predictions. Through techniques such as pattern recognition, anomaly detection, and predictive analytics, AI algorithms can identify valuable insights and patterns in data, enabling more informed decision-making and problem-solving.
Considering Ethical Considerations
While the integration of AI and knowledge management offers numerous benefits, it also raises important ethical considerations. Organizations must ensure the responsible use of AI technologies while protecting privacy, promoting fairness, and avoiding biases. The deployment of AI systems should adhere to ethical guidelines and regulations to ensure transparency, accountability, and the fair treatment of knowledge and data.
Furthermore, organizations should consider the potential impact of AI on human workers. While AI can enhance knowledge management processes, it should be seen as a tool that complements human intelligence rather than replacing it. Organizations should promote a culture that supports the collaboration between humans and AI systems, fostering a symbiotic relationship that leverages the strengths of both.
Conclusion
The intersection of AI and knowledge management presents a compelling opportunity for organizations to unlock the full potential of their knowledge assets. By leveraging AI technologies such as natural language processing, machine learning, and knowledge graphs, organizations can enhance knowledge discovery, capture, organization, sharing, transfer, and application. However, it is essential for organizations to consider the ethical implications of AI and ensure the responsible and transparent use of these technologies. With the right strategies and considerations in place, organizations can harness the power of AI to drive innovation, collaboration, and success in the era of artificial intelligence.
### 5.2 AI-Driven Knowledge Discovery
In the era of Artificial Intelligence (AI), knowledge management has taken on a whole new dimension. With the advent of AI technologies, organizations are now able to harness the power of intelligent algorithms to discover insights from vast amounts of data and unlock the hidden potential of their knowledge repositories. This section explores how AI can drive knowledge discovery, enhancing the capabilities of traditional knowledge management systems.
AI-driven knowledge discovery involves the use of machine learning algorithms and other AI techniques to uncover patterns, trends, and relationships within datasets. These insights can then be used to generate valuable knowledge that can inform decision-making and drive innovation.
One of the key benefits of AI-driven knowledge discovery is its ability to process and analyze vast amounts of data at a speed and accuracy that is beyond human capability. AI algorithms can sift through massive volumes of structured and unstructured data, such as documents, images, videos, and social media feeds, to identify relevant information and extract meaningful insights.
To facilitate knowledge discovery, AI systems employ various techniques such as natural language processing, machine learning, and deep learning. Natural language processing enables AI algorithms to understand and interpret human language, allowing them to extract knowledge from text-based sources such as documents, articles, and research papers. Machine learning algorithms enable the system to learn from past data and make predictions or recommendations based on patterns and trends. Deep learning, a subset of machine learning, uses neural networks to simulate the working of the human brain and analyze complex, high-dimensional data.
AI-driven knowledge discovery can be particularly useful in domains that generate large volumes of data, such as healthcare, finance, and manufacturing. For example, in healthcare, AI algorithms can analyze medical records, patient histories, and biomedical literature to identify patterns in disease progression, recommend personalized treatment plans, and even predict outbreaks of infectious diseases. In finance, AI can analyze market data, news articles, and social media feeds to identify market trends, detect anomalies, and make informed investment decisions. In manufacturing, AI algorithms can analyze sensor data from production lines to identify patterns that indicate quality issues or predict equipment failures.
Furthermore, AI-driven knowledge discovery can also help organizations overcome the limitations of traditional keyword-based search methods. Traditional search engines rely on exact keyword matches, which often result in either too many irrelevant search results or exclude potentially valuable information that does not precisely match the search terms. AI algorithms, on the other hand, can understand context and meaning, enabling more accurate and relevant search results. By analyzing the content and context of documents, AI algorithms can identify relationships and connections that may not be evident through keyword matching alone.
The integration of AI into knowledge management systems also enables organizations to perform advanced data mining and analysis techniques. For example, clustering algorithms can group similar documents or data points together based on their characteristics, allowing for more efficient organization and navigation of knowledge repositories. Classification algorithms can automatically categorize documents into predefined topics or themes, making it easier to retrieve relevant information. Sentiment analysis algorithms can extract emotions and opinions from text data, providing insights into customer feedback and sentiment trends.
AI-driven knowledge discovery also has the potential to uncover hidden patterns and insights that would otherwise remain undiscovered. By applying advanced analytics techniques, such as association rule mining and anomaly detection, AI algorithms can identify relationships and patterns within datasets that may not be immediately apparent to human analysts. These insights can help organizations make data-driven decisions, identify new opportunities, and improve operational efficiency.
However, it is important to note that AI-driven knowledge discovery is not without challenges. Data quality, data privacy, and ethical considerations are critical factors that need to be addressed. Organizations need to ensure that they have access to reliable and accurate data to avoid biases and erroneous conclusions. They also need to establish robust data governance and security measures to protect sensitive information and comply with regulations.
In conclusion, AI-driven knowledge discovery represents a powerful tool for organizations to unlock the potential of their knowledge repositories and gain valuable insights from vast amounts of data. By leveraging AI technologies, organizations can enhance their knowledge management systems, enabling more efficient and effective knowledge discovery processes. However, it is crucial to address the challenges and considerations associated with AI, to ensure the reliability, privacy, and ethical use of knowledge discovery in the AI era.
### 5.3 AI-Enabled Knowledge Capture and Organization
In the era of Artificial Intelligence (AI), the field of Knowledge Management (KM) has witnessed significant advancements. With the advent of AI technologies, organizations now have the ability to capture, organize, and leverage knowledge in unprecedented ways. AI enables the automated extraction of valuable insights from vast amounts of unstructured data, thereby revolutionizing the traditional approaches to knowledge capture and organization. This section explores the role of AI in knowledge capture and organization, highlighting its benefits, challenges, and potential applications.
Leveraging AI for Knowledge Capture
AI techniques, such as Natural Language Processing (NLP) and Machine Learning (ML), can facilitate the efficient capture of knowledge from various sources. These techniques enable computers to understand and process human language, extracting meaningful information from unstructured text, documents, and even audiovisual content. By utilizing AI algorithms, organizations can automate the extraction of key concepts, entities, and relationships from large volumes of data, fostering the creation of structured knowledge repositories.
One application of AI-enabled knowledge capture is in the domain of content analysis. AI algorithms can analyze vast amounts of text data and extract relevant information, enabling organizations to identify trends, patterns, and insights. For example, in the healthcare sector, AI can process electronic medical records and identify correlations between certain medical conditions, symptoms, and treatments. This information can help healthcare professionals in decision-making processes, improving patient outcomes.
Furthermore, AI can enhance the process of capturing tacit knowledge. Tacit knowledge refers to the expertise and insights possessed by individuals that are difficult to articulate or document. Through machine learning algorithms, AI can analyze patterns of behavior, interactions, and decision-making processes to capture and codify tacit knowledge. This enables organizations to preserve critical knowledge that would otherwise be lost when employees retire or leave the organization.
Organizing Knowledge with AI
Once knowledge has been captured, AI can assist in organizing and structuring it to enhance accessibility and searchability. Traditional knowledge management systems often rely on manual categorization and tagging, which can be time-consuming and prone to errors. AI-powered algorithms, on the other hand, can automatically categorize and tag knowledge based on content analysis, semantic understanding, and contextual relevance.
One approach to organizing knowledge is through the use of knowledge graphs. A knowledge graph represents knowledge as a network of interconnected concepts, entities, and their relationships. AI algorithms can analyze the captured knowledge and automatically create or update the knowledge graph, providing a holistic view of the organization's knowledge landscape. This allows for easier navigation, retrieval, and discovery of relevant knowledge.
Another AI-based technique for organizing knowledge is through clustering and topic modeling. These techniques use unsupervised learning algorithms to identify groups of related documents or topics within a large knowledge repository. By clustering similar documents or identifying key topics, AI can facilitate efficient knowledge retrieval and exploration. This enables users to discover related information, uncover hidden patterns, and gain a deeper understanding of the organization's knowledge assets.
Benefits and Challenges of AI-Enabled Knowledge Capture and Organization
The integration of AI techniques in knowledge capture and organization processes brings numerous benefits to organizations. Firstly, AI can enhance the speed and accuracy of knowledge extraction, enabling organizations to process vast amounts of data in a fraction of the time. This allows for real-time insights and informed decision-making. Secondly, AI provides the ability to capture and codify tacit knowledge, preserving valuable expertise within the organization. This helps to prevent knowledge loss and facilitates knowledge sharing and transfer.
However, there are also challenges associated with AI-enabled knowledge capture and organization. One of the key challenges is ensuring data quality and accuracy. AI algorithms heavily rely on data, and any biases or inaccuracies in the data can affect the quality of knowledge extraction and organization. It is crucial for organizations to have robust data governance frameworks in place to ensure the reliability and integrity of the captured knowledge.
Privacy and security concerns are also noteworthy challenges. AI algorithms may have access to sensitive information, and organizations must ensure compliance with data protection regulations and implement robust security measures to prevent unauthorized access or data breaches. Ethical considerations, such as the fair and transparent use of AI in knowledge management, should also be addressed to maintain trust and mitigate any potential biases or unintended consequences.
Applications of AI-Enabled Knowledge Capture and Organization
The integration of AI with knowledge capture and organization has far-reaching applications across various industries and domains. In the field of customer relationship management, AI-powered knowledge management systems can facilitate personalized interactions with customers, providing them with relevant information and assistance based on their preferences and needs.
In the legal sector, AI-enabled knowledge capture and organization can streamline legal research and case analysis. AI algorithms can process vast amounts of legal documents, precedents, and court rulings, extracting relevant information, identifying legal concepts, and highlighting important insights. This enables legal professionals to access and leverage comprehensive legal knowledge efficiently.
In the field of research and development, AI-powered knowledge management systems can accelerate the discovery of new ideas and technologies. By analyzing scientific literature, patents, and experimental data, AI algorithms can identify connections, trends, and potential areas of innovation. This can help researchers and scientists explore new avenues of inquiry and make breakthroughs in their respective fields.
Conclusion
AI-enabled knowledge capture and organization offer unprecedented opportunities for organizations to leverage their knowledge assets effectively. By automating the capture and organization processes, AI enhances the efficiency, accessibility, and searchability of knowledge repositories. However, organizations must address the challenges associated with data quality, privacy, and ethics to harness the full potential of AI in knowledge management. As AI continues to advance, the integration of AI techniques with knowledge management practices will play a vital role in enabling organizations to unlock the value of their knowledge and remain competitive in the AI era.
### 5.4 AI-Powered Knowledge Sharing and Collaboration
In the era of Artificial Intelligence (AI), knowledge sharing and collaboration have undergone a significant transformation. AI technologies have revolutionized the way organizations manage and disseminate knowledge, enabling more efficient and effective sharing of information across individuals and teams. This section explores the application of AI in knowledge sharing and collaboration, highlighting the benefits and challenges associated with AI-powered knowledge management systems.
The Power of AI in Knowledge Sharing
AI-powered knowledge sharing and collaboration platforms leverage machine learning algorithms and natural language processing techniques to facilitate the exchange of information and expertise. These platforms enable organizations to break down silos, promote cross-functional collaboration, and create a culture of knowledge sharing.
One of the key advantages of AI in knowledge sharing is its ability to automate the process of capturing and organizing knowledge. Natural language processing algorithms can analyze and extract valuable insights from unstructured data sources such as documents, emails, and chat conversations. This enables organizations to transform vast amounts of information into structured knowledge repositories that can be easily searched and accessed by employees.
Additionally, AI algorithms can assist in identifying experts within an organization based on their areas of expertise and past contributions. By leveraging machine learning models, organizations can create algorithms that match individuals with the right knowledge and skills to specific tasks or projects. This not only optimizes resource allocation but also encourages collaboration and knowledge transfer.
AI-Driven Collaboration Tools
AI-powered collaboration tools are another significant development in knowledge management. These tools incorporate AI capabilities such as natural language processing, sentiment analysis, and machine learning to enhance communication and collaboration among team members.
For example, AI-powered chatbots can provide instant assistance by answering questions, providing relevant information, or directing users to appropriate resources. These chatbots can be integrated into collaboration platforms, allowing employees to access knowledge and expertise in real-time.
Furthermore, AI algorithms can analyze communication patterns and provide actionable insights to improve collaboration within teams. By analyzing data such as email conversations, meeting transcripts, and project updates, AI-powered tools can identify potential bottlenecks, communication gaps, or areas where collaboration can be enhanced. This enables organizations to take proactive measures to improve teamwork and knowledge sharing.
Cross-Functional Collaboration and Knowledge Transfer
AI-powered knowledge sharing platforms enable cross-functional collaboration, facilitating the exchange of knowledge and expertise across different departments and disciplines. By breaking down organizational silos, these platforms encourage employees to share insights, ideas, and best practices, ultimately fostering a culture of innovation.
Moreover, AI algorithms can play a significant role in knowledge transfer within organizations. Through machine learning models, tacit knowledge possessed by experienced employees can be captured, documented, and made accessible to others. This ensures that critical knowledge is retained within the organization, even when key personnel leave or retire. AI-powered knowledge transfer systems can identify, capture, and organize valuable insights, allowing organizations to leverage the expertise of their employees effectively.
Challenges in AI-Powered Knowledge Sharing and Collaboration
While AI has revolutionized knowledge sharing and collaboration, it also presents some challenges that need to be addressed. One of the main challenges is ensuring the quality and accuracy of the shared knowledge. AI algorithms can analyze large volumes of data, but they may also capture and propagate incorrect or biased information. It is crucial for organizations to implement robust validation mechanisms to ensure the reliability and relevance of the shared knowledge.
Another challenge is privacy and security. AI-powered knowledge sharing platforms must adhere to strict data protection protocols to safeguard sensitive information. Organizations must implement secure authentication mechanisms, access controls, and encryption techniques to protect the privacy and confidentiality of shared knowledge.
Additionally, there may be resistance to adopting AI-driven knowledge sharing and collaboration tools. Employees may fear that AI technologies could replace their roles or compromise the quality of their work. Organizations need to address these concerns by emphasizing the role of AI as an enabler rather than a replacement. Clear communication and training programs are essential to ensure that employees understand and embrace the benefits of AI-powered knowledge management systems.
Conclusion
AI-powered knowledge sharing and collaboration have the potential to transform the way organizations manage and disseminate knowledge. By leveraging AI technologies, organizations can break down silos, foster cross-functional collaboration, and promote knowledge sharing. AI-driven collaboration tools enhance communication and facilitate real-time access to knowledge and expertise. Furthermore, AI algorithms enable effective knowledge transfer, ensuring that critical expertise is retained within the organization.
However, organizations must address challenges such as validating the quality and accuracy of shared knowledge, ensuring privacy and security, and overcoming resistance to AI adoption. With the right strategies and practices in place, AI-powered knowledge sharing and collaboration can revolutionize knowledge management and drive innovation in the AI era.
### 5.5 AI-Supported Knowledge Transfer and Application
In the era of Artificial Intelligence (AI), the combination of Fractals and Knowledge Management (KM) has the potential to revolutionize the way organizations transfer and apply knowledge. AI offers powerful tools and techniques for extracting, organizing, and analyzing vast amounts of data, while Fractals provide a unique lens through which to understand complex patterns and structures. This section explores the role of AI in supporting knowledge transfer and application, and how Fractals can enhance these processes.
The Power of AI in Knowledge Transfer
AI technologies such as machine learning algorithms, natural language processing, and computer vision, have demonstrated remarkable capabilities in extracting valuable insights from data. This has significant implications for knowledge transfer, as organizations can leverage AI to process and analyze large volumes of information quickly and efficiently.
One of the key applications of AI in knowledge transfer is automated knowledge extraction. Through advanced algorithms, AI can sift through unstructured data sources such as documents, articles, and reports to identify relevant knowledge and extract key insights. This process not only saves time and effort but also ensures that valuable knowledge is not lost in the vast sea of information.
AI can also support knowledge transfer through intelligent recommendation systems. By analyzing users' preferences, behaviors, and historical data, AI algorithms can suggest relevant content, resources, and experts, facilitating the transfer of knowledge in a personalized and targeted manner. These recommendation systems can be particularly useful in large organizations with diverse knowledge repositories, where finding the right knowledge can be a challenge.
Enhancing Knowledge Application with AI
Knowledge application is a critical aspect of KM, as it involves utilizing knowledge to solve problems, make informed decisions, and drive innovation. AI can significantly enhance knowledge application by providing intelligent tools and platforms that enable users to access, process, and apply knowledge effectively.
One of the ways AI supports knowledge application is through cognitive computing. Cognitive computing systems, powered by AI, can understand, reason, and learn from complex datasets, enabling users to interact with the system in a more natural and intuitive manner. This allows users to ask questions, analyze data, and receive contextually relevant insights, facilitating knowledge application in real-time.
AI can also assist in automating repetitive and mundane tasks, freeing up employees' time and energy for more value-added activities. By leveraging AI-powered technologies such as robotic process automation (RPA) and intelligent virtual assistants, organizations can streamline their workflows, automate routine processes, and improve overall productivity. This, in turn, enables employees to focus on higher-order tasks that require critical thinking and creative problem-solving, leading to more effective knowledge application.
Fractal Insights in Knowledge Transfer and Application
Fractals provide a unique perspective on the inherent structure, complexity, and self-similarity of knowledge. By applying fractal concepts in KM processes, organizations can gain deeper insights into the underlying patterns and interconnections within their knowledge repositories.
In the context of knowledge transfer, fractal analysis can help identify common patterns and themes across different domains and disciplines. For example, by analyzing the distribution of key concepts and ideas, organizations can identify recurring themes that cut across multiple fields, enabling cross-pollination of knowledge and fostering interdisciplinary collaboration. Fractal analysis can also help uncover hidden connections and relationships within knowledge, leading to the discovery of novel insights and ideas.
When it comes to knowledge application, fractal insights can enhance problem-solving and decision-making processes. Fractals often exhibit patterns at multiple scales, and this property can be leveraged to address complex problems that involve interactions between different levels of abstraction. By understanding these hierarchical relationships, organizations can develop more effective strategies for applying knowledge in diverse contexts.
Leveraging AI and Fractals for Enhanced Knowledge Transfer and Application
The synergy between AI and Fractals presents exciting opportunities for organizations to enhance knowledge transfer and application processes. By integrating AI-driven technologies with fractal analysis, organizations can unlock new dimensions of knowledge, improve decision-making, and foster innovation.
AI can support the fractal-based analysis of knowledge repositories, enabling organizations to identify self-similar patterns, clusters, and hierarchies within their knowledge assets. This analysis can be used to develop customized knowledge taxonomies, categorization schemes, and recommendation models, enabling more targeted and efficient knowledge transfer.
Additionally, AI-powered visualization techniques can help organizations comprehend complex fractal patterns and structures within their knowledge repositories. By representing knowledge visually, organizations can gain new insights, spot emerging trends, and guide the application of knowledge in a more intuitive and accessible manner.
Furthermore, AI algorithms can identify gaps and areas of misinformation within knowledge repositories, improving the quality and reliability of transferred knowledge. By detecting and correcting inaccuracies and biases, AI can ensure that knowledge is transferred accurately and effectively, reducing the risk of misinformation being propagated.
In summary, AI-supported knowledge transfer and application can leverage the power of AI technologies to extract, organize, and analyze knowledge, while incorporating fractal insights to understand complex patterns and structures. This synergy of AI and Fractals opens up new possibilities for organizations to harness their knowledge assets, solve problems, and drive innovation in the AI era. By embracing these advancements, organizations can elevate their KM practices to new heights and gain a competitive edge in the ever-evolving knowledge-driven landscape.
### 5.6 Ethical Considerations in AI-Driven Knowledge Management
As the integration of Artificial Intelligence (AI) and Knowledge Management (KM) continues to evolve, it is crucial to address the ethical considerations surrounding AI-driven knowledge management. The advancements in AI technology bring with them profound implications and potential ethical challenges. In this section, we will explore some of the key ethical considerations that arise in the context of AI-driven knowledge management.
Transparency and Explainability
One of the primary ethical concerns in AI-driven knowledge management is the lack of transparency and explainability in AI algorithms. AI models, particularly deep learning algorithms, often function as black boxes, making it difficult to understand how decisions are made or knowledge is derived. This lack of transparency raises concerns regarding accountability and the potential for biased or unfair outcomes.
To address this concern, organizations must strive for transparency and explainability in their AI-driven knowledge management systems. It is essential to develop AI algorithms that can provide clear explanations of their decision-making processes and the sources of their knowledge. This transparency will not only enhance trust but also enable individuals and organizations to understand and potentially challenge the outcomes of AI-driven knowledge management systems.
Data Bias and Fairness
Another critical ethical consideration in AI-driven knowledge management is the presence of data bias and the resulting lack of fairness in AI systems. AI algorithms learn from historical data, and if this data contains biases or reflects discrimination, it can perpetuate and even amplify these biases in decision-making processes.
Organizations must be vigilant in detecting and mitigating bias in their AI-driven knowledge management systems. This requires careful attention to the design of training datasets, data collection processes, and algorithmic decision-making. It is crucial to ensure that the data used in AI models is representative and diverse, avoiding the perpetuation of existing biases.
Furthermore, organizations must actively monitor and evaluate the output of their AI-driven knowledge management systems to identify potential biases. Regular audits and assessments can help identify and rectify any biases that may arise, ensuring fair and equitable outcomes.
Privacy and Security Concerns
The integration of AI in knowledge management often involves the processing and analysis of vast amounts of data, including sensitive and personal information. This raises significant privacy and security concerns. Organizations must ensure that they adhere to stringent data protection regulations and implement robust security measures to safeguard the privacy of individuals' information.
Data anonymization techniques, encryption methods, and access controls are just a few of the measures that must be implemented to protect the privacy and security of data. Additionally, organizations should establish clear policies and procedures for data handling and storage, ensuring that data is used only for legitimate purposes and is kept secure from unauthorized access.
Accountability and Responsibility
With the increasing role of AI in knowledge management, the question of accountability and responsibility arises. Who is responsible for the actions and decisions made by AI systems? Should responsibility lie with the developers, the organization deploying the AI, or the AI system itself?
Clear guidelines and frameworks for accountability and responsibility must be established to ensure ethical AI-driven knowledge management. Organizations should adhere to ethical principles, codes of conduct, and legal regulations that govern the use of AI. It is important to define roles and responsibilities, allocating accountability and establishing protocols for monitoring and auditing the performance of AI-driven knowledge management systems.
Human-Centric Focus
While AI has the potential to revolutionize knowledge management, it is essential to maintain a human-centric focus. AI systems should complement human intelligence and contribute to human well-being rather than replace human judgment entirely. Organizations must ensure that AI-driven knowledge management systems are designed and deployed in a manner that empowers individuals and supports human decision-making.
Incorporating human oversight and control in AI systems is vital to address ethical concerns. Humans should be able to intervene, understand, and modify the decisions derived from AI algorithms. Maintaining a balance between human judgment and AI capabilities ensures that ethical considerations are taken into account and prevents undue reliance on AI systems.
Conclusion
Ethical considerations in AI-driven knowledge management are of utmost importance to ensure the responsible and sustainable deployment of AI technology. Transparency, fairness, privacy, accountability, and a human-centric focus are key principles that must guide organizations as they incorporate AI into their knowledge management strategies.
Addressing these ethical considerations will not only foster trust, but also promote the adoption and acceptance of AI-driven knowledge management systems. By prioritizing ethical practices, organizations can leverage AI to enhance knowledge creation, storage, sharing, and transfer while safeguarding the interests and rights of individuals and society as a whole.
## 6. Fractal Patterns in AI Applications
### 6.1 Fractals in Machine Learning
Fractals, with their intricate self-similar patterns, have found their way into various fields of science and technology. Machine learning, a subfield of artificial intelligence, is no exception. In this section, we explore the fascinating relationship between fractals and machine learning and uncover how fractal concepts can enhance the performance and understanding of machine learning models.
Fractal Dimension and Complexity
Fractal dimension is a measure of complexity, providing insights into the self-similarity and intricate structures found in fractal patterns. In machine learning, understanding the complexity of data is crucial for developing accurate models. Fractal dimension can be applied to quantify and analyze the complexity of datasets.
By utilizing fractal dimension, machine learning algorithms can gain a deeper understanding of the structure and relationships within the data. This understanding enables the development of more robust models capable of capturing complex patterns, leading to improved predictions and decision-making.
Self-Similarity and Recursive Algorithms
Fractals exhibit self-similarity, meaning that their patterns repeat at various scales. This property can be harnessed in machine learning through recursive algorithms. Recursive algorithms, also known as fractal algorithms, operate by breaking down a problem into smaller, self-similar sub-problems.
Recursive algorithms are particularly useful in machine learning tasks like clustering, classification, and pattern recognition. They enable the exploration of complex data hierarchies and the discovery of recurring patterns at different levels of granularity. By leveraging the self-similarity inherent in fractal patterns, recursive algorithms can enhance the efficiency and accuracy of machine learning processes.
Fractals and Feature Extraction
Feature extraction is a critical step in machine learning, involving the transformation of raw input data into a format suitable for model training. Fractal analysis techniques can be employed in feature extraction to capture essential characteristics of the data.
Fractal-based feature extraction algorithms can identify and quantify patterns within data, helping to capture both local and global information. This approach enables the representation of complex features that may not be easily discernible using traditional methods. By incorporating fractal features, machine learning models can gain additional insights into the underlying structure of the data, resulting in improved performance and generalization.
Fractal-Based Neural Networks
Neural networks, inspired by the structure of the human brain, have revolutionized machine learning. Fractal concepts can be applied to enhance the architecture and performance of neural networks.
Fractal neural networks are characterized by their hierarchical structure, where each layer represents a different level of abstraction. This hierarchical organization mirrors the self-similarity observed in fractals. By incorporating fractal principles into neural network architectures, models can effectively capture complex relationships and discover intricate patterns within the data.
Furthermore, fractal-based neural networks can improve computational efficiency by reducing the number of parameters required for training. This property is especially significant in deep learning, where overfitting and computational costs pose significant challenges. Fractal-inspired architectures help overcome these obstacles, promoting better generalization and scalability in deep learning applications.
Fractal-Based Optimization Algorithms
Optimization is a fundamental process in machine learning, responsible for fine-tuning model parameters to minimize errors and improve performance. Fractal-based optimization algorithms offer a unique approach to this task.
Traditional gradient-based optimization algorithms often face challenges regarding convergence and exploring the entire solution space. Fractal optimization algorithms, such as fractal gradient descent, introduce self-similarity and recursion to the optimization process. These algorithms navigate the solution space in a fractal pattern, ensuring comprehensive coverage and enhanced convergence properties.
Fractal-inspired optimization algorithms offer improved exploration of complex and multimodal solution landscapes, leading to enhanced performance and improved convergence rates. By leveraging the self-similarity and recursive properties of fractals, these algorithms can find optimal solutions more efficiently.
In conclusion, fractals have emerged as powerful tools for enhancing machine learning algorithms. The self-similar patterns and principles found in fractals can be leveraged to better understand data complexity, develop advanced feature extraction techniques, optimize model performance, and even improve the architecture of neural networks. As the field of machine learning advances, incorporating fractal concepts is likely to play an increasingly important role in unlocking the full potential of artificial intelligence.
### 6.2 Fractals in Natural Language Processing
Natural Language Processing (NLP) is a field of artificial intelligence that focuses on enabling computers to understand, interpret, and generate human language. It encompasses a broad range of tasks, including language translation, sentiment analysis, text summarization, and question-answering systems. When combined with the concept of fractals, NLP has the potential to revolutionize knowledge management in the AI era.
Fractal patterns can be found in various aspects of natural language processing, from text representations to language models. Fractals, with their self-similar and recursive properties, provide a unique perspective for understanding and analyzing the complexity of language. Let's explore some key areas where fractals play a significant role in NLP.
Fractal-Based Text Representations
Text representations are essential for NLP tasks, as they capture the semantics and context of the text. One of the popular text representation techniques is the Word2Vec model, which represents words as dense vectors in a continuous space. However, the fractal word embedding approaches have emerged as powerful alternatives, leveraging the self-similarity property of fractals.
Fractal word embeddings utilize recursive structures such as fractal trees to represent words. This approach captures the hierarchical relationships between words, allowing for more nuanced representations of meaning. Fractal-based representations not only improve the accuracy of NLP models but also enable more efficient knowledge retrieval and semantic analysis.
Fractal Language Models
Language models play a crucial role in tasks such as text generation, speech recognition, and machine translation. Traditional language models, such as n-gram models and recurrent neural networks, have limitations in capturing long-range dependencies and generating coherent text. Fractal-based language models address these issues by incorporating self-similarity and recursive structures.
Fractal language models utilize fractal algorithms, such as Iterated Function Systems (IFS) and L-systems, to generate text. These models generate text by iteratively applying certain transformation rules, resulting in the emergence of complex linguistic patterns. Fractal language models have shown promising results in generating coherent and contextually rich text, allowing for more natural conversation and text synthesis.
Fractal Syntax Parsing
Syntax parsing is a fundamental task in NLP that involves analyzing the grammatical structure of sentences. Traditional parsing techniques, such as Context-Free Grammars (CFG) and Dependency Parsing, often struggle with the inherent ambiguity and complexity of human language. Fractal-based syntax parsing approaches offer an innovative solution by leveraging self-similarity.
Fractal syntax parsing models utilize recursive structures and fractal algorithms to analyze the hierarchical and recursive nature of language syntax. These models capture not only the individual word dependencies but also the overall syntactic structure of the sentence. Fractal syntax parsing enables more accurate and robust parsing, leading to enhanced understanding of natural language and improved performance in downstream tasks.
Fractal-Based Text Classification
Text classification is a common NLP task that involves categorizing text documents into predefined classes. While traditional machine learning algorithms, such as Support Vector Machines and Naive Bayes, have been widely used for text classification, fractal-based approaches offer unique advantages.
Fractal-based text classification models utilize self-similarity and recursive structures to capture the fine-grained patterns and intricate relationships within the text data. By leveraging fractal properties, these models can handle the complexity and variability of natural language more effectively, leading to improved classification accuracy. Fractal-based text classification has been particularly successful in dealing with highly unbalanced and noisy text data.
In conclusion, the integration of fractal geometry with natural language processing holds immense potential for revolutionizing knowledge management in the AI era. Fractal-based text representations, language models, syntax parsing, and text classification techniques offer new insights and improved performance in various NLP tasks. By incorporating self-similarity and recursive structures, these approaches provide a deeper understanding of the complexity of language and enable more accurate analysis and generation of human-like text. With further advancements and research in fractal-based NLP, we can expect to witness significant advancements in knowledge management and AI-driven applications.
### 6.3 Fractals in Computer Vision
Computer vision is a field of artificial intelligence that focuses on enabling computers to interpret and understand visual information from the real world. It involves developing algorithms and techniques that can extract meaningful insights and make sense of images and videos. In recent years, computer vision has significantly progressed, thanks to advancements in deep learning and neural networks. However, an emerging trend within computer vision research is the exploration of fractal patterns and their applications in image analysis and understanding.
Fractals, with their self-similar patterns and infinite complexity, have found intriguing applications in computer vision. They offer unique opportunities for capturing finer details, enhancing image processing algorithms, and enabling more efficient and accurate analysis of visual data. Here, we will explore how the concept of fractals is being leveraged in various aspects of computer vision.
Fractal-Based Image Compression
One of the fundamental challenges in computer vision is handling the massive amount of image data. It is crucial to develop techniques that can efficiently store and transmit these images without losing essential details. Traditional image compression algorithms, such as JPEG, use block-based methods that sacrifice some levels of detail. However, fractal-based image compression provides an alternative approach.
Fractal image compression leverages the self-similarity and repeating patterns found in images to achieve high compression ratios while retaining image quality. This technique breaks an image into smaller blocks and uses fractal codes to encode the relationship between these blocks. By using fractal transformations, the encoder can efficiently recreate the entire image from a smaller set of fractal patterns, reducing the overall size of the data. This approach not only reduces storage requirements but also enables faster transmission of images over networks.
Fractal-Based Object Recognition
Object recognition is a fundamental task in computer vision, where algorithms aim to identify and classify objects within an image or video. Fractal geometry offers a novel approach to object recognition by capturing fine-grained details and complex patterns that are missed by traditional feature-based methods.
Fractal analysis can be used to extract unique fractal signatures from objects in an image. These signatures encode the geometrical properties and statistical characteristics of the object's texture and shape. By comparing these fractal signatures to a database of known objects, computer vision systems can quickly identify and classify objects with high accuracy.
Fractal-based object recognition has proven effective in various applications, including facial recognition, medical image analysis, and surveillance systems. By leveraging the intricate details captured by fractals, these systems can robustly detect and recognize objects under challenging conditions such as low lighting, occlusion, or partial obstruction.
Fractal-Based Image Segmentation
Image segmentation is the process of partitioning an image into meaningful regions based on similarities in color, texture, or other visual properties. Accurate image segmentation is crucial for many computer vision tasks, such as object tracking, image-based modeling, and scene understanding.
Fractal-based image segmentation techniques exploit the self-similarity and repetitive patterns present in images to define boundaries between different objects or regions. Fractal algorithms analyze the variations in pixel intensities and their relationships to identify coherent regions within an image.
These techniques offer advantages over traditional segmentation methods as they can handle complex scenes with irregular object shapes, intricate textures, and varying scales. By leveraging fractal properties, computer vision systems can achieve more accurate and robust segmentation results, even in the presence of noise or overlapping objects.
Fractal-Based Image Enhancement
Image enhancement aims to improve the visibility, clarity, and quality of images by applying various filters or transformation techniques. Fractal-based approaches offer a unique way to enhance images by leveraging the self-similarity and hierarchical characteristics of fractal patterns.
Fractal image enhancement algorithms analyze the fractal dimension and other statistical properties of an image to identify areas with low contrast or noise. By applying specific fractal-based transformations, these algorithms can enhance details in the image while preserving the overall structure and reducing noise artifacts.
This approach is particularly beneficial in challenging scenarios such as low-light conditions or noisy images. Fractal-based image enhancement techniques can bring out hidden details, improve edge sharpness, and enhance overall image quality, making it easier for computer vision algorithms to analyze and interpret the visual information accurately.
Fractal-Based Video Analysis
The applications of fractals extend beyond static images and extend to video analysis as well. Fractal-based techniques are used for video compression, motion estimation, action recognition, and video surveillance.
Due to the repetitive nature of videos, fractal-based video compression algorithms can achieve high compression rates without significant loss of quality. These algorithms exploit the self-similarities across successive frames and the spatial and temporal correlations within videos. By modeling complex motion patterns efficiently, fractal-based methods reduce storage requirements and transmit videos more efficiently.
Fractal-based motion estimation algorithms analyze the fractal properties of video sequences to track and detect motion. These techniques can accurately estimate the motion of objects by capturing the patterns and statistical properties that represent the motion information.
Furthermore, fractal-based methods have been applied to video surveillance systems. By analyzing the fractal properties of video content, these systems can automatically detect abnormal behaviors, monitor crowd movements, and assist in object tracking.
In conclusion, fractals have proven to be a valuable tool in various computer vision applications. From image compression and object recognition to image segmentation and video analysis, fractal-based techniques offer new avenues for enhancing the capabilities of computer vision systems. By leveraging the self-similar patterns and infinite complexity of fractals, researchers and practitioners continue to explore the potential of incorporating fractal geometry into the computational frameworks of computer vision algorithms.
### 6.4 Fractal-Based AI Algorithms
Fractals, with their self-similar patterns and infinite complexity, have found their way into various fields and applications, including artificial intelligence (AI). In this section, we explore the use of fractal-based algorithms in AI and their implications for knowledge management.
Understanding Fractal-Based AI Algorithms
Fractal-based AI algorithms leverage the inherent properties of fractals to enhance the performance and capabilities of AI systems. These algorithms are designed to mimic the self-similarity and recursive patterns found in fractals, allowing AI models to capture and process complex information in a more efficient and effective manner.
One of the key advantages of fractal-based AI algorithms is their ability to handle data with varying degrees of complexity and scale. Fractals, by nature, exhibit self-similarity across different levels of magnification, which means that a small portion of a fractal pattern resembles the overall structure. This property enables AI algorithms to extract meaningful patterns and relationships from large and intricate datasets.
Applications of Fractal-Based AI Algorithms
Fractal-based AI algorithms have been successfully applied in various domains, including image and signal processing, data compression, pattern recognition, and optimization problems. These algorithms have demonstrated their effectiveness in generating accurate predictions, classifying objects, and solving complex problems.
One notable application of fractal-based AI algorithms is in the field of image recognition and computer vision. Traditional convolutional neural networks (CNNs) have limitations in handling large and intricate images due to the fixed receptive field of the filters. Fractal-based algorithms, on the other hand, can recursively segment images into smaller parts, allowing for a more detailed analysis of complex visual information. This capability has led to significant advancements in tasks such as object detection, image classification, and facial recognition.
Another area where fractal-based AI algorithms have shown promise is anomaly detection. Anomalies are often hidden within large volumes of data and can be difficult to identify using traditional statistical techniques. Fractal-based algorithms, with their ability to capture intricate details and patterns, can effectively detect anomalies in datasets by analyzing the self-similarity and deviation from the expected fractal structure. This capability is particularly useful in fraud detection, cybersecurity, and predictive maintenance.
Advantages of Fractal-Based AI Algorithms for Knowledge Management
The application of fractal-based AI algorithms brings several advantages to the field of knowledge management. One significant benefit is the improved ability to process and make sense of large and complex knowledge repositories.
In traditional knowledge management systems, the organization and categorization of information rely on predefined taxonomies and hierarchical structures. However, these approaches often struggle to capture the inherent complexity and interconnectedness of knowledge. Fractal-based AI algorithms, with their ability to capture intricate patterns and relationships, offer a more flexible and dynamic approach to knowledge organization. By analyzing the self-similarities and recursive patterns in knowledge datasets, these algorithms can automatically discover and categorize information in a more contextually relevant and holistic manner.
Furthermore, fractal-based AI algorithms can enhance knowledge discovery by uncovering hidden relationships and insights within vast amounts of data. These algorithms excel in identifying patterns within noisy and unstructured datasets and can unveil previously unknown correlations, trends, and dependencies. By leveraging the self-similarity and recursive properties of fractals, AI algorithms can effectively extract valuable knowledge from diverse data sources, including text documents, scientific articles, social media feeds, and multimedia content.
Lastly, the application of fractal-based AI algorithms in knowledge management can facilitate knowledge transfer and application. By capturing the fractal nature and interconnections of knowledge, these algorithms enable more effective knowledge sharing and collaboration among individuals and teams. They can recommend relevant and contextually appropriate knowledge resources to users based on their preferences and requirements, leveraging the recursive patterns within the knowledge repository.
Conclusion
Fractal-based AI algorithms offer a powerful and innovative approach to knowledge management in the era of artificial intelligence. These algorithms can effectively handle complex and large-scale knowledge repositories, enabling more accurate and contextually relevant knowledge organization, discovery, sharing, and collaboration. By leveraging the inherent self-similarity and recursive patterns of fractals, AI systems can unlock the hidden potential of knowledge and drive innovation across various domains. As AI technology continues to advance, the integration of fractal-based algorithms in knowledge management systems holds great promise for the future of AI-driven decision-making and problem-solving.
### 6.5 Fractal-Driven AI Innovation
Fractals have emerged as a powerful concept in the field of artificial intelligence (AI), revolutionizing various aspects of knowledge management in the AI era. As AI continues to advance, the integration of fractal patterns and principles in AI algorithms and systems offers new and exciting opportunities for innovation and discovery.
Understanding Fractal-Driven AI Innovation
Fractals are intricate geometric patterns that exhibit self-similarity at different scales. They possess a unique property of infinite detail, with each small portion mirroring the overall structure. These complex patterns can be found abundantly in nature, from the branches of a tree to the shape of coastlines, illustrating the inherent elegance and efficiency of natural systems.
In the context of AI, fractals offer a new perspective on developing innovative algorithms and approaches. Fractal-driven AI innovation focuses on harnessing the power of self-similarity and recursive patterns to improve AI systems' performance, efficiency, and adaptability.
Leveraging Fractals in Machine Learning
One area of AI where the application of fractals has shown significant promise is machine learning. Traditional machine learning algorithms often require large amounts of labeled data for training. However, by incorporating fractal principles, researchers have been able to optimize the learning process and enhance the efficiency of algorithm training.
Fractal-based machine learning algorithms exploit the self-similar patterns in datasets, enabling the extraction of meaningful features with fewer training samples. These algorithms effectively capture and represent complex data patterns at different scales, enhancing the accuracy of predictions and reducing the computational complexity of learning tasks.
Moreover, fractal-driven machine learning methods excel at handling high-dimensional data with varying degrees of noise and uncertainty. By leveraging the self-similarity property, these algorithms can discern underlying patterns even in noisy and incomplete datasets, leading to more robust and reliable models.
Fractal-Based Natural Language Processing
Natural language processing (NLP) is another field that benefits from fractal-driven innovation. Understanding and analyzing human language involve dealing with rich and intricate patterns. Fractal principles offer a unique perspective on capturing and modeling the complex structures inherent in language.
Fractal-based NLP algorithms have shown promising results in various tasks, such as sentiment analysis, text summarization, and machine translation. By applying fractal analysis techniques, these algorithms can identify and extract recurring patterns in text, facilitating the interpretation and representation of language data.
Additionally, fractal-driven NLP aids in handling the inherent complexity of language, allowing for more accurate and nuanced understanding. By capturing the self-similarity in linguistic patterns, these algorithms can better model the hierarchical structure of language, capturing semantic relationships and syntactic dependencies more effectively.
Fractals in Computer Vision
Computer vision, an essential part of AI systems, focuses on enabling machines to perceive and understand visual information. Fractals provide a valuable framework for analyzing and interpreting complex visual patterns and structures.
Fractal-based computer vision algorithms excel at tasks such as image recognition, object detection, and image segmentation. By leveraging fractal principles, these algorithms capture the hierarchical nature of visual data, allowing for the efficient representation and analysis of images and videos.
Furthermore, fractal-driven computer vision algorithms offer enhanced resilience to noise and occlusions. The self-similarity property allows these algorithms to accurately recover missing or corrupted visual information, enabling better object recognition and reconstruction.
Fractal-Based AI System Design
In addition to specific AI applications, fractal-driven innovations have also influenced the design and architecture of AI systems. Fractals offer a novel approach to designing AI systems that are adaptive, self-organizing, and robust.
By incorporating fractal concepts into the system architecture, AI systems can exhibit self-similar patterns at different levels, allowing for scalability and flexibility. Fractal-based AI systems can adapt to changing environments and data distributions, making them more resilient and capable of handling diverse tasks.
Moreover, these systems can efficiently process and analyze large volumes of data by employing hierarchical and recursive techniques inspired by fractal principles. The self-similarity property optimizes computational resources, enabling faster and more efficient AI operations.
Future Directions and Challenges
The integration of fractals in AI has opened up exciting prospects for innovation and advancement. However, several challenges need to be addressed as fractal-driven AI progresses.
Firstly, there is a need for further research on the theoretical foundations of fractal-driven AI and the development of new algorithms and techniques. As AI continues to evolve, exploring the full potential of fractal patterns and principles will guide the development of more powerful and efficient AI systems.
Secondly, ethical considerations must be taken into account when utilizing fractal-driven AI. Ensuring fairness, transparency, and accountability in AI algorithms that incorporate fractal principles is crucial to avoid biases and discriminatory outcomes.
Lastly, translating fractal-driven AI innovations into practical applications and real-world environments requires collaboration between AI researchers, domain experts, and industry practitioners. Building interdisciplinary partnerships will lead to the successful implementation of fractal-driven AI in various knowledge management domains.
Conclusion
Fractal-driven AI innovation represents a promising direction for knowledge management in the era of artificial intelligence. Leveraging the inherent self-similarity and recursive patterns of fractals offers new insights and opportunities for developing more efficient and robust AI algorithms and systems. By harnessing the power of fractals, we can unlock the full potential of AI in managing knowledge, enabling us to better understand the world, make informed decisions, and drive innovation forward.
## 7. Knowledge Management in AI Systems
### 7.1 Knowledge Management Architecture in AI Systems
In the era of Artificial Intelligence (AI), knowledge management plays a crucial role in effectively leveraging the power of AI systems. AI systems generate vast amounts of data and insights, and without proper knowledge management architecture, this valuable information may be lost or underutilized.
Knowledge management in AI systems refers to the structured and systematic approach of capturing, organizing, storing, retrieving, sharing, collaborating, transferring, and applying knowledge within AI systems. It provides the foundation for AI-driven knowledge discovery, capture, organization, sharing, collaboration, transfer, and application. The architecture of knowledge management in AI systems encompasses various components and processes that ensure the seamless flow of knowledge across the system.
Knowledge Capture and Acquisition
Knowledge capture and acquisition are the initial steps in the knowledge management architecture of AI systems. This process involves collecting, extracting, and assimilating knowledge from various sources, such as text documents, images, videos, audios, sensor data, social media streams, and other relevant data sources. AI systems employ techniques such as data mining, natural language processing, computer vision, and machine learning algorithms to capture and acquire knowledge from these diverse sources.
The captured knowledge needs to be appropriately represented, structured, and classified for effective organization and retrieval. This stage often involves preprocessing the data, cleaning it, removing noise, and transforming it into a suitable format that can be processed by AI algorithms.
Knowledge Storage and Retrieval
Once the knowledge is captured and acquired, it needs to be stored in a manner that allows easy accessibility and retrieval. Knowledge storage in AI systems may involve the use of specialized databases, knowledge graphs, data lakes, cloud-based storage systems, or other storage mechanisms. These storage systems must provide efficient indexing, searching, and retrieval capabilities to enable quick access to relevant knowledge.
In the context of AI systems, knowledge retrieval is not limited to traditional search mechanisms. It involves leveraging AI techniques to perform advanced searches, semantic matching, similarity analysis, and recommendation systems to retrieve knowledge that is most relevant to the user's needs. This enables AI systems to provide tailored and personalized knowledge recommendations based on user preferences, historical interactions, and contextual information.
Knowledge Sharing and Collaboration
Knowledge sharing and collaboration are pivotal aspects of knowledge management architecture in AI systems. AI systems need to facilitate the exchange of knowledge between individuals, teams, and even across different AI systems. This enables collaborative problem-solving, innovation, and collective intelligence.
Social collaboration platforms, chatbots, virtual assistants, discussion forums, and knowledge sharing portals are some of the tools used in AI systems to promote knowledge sharing and collaboration. These platforms facilitate real-time communication, knowledge sharing, question-answering, brainstorming, and collaboration on problem-solving tasks. AI algorithms can also be used to extract insights from user interactions and conversations, further enriching the knowledge base.
Knowledge Transfer and Application
Knowledge transfer and application are crucial steps in the knowledge management architecture of AI systems. The ultimate goal of AI systems is to utilize the captured knowledge to provide intelligent solutions, make informed decisions, and drive meaningful action. This involves transferring relevant knowledge to the appropriate context and applying it effectively in various domains.
AI systems can transfer knowledge by leveraging machine learning algorithms, deep neural networks, and other AI techniques to analyze patterns, make predictions, and generate insights. Transfer learning, in particular, enables the transfer of knowledge learned from one domain to another, enhancing the efficiency and effectiveness of AI systems.
Once knowledge is transferred, AI systems can apply it in various ways, such as automation, decision support, recommendation systems, anomaly detection, predictive analytics, and optimization. The application of knowledge in AI systems leads to improved performance, increased efficiency, and better outcomes.
Knowledge Management Architecture and AI Integration
The design and implementation of a robust knowledge management architecture in AI systems require a deep integration between AI techniques and knowledge management methodologies. AI algorithms and techniques enable automated knowledge discovery, extraction, representation, retrieval, and application, while knowledge management principles provide the framework for organizing, storing, sharing, and transferring knowledge effectively.
The architecture should also consider the scalability, security, and privacy of the knowledge management system. As AI systems handle vast amounts of sensitive information, proper data governance, access control, and data protection mechanisms must be incorporated into the architecture. Additionally, considering ethical considerations and potential biases in AI algorithms is crucial to ensure unbiased and fair knowledge management practices.
In conclusion, knowledge management architecture in AI systems is vital for unlocking the full potential of AI. It provides the framework for capturing, organizing, storing, retrieving, sharing, collaborating, transferring, and applying knowledge within AI systems. By integrating AI techniques with knowledge management methodologies, organizations can harness the power of AI to enhance decision-making, optimize processes, and drive innovation.
### 7.2 Cognitive Computing and Knowledge Management
Cognitive computing is an interdisciplinary field of computer science that combines aspects of artificial intelligence, machine learning, and natural language processing to create systems that can simulate and augment human cognitive abilities. In the era of artificial intelligence (AI), cognitive computing has emerged as a powerful framework for knowledge management, enabling organizations to harness the power of AI to leverage their vast amounts of data and information effectively.
One of the essential aspects of cognitive computing is its ability to understand, reason, and learn from unstructured data, including text, images, and audiovisual content. By applying advanced algorithms and techniques, cognitive computing systems can analyze and interpret this data, extracting valuable insights and knowledge. This capability is particularly valuable in knowledge management, as it allows organizations to unlock the hidden knowledge contained within their data repositories.
In the context of knowledge management, cognitive computing can play a transformative role in several key areas.
7.2.1 Cognitive Analytics and Knowledge Discovery
Cognitive computing systems excel at analyzing large volumes of diverse and unstructured data to uncover patterns, relationships, and correlations that may not be immediately apparent to humans. By applying machine learning algorithms and natural language processing techniques, these systems can automatically extract, classify, and categorize knowledge from various sources, such as documents, emails, social media, and enterprise systems.
Through cognitive analytics, organizations can gain a deeper understanding of their data, identifying trends, outliers, and emerging patterns. This analysis can help in discovering new knowledge and insights, such as customer preferences, market trends, and operational inefficiencies. By combining cognitive analytics with traditional knowledge management techniques, organizations can improve decision-making, enhance innovation, and drive business growth.
7.2.2 Intelligent Knowledge Capture and Organization
The traditional approach to knowledge capture and organization often involved manual processes, making it time-consuming and prone to human error. Cognitive computing technologies, on the other hand, can automate and streamline these processes, making knowledge management more efficient and effective.
Cognitive systems can automatically extract relevant information from various sources, including structured and unstructured data, and organize it in a structured and searchable format. For example, they can ingest documents, extract key concepts and entities, and create knowledge graphs that represent relationships and hierarchies among different pieces of information. This automated knowledge extraction and organization allow organizations to discover and access relevant knowledge quickly, improving employees' productivity and reducing the effort required to find specific information.
7.2.3 Intelligent Knowledge Sharing and Collaboration
Effective knowledge sharing and collaboration are crucial for organizations to leverage the collective intelligence of their employees. Cognitive computing and AI technologies offer new opportunities to facilitate these processes by capturing, organizing, and delivering knowledge in more intuitive and accessible ways.
For instance, cognitive computing systems can leverage natural language processing and machine learning algorithms to understand user queries and provide relevant and personalized knowledge recommendations. They can also facilitate real-time collaboration by enabling conversational interfaces and virtual assistants that help users find information, answer questions, and collaborate with others. These intelligent knowledge sharing systems enhance the overall efficiency and effectiveness of knowledge exchange within an organization, enabling employees to access the right information at the right time.
7.2.4 AI-Enhanced Knowledge Transfer and Application
Knowledge transfer and application play a vital role in creating value from organizational knowledge. Cognitive computing technologies can augment these processes by providing AI-driven recommendations and insights to employees based on their context and needs.
For example, cognitive computing systems can recommend relevant knowledge resources to employees as they work on specific tasks or projects, enabling them to access the most up-to-date and relevant information. These systems can also leverage machine learning algorithms to analyze historical data and past experiences, identifying patterns and providing recommendations for decision-making and problem-solving.
By embedding cognitive computing capabilities into knowledge management systems, organizations can bridge the gap between explicit and tacit knowledge, facilitate knowledge transfer, and enable employees to make better-informed decisions based on the collective wisdom of the organization.
7.2.5 Ethical Considerations in Cognitive Knowledge Management
While cognitive computing offers immense potential for knowledge management, there are also important ethical considerations that organizations must address. As cognitive systems analyze and interpret data, they can inadvertently introduce biases, reinforce existing prejudices, or misuse sensitive information.
Organizations must establish ethical frameworks and guidelines for the use of cognitive technologies in knowledge management. This includes ensuring transparency and accountability in AI algorithms, safeguarding privacy and data protection, and addressing issues of fairness and bias in knowledge discovery and sharing. By incorporating ethical considerations into cognitive knowledge management practices, organizations can build trust, foster responsible AI usage, and ensure that the benefits of cognitive computing are realized while minimizing potential risks.
In conclusion, cognitive computing, with its ability to understand and learn from unstructured data, is revolutionizing knowledge management in the AI era. By utilizing advanced AI techniques, organizations can discover hidden knowledge, capture and organize information more efficiently, facilitate knowledge sharing and collaboration, enhance knowledge transfer and application, and address ethical concerns. The integration of cognitive computing and knowledge management paves the way for organizations to unleash the full potential of their data and transform it into valuable insights and actionable knowledge.
### 7.3 Knowledge Graphs and AI
In the era of Artificial Intelligence (AI), one of the most powerful tools in knowledge management is the use of knowledge graphs. A knowledge graph is a method of organizing knowledge in a structured format that allows for efficient data retrieval and analysis. By representing relationships between various entities, knowledge graphs enable AI systems to understand and reason about complex information.
What is a Knowledge Graph?
A knowledge graph is essentially a graph-based database that represents knowledge in a structured format. It consists of nodes and edges, where nodes represent entities or concepts, and edges represent the relationships between them. These relationships can be hierarchical, associative, or contextual, allowing for a rich representation of knowledge.
The nodes in a knowledge graph can be anything from a person, organization, location, concept, or even a specific piece of information. For example, in a knowledge graph about a medical domain, the nodes could represent diseases, symptoms, treatments, and relationships between them. The edges would connect these nodes, indicating relationships such as "causes," "treats," or "associated with."
Knowledge Graph Construction
The process of constructing a knowledge graph involves gathering and integrating information from various sources, such as curated databases, structured data, unstructured text, and even user-generated content. This information is then transformed into a graph format using standardized ontologies and schemas.
To create a knowledge graph, AI systems utilize various techniques, including natural language processing (NLP), machine learning, and semantic technologies. NLP algorithms help extract and understand information from unstructured text data, enabling the identification of entities, relationships, and key concepts. Machine learning techniques can be employed to automatically categorize and classify information, as well as resolve ambiguities in the data. Semantic technologies, such as RDF (Resource Description Framework) and OWL (Web Ontology Language), provide the foundation for representing and organizing knowledge in a standardized and interoperable manner.
Benefits of Knowledge Graphs in AI
Knowledge graphs offer several benefits for AI applications in knowledge management:
Enhanced Search and Information Retrieval: By structuring knowledge in a graph format, AI systems can perform more accurate and context-aware searches. Knowledge graphs enable efficient retrieval of relevant information, even when the search query is ambiguous or incomplete. The interconnectedness of nodes in a knowledge graph allows for the exploration of related concepts, expanding the scope of search results.
Interactive Exploration and Discovery: Knowledge graphs provide a visual and interactive experience for users to explore and discover information. Users can navigate the graph, traverse relationships between entities, and uncover new knowledge connections. This interactive exploration facilitates the discovery of insights, patterns, and trends that may not be apparent through traditional search methods.
Contextual Reasoning and Inference: With the rich representation of relationships between entities, knowledge graphs enable AI systems to perform complex reasoning and inference tasks. By leveraging the contextual information encoded in the graph, AI algorithms can identify implicit relationships, make logical deductions, and answer complex queries. This capability is particularly valuable in domains where domain-specific knowledge and expertise are critical.
Integrated Knowledge from Heterogeneous Sources: Knowledge graphs provide a unified framework for integrating knowledge from diverse and heterogeneous sources. By representing information using a common graph format, data from structured databases, unstructured text, and even external linked data sources can be seamlessly connected and queried. This integration enables a holistic view of knowledge and enhances cross-domain analysis and insights.
Knowledge-enabled AI Applications: Knowledge graphs serve as a backbone for developing AI applications that require deep domain understanding. By capturing and organizing domain-specific knowledge, AI programs can leverage this structured knowledge to enhance their performance in tasks such as language understanding, question answering, recommendation systems, and decision-making.
Applications of Knowledge Graphs in AI
Knowledge graphs have been applied successfully in various AI-driven knowledge management applications:
Question Answering Systems: Knowledge graphs can power question answering systems by capturing and organizing factual knowledge. Users can ask complex questions, and the system can leverage the relationships encoded in the graph to provide accurate and context-aware answers.
Semantic Search: By utilizing the structured knowledge in a graph, semantic search engines can deliver more relevant and precise search results. The relationships between entities can guide the search process, ensuring that the retrieved information matches the user's intent.
Recommendation Systems: Knowledge graphs enable personalized recommendations by capturing the preferences and relationships between individuals, products, or content. By analyzing the connections and patterns in the graph, recommendation systems can suggest relevant items based on an individual's interests and preferences.
Expert Systems: Knowledge graphs can be used to build expert systems that emulate human expertise in specific domains. By capturing and codifying expert knowledge in a graph format, these systems can provide intelligent advice, diagnostics, and decision support.
Data Integration and Analysis: Knowledge graphs facilitate data integration and analysis by providing a unified framework for linking and querying disparate data sources. This capability enables organizations to gain insights and extract value from complex and heterogeneous datasets.
Challenges and Future Directions
While knowledge graphs offer significant benefits in AI-powered knowledge management, several challenges need to be addressed to fully unleash their potential:
Data Quality and Integrity: The quality and integrity of the data used to construct knowledge graphs are essential for accurate and reliable knowledge representation. Ensuring data quality, resolving inconsistencies, and addressing data biases are ongoing challenges that need to be tackled.
Scalability and Performance: As knowledge graphs grow in size and complexity, scalability and performance become crucial considerations. Efficient techniques for managing and querying large-scale knowledge graphs need to be developed to enable real-time and interactive analysis.
Ontology Design and Evolution: Designing and evolving ontologies that capture accurate and up-to-date domain knowledge require careful consideration and collaboration between domain experts, knowledge engineers, and AI practitioners. The process of ontology engineering needs to be streamlined to support agile and iterative development.
Ethical and Privacy Concerns: As AI systems leverage knowledge graphs for decision-making, ethical considerations such as fairness, transparency, and privacy become paramount. Ensuring that knowledge graphs adhere to ethical guidelines and protect user privacy is essential for responsible AI deployment.
In the future, knowledge graphs are expected to play a vital role in the evolution of AI and knowledge management. As AI technologies become more sophisticated and capable of reasoning over complex and interconnected knowledge, knowledge graphs will continue to provide the foundation for intelligent decision-making, knowledge discovery, and collaboration in the AI era.
### 7.4 Semantic Technologies and Knowledge Management
In the era of Artificial Intelligence (AI), the vast amount of data generated and consumed by AI systems requires efficient methods for organizing, managing, and extracting knowledge. Semantic technologies play a crucial role in enhancing knowledge management by providing a semantic layer that adds meaning and context to the data, making it easier to understand and utilize.
Semantic technologies are a collection of standards, tools, and techniques that enable machines to understand, interpret, and process information in a meaningful way. They are designed to bridge the gap between human knowledge and digital data, facilitating knowledge discovery, integration, and sharing.
Understanding the Semantic Web
The Semantic Web is a key component of semantic technologies that aims to enable data integration and knowledge sharing across different domains on the World Wide Web. It provides a framework for representing and linking data in a structured and machine-readable format, making it easier for AI systems to interpret and analyze information.
At the core of the Semantic Web are ontologies, which define the relationships and properties of concepts within a specific domain. Ontologies provide a common vocabulary that allows machines to understand and reason about the information. They enable AI systems to go beyond simple keyword matching and retrieve relevant knowledge based on semantic associations.
Semantic Technologies for Knowledge Management
Semantic technologies offer several benefits for knowledge management in AI systems:
Knowledge Representation: Semantic technologies provide a powerful way to represent knowledge in a machine-readable format. By utilizing ontologies and semantic graphs, information can be structured and organized hierarchically, capturing the relationships and dependencies between different knowledge components. This enables efficient knowledge storage and retrieval, ensuring that relevant information can be easily accessed and utilized by AI systems.
Knowledge Discovery: AI systems heavily rely on knowledge discovery to extract useful insights and patterns from the data. Semantic technologies enable the integration of diverse data sources and the establishment of meaningful connections between them. This facilitates more accurate and comprehensive knowledge discovery, allowing AI systems to uncover hidden relationships and discover new knowledge that can enhance decision-making and problem-solving.
Data Integration and Interoperability: AI systems often operate on vast amounts of heterogeneous data from multiple sources. Semantic technologies enable data integration and interoperability by providing a standardized framework for representing and linking data. This ensures that data from different sources can be seamlessly combined and analyzed by AI systems, significantly improving their ability to understand and leverage diverse knowledge.
Semantic Search and Querying: Conventional keyword-based search methods may not always yield the most relevant results. Semantic technologies enable semantic search, which goes beyond simple keyword matching and takes into account the meaning and context of the query. This enhances the accuracy and precision of search results, enabling AI systems to retrieve more relevant knowledge and insights.
Knowledge Sharing and Collaboration: Semantic technologies facilitate knowledge sharing and collaboration by providing a common understanding of information across different stakeholders. By utilizing standardized ontologies and vocabularies, AI systems can communicate and exchange knowledge more effectively. This enables seamless collaboration between humans and AI systems, allowing for the seamless integration of human intelligence and machine intelligence in knowledge management processes.
Use Cases of Semantic Technologies in Knowledge Management
Semantic technologies are being applied in various domains to enhance knowledge management in AI systems. Some prominent use cases include:
Healthcare: Semantic technologies are used to integrate and analyze patient records, medical literature, and clinical guidelines. This enables AI systems to provide personalized diagnoses, treatment recommendations, and assist in medical research.
Finance: Semantic technologies are employed to analyze financial data, identify trends and patterns, and provide personalized financial advice. They assist in risk assessment, fraud detection, and portfolio optimization.
Customer Service: Semantic technologies enable intelligent chatbots and virtual assistants to understand customer queries and provide accurate and relevant responses. They enhance the efficiency and effectiveness of customer service by automating repetitive tasks and quickly retrieving knowledge.
E-commerce: Semantic technologies are utilized in product recommendation systems to provide personalized recommendations based on user preferences and purchasing behavior. They improve the overall shopping experience and enhance customer satisfaction.
Enterprise Knowledge Management: Semantic technologies facilitate the integration and retrieval of knowledge from diverse enterprise data sources such as documents, emails, and databases. They enhance knowledge sharing and collaboration across different departments and enable efficient decision-making.
Challenges and Future Directions
While semantic technologies offer significant benefits for knowledge management in AI systems, there are also challenges that need to be addressed:
Scalability: As the volume of data continues to grow exponentially, ensuring the scalability and performance of semantic technologies is crucial. Efforts are needed to optimize the storage, retrieval, and processing of large-scale semantic data to meet the demands of AI systems.
Data Quality and Accuracy: Semantic technologies heavily rely on high-quality data and accurate ontologies. Ensuring the integrity, completeness, and correctness of data is essential for reliable knowledge management. Techniques for data validation and ontology quality assurance need to be developed and standardized.
Semantic Interoperability: Achieving seamless integration and interoperability between different semantic technologies and platforms is a challenge. The development of standards and protocols for semantic data exchange and integration is necessary to enable effective knowledge sharing and collaboration.
Ethical Considerations: Semantic technologies raise ethical concerns related to privacy, consent, and data ownership. It is essential to implement mechanisms that protect the privacy and security of sensitive knowledge while still enabling the extraction of useful insights.
Looking into the future, semantic technologies are expected to play an increasingly important role in knowledge management within the AI era. Advancements in natural language processing, machine learning, and semantic reasoning hold the potential to further improve the capabilities of AI systems in understanding, organizing, and utilizing knowledge. Additionally, the integration of semantic technologies with emerging technologies like blockchain and distributed ledgers can enable secure and transparent knowledge exchange and verification.
In conclusion, semantic technologies enhance knowledge management in AI systems by providing a rich semantic layer that enables effective knowledge representation, discovery, sharing, and collaboration. By leveraging ontologies, semantic graphs, and semantic search techniques, AI systems can extract meaningful insights from diverse data sources, leading to more accurate and comprehensive knowledge utilization. Despite the challenges, the future of semantic technologies looks promising, with potential for further advancements in various domains that leverage the power of AI and knowledge management.
### 7.5 AI-Driven Knowledge Management Platforms
In the era of artificial intelligence (AI), knowledge management has become an essential component of organizations' strategies. The ability to effectively capture, organize, share, transfer, and apply knowledge is crucial for staying competitive and driving innovation. AI, with its advanced capabilities in data processing and analysis, has revolutionized knowledge management practices, enabling organizations to leverage their vast amounts of data and extract actionable insights. AI-driven knowledge management platforms have emerged as powerful tools to enhance collaboration, facilitate decision-making, and drive organizational learning.
AI-driven knowledge management platforms leverage various AI technologies to automate and optimize knowledge management processes. These platforms harness the power of machine learning, natural language processing (NLP), computer vision, and other AI techniques to unlock the value hidden within organizational data and create a knowledge-driven ecosystem. Let's explore some key features and benefits of AI-driven knowledge management platforms:
Advanced Knowledge Discovery:
AI-driven knowledge management platforms utilize machine learning algorithms to discover valuable insights from massive volumes of structured and unstructured data. By automatically extracting patterns, relationships, and trends, these platforms enable organizations to uncover hidden knowledge and enhance decision-making. Through advanced data mining techniques, AI-powered platforms can analyze diverse data sources, including documents, emails, databases, and social media, to identify relevant information and discover new knowledge.
Intelligent Knowledge Capture and Organization:
AI-driven knowledge management platforms automate the capture and organization of knowledge. Through NLP and machine learning models, these platforms can analyze and extract information from various sources, including text, audio, and video. By automatically categorizing and tagging content, they make it easier to search and retrieve relevant knowledge. Intelligent knowledge organization techniques, such as semantic analysis and entity recognition, enhance the accuracy and efficiency of knowledge capture, enabling organizations to manage information effectively.
Enhanced Knowledge Sharing and Collaboration:
One of the key benefits of AI-driven knowledge management platforms is their ability to facilitate knowledge sharing and collaboration among team members. These platforms provide advanced collaboration tools, such as discussion forums, chatbots, and wikis, to foster knowledge exchange. By leveraging AI technologies, such as natural language understanding and sentiment analysis, these platforms can improve communication and enable real-time knowledge sharing. Organizations can harness collective intelligence and promote a culture of collaboration through these AI-powered platforms.
Efficient Knowledge Transfer and Application:
AI-driven knowledge management platforms accelerate the transfer and application of knowledge across the organization. They provide intelligent recommendation systems that suggest relevant knowledge based on user preferences and context. By analyzing user behavior and historical data, these platforms can identify patterns and recommend relevant content. This facilitates the transfer of knowledge from experts to novices, ensures the continuity of knowledge within the organization, and enables employees to make informed decisions based on accurate and up-to-date information.
AI-Enabled Expertise Location:
AI-driven knowledge management platforms can help organizations identify subject matter experts within the organization. By analyzing the expertise profiles of employees, including their skills, experiences, and contributions, these platforms can provide recommendations for connecting employees with specific knowledge needs. This capability fosters a collaborative environment where employees can easily access the right expertise and tap into the collective knowledge of the organization.
Continuous Learning and Improvement:
AI-driven knowledge management platforms enable continuous learning and improvement by capturing and analyzing user feedback and interactions. These platforms can leverage AI algorithms to identify knowledge gaps, monitor knowledge usage and relevance, and provide insights for improving knowledge content. By analyzing user behavior and feedback, these platforms can adapt and personalize knowledge recommendations, ensuring that users receive the most relevant and valuable information.
Integration with Existing Systems:
AI-driven knowledge management platforms can integrate with existing enterprise systems, such as customer relationship management (CRM) systems, project management tools, and learning management systems (LMS). This integration allows organizations to leverage their existing data and workflows, resulting in a seamless and efficient knowledge management process. By accessing and analyzing data from different systems, these platforms can provide a comprehensive view of knowledge across the organization.
In conclusion, AI-driven knowledge management platforms have transformed the way organizations manage and leverage knowledge in the AI era. These platforms enable organizations to discover valuable insights, capture and organize knowledge intelligently, enhance collaboration and knowledge sharing, facilitate knowledge transfer and application, locate subject matter experts, and enable continuous learning and improvement. By leveraging AI technologies, organizations can extract maximum value from their vast amounts of data and unlock the potential of their knowledge resources. AI-driven knowledge management platforms empower organizations to make data-driven decisions, drive innovation, and stay competitive in the ever-evolving AI landscape.
## 8. Building AI-Powered Knowledge Repositories
### 8.1 Data Collection and Preprocessing
Data collection and preprocessing are crucial steps in building AI-powered knowledge repositories. These processes involve gathering relevant data from various sources and transforming it into a format suitable for analysis and storage. In the era of artificial intelligence (AI), the integration of fractal patterns into data collection and preprocessing techniques can enhance the efficiency and effectiveness of knowledge management.
Data Collection
Data collection is the initial step in the knowledge management process. In the AI era, vast amounts of data are generated from diverse sources such as sensors, social media, websites, and other digital platforms. Fractal-based approaches can contribute to the efficient collection of data by providing innovative techniques for capturing complex and self-similar patterns.
Fractal geometry offers a unique perspective on data acquisition through the concept of self-similarity. Fractals exhibit patterns that repeat at different scales, allowing for more comprehensive data collection. For example, in the context of social media analytics, a fractal-based approach can be used to collect data from various social media platforms while capturing both macro-level trends and micro-level details.
Furthermore, fractal-based data collection methods can enable the collection of data that is representative of both structured and unstructured information. By leveraging fractal patterns, AI-powered systems can efficiently extract and organize relevant data regardless of its format, allowing for a more comprehensive understanding of knowledge domains.
Data Preprocessing
Data preprocessing involves transforming raw data into a suitable format for further analysis and knowledge management. This step plays a crucial role in improving data quality, reducing noise, and ensuring compatibility with AI algorithms. Fractal-based techniques can enhance data preprocessing by providing innovative methods for noise reduction, feature extraction, and data normalization.
One of the challenges in data preprocessing is handling noisy data that may contain outliers or irrelevant information. Fractals offer robust methods for noise reduction by incorporating self-similarity measures. By detecting and removing anomalous patterns in data, fractal-based algorithms can enhance the accuracy and reliability of knowledge repositories. This is particularly advantageous in domains such as finance or healthcare, where outliers can significantly impact decision-making processes.
In addition to noise reduction, fractal-based techniques can also facilitate feature extraction. Fractal analysis can uncover hidden patterns and structures within data, allowing for the identification of relevant features that contribute to knowledge discovery. By leveraging the self-similar nature of fractals, AI systems can extract meaningful features that represent the underlying structure of complex datasets. This enables more accurate analysis and interpretation of knowledge.
Moreover, data normalization is a critical preprocessing step to ensure that data is consistent and comparable across different sources. Fractal-based normalization techniques can be applied to adjust data ranges and distributions, ensuring that data is standardized and suitable for subsequent analysis. By incorporating self-similar patterns into normalization processes, AI-powered systems can overcome challenges associated with non-uniform data distributions, enhancing the quality and reliability of knowledge repositories.
Fractals and Optimized Data Collection
Fractal-based approaches not only enhance data collection and preprocessing, but they also optimize these processes by minimizing redundant information and improving data efficiency. Fractals enable the identification and extraction of representative data samples that preserve the inherent structures and characteristics of the entire dataset.
By leveraging fractal patterns, AI systems can sample representative subsets of data that effectively capture the diversity and complexity of the entire dataset. This approach significantly reduces the amount of data required for analysis, resulting in more efficient storage and faster processing times. Additionally, fractal-based sampling methods can ensure that knowledge repositories are less susceptible to biases and outliers, leading to more accurate and reliable insights.
Furthermore, fractal-based techniques can also contribute to the optimization of data collection by dynamically adapting the data acquisition process. As new data becomes available, fractal-based algorithms can intelligently prioritize the collection of information that has the greatest potential for knowledge enhancement. This adaptive approach ensures that knowledge repositories are continuously updated with the most relevant and valuable data, improving the overall effectiveness of AI-driven knowledge management.
In conclusion, the integration of fractal patterns into data collection and preprocessing processes in the AI era offers significant benefits for knowledge management. Fractal-based techniques enable efficient and effective data acquisition, noise reduction, feature extraction, and data normalization. By leveraging self-similarity and complex patterns, AI systems can optimize data collection, leading to more accurate analysis, reliable insights, and enhanced knowledge repositories.
### 8.2 Knowledge Extraction and Representation
In the era of artificial intelligence, knowledge management plays a crucial role in harnessing the power of AI to extract and represent knowledge effectively. Knowledge extraction involves the process of identifying, capturing, and transforming relevant information from various sources into a structured format that AI algorithms can understand and process. On the other hand, knowledge representation focuses on organizing and encoding this information in a way that facilitates efficient retrieval, sharing, and application.
Understanding Knowledge Extraction
Knowledge extraction encompasses a range of techniques aimed at sifting through vast amounts of data to identify meaningful patterns, relationships, and insights. AI-powered algorithms enable automated extraction and analysis of information from both structured and unstructured data sources, such as documents, databases, websites, and social media.
One common approach to knowledge extraction is natural language processing (NLP), a subfield of AI that focuses on the interaction between computers and human language. NLP techniques involve parsing text data, understanding semantics, and extracting key information, such as entities, relationships, and events. For instance, entity recognition algorithms can identify and classify named entities mentioned in a text, such as people, places, organizations, or dates.
Another important technique in knowledge extraction is machine learning, which involves training algorithms to recognize and extract patterns from data. Supervised learning algorithms can be used to train models to identify specific information, such as key facts, sentiment, or topic classification, from text or other types of data. Unsupervised learning algorithms, on the other hand, can discover hidden patterns or groupings within the data without any predefined labels.
Furthermore, knowledge extraction can also leverage computer vision techniques to process image or video data. Image recognition algorithms can extract useful information from images, such as object detection or image classification, which can be further utilized in knowledge representation.
Representing Extracted Knowledge
Once knowledge is extracted from various sources, it needs to be represented in a structured format that can be easily understood and utilized by AI systems. Knowledge representation involves encoding information in a way that allows for efficient storage, retrieval, and analysis.
One popular approach to knowledge representation is the use of knowledge graphs. Knowledge graphs represent knowledge as a network of interconnected entities and relationships. They provide a flexible and intuitive way to organize and represent complex information by using nodes to represent entities and edges to represent relationships between them. Graph-based representation enables AI systems to traverse the network and infer new knowledge from existing relationships and patterns.
Semantic technologies also play a crucial role in knowledge representation. These technologies focus on capturing the meaning or semantics of information, allowing for more accurate and context-aware knowledge representation. Techniques such as ontologies, which define a set of concepts and their relationships, and semantic annotations, which provide additional metadata to describe the meaning of the data, enhance the representation and understanding of knowledge.
In addition to knowledge graphs and semantic technologies, other representation formats such as taxonomies, hierarchical structures, or vector embeddings can be used to capture and organize knowledge. The choice of representation format depends on the specific requirements of the AI application and the nature of the extracted knowledge.
Challenges and Opportunities
Knowledge extraction and representation present various challenges and opportunities in the AI era. One of the main challenges is the quality and accuracy of the extracted knowledge. AI algorithms are prone to errors and biases, which can impact the reliability of the knowledge extracted. Thus, ensuring the correctness and completeness of the extracted knowledge is crucial.
Another challenge is the scalability of knowledge extraction and representation. As the amount of available data continues to grow exponentially, AI systems need to handle large volumes of information in real-time. Efficient algorithms and infrastructure are necessary to process and represent knowledge in a timely manner.
Additionally, there is a need for standardization and interoperability in knowledge representation. AI systems often need to exchange and integrate knowledge across different platforms and domains. Common standards and formats help ensure compatibility and facilitate knowledge sharing and reuse.
On the other hand, knowledge extraction and representation present numerous opportunities for advancing AI-powered knowledge management. By effectively extracting and representing knowledge, organizations can enhance decision-making, improve problem-solving, and foster innovation. AI-enabled knowledge management systems can provide timely insights, help identify emerging patterns, and support knowledge-driven collaboration and learning.
Moreover, the combination of knowledge extraction and representation with other AI techniques, such as machine learning and deep learning, opens up new possibilities for knowledge discovery and analysis. By leveraging the power of AI algorithms, organizations can uncover hidden knowledge, gain new perspectives, and make predictions based on the extracted information.
In conclusion, knowledge extraction and representation are essential components of AI-driven knowledge management. These processes enable the transformation of raw data into meaningful and actionable knowledge. By leveraging AI techniques, such as natural language processing, machine learning, and computer vision, organizations can extract relevant information and encode it in structured formats, facilitating efficient knowledge storage, retrieval, and application. However, challenges related to data quality, scalability, and standardization need to be addressed to fully exploit the potential of knowledge extraction and representation in the AI era.
### 8.3 AI-Enabled Knowledge Repository Design
With the advancements in artificial intelligence (AI) and the increasing importance of knowledge management in the AI era, organizations are leveraging AI technologies to design and develop knowledge repositories that are more intelligent, efficient, and effective. These AI-enabled knowledge repositories play a crucial role in capturing, organizing, and leveraging vast amounts of information and insights to drive innovation and decision-making processes. In this section, we will explore the design principles and key considerations for building AI-powered knowledge repositories.
Understanding the Needs of Knowledge Management
Before delving into the specifics of designing an AI-enabled knowledge repository, it is essential to understand the core needs and requirements of knowledge management in the AI era. Effective knowledge management involves the creation, acquisition, storage, retrieval, sharing, collaboration, transfer, and application of knowledge within an organization. This involves not only capturing explicit knowledge (e.g., documents, reports, research papers) but also managing tacit knowledge (e.g., expertise, experience, insights) of individuals and teams.
AI technologies have the potential to transform the way knowledge is managed by automating various tasks and enhancing decision-making processes. AI-enabled knowledge repositories should be designed to leverage the power of AI algorithms to analyze, contextualize, extract, and represent knowledge in a way that is easily accessible and reusable by both humans and machines.
Knowledge Extraction and Representation
The first step in building an AI-enabled knowledge repository is the extraction and representation of knowledge. AI techniques, such as natural language processing (NLP) and machine learning (ML), can be employed to extract information from various sources, such as documents, databases, and unstructured data. NLP algorithms can analyze textual content to identify entities, relationships, and concepts, while ML algorithms can discover patterns and trends within the data.
Once the knowledge is extracted, it needs to be represented in a structured and semantically meaningful manner. Knowledge graphs, which are graphical representations of knowledge, can be employed to model the relationships and dependencies between different entities and concepts. This allows for efficient navigation, querying, and reasoning over the knowledge base.
Knowledge Organization and Categorization
An effective AI-enabled knowledge repository should facilitate the organization and categorization of knowledge to enable efficient retrieval and browsing. Traditional techniques, such as taxonomies and ontologies, can be combined with AI approaches to automate the categorization of knowledge. AI algorithms, such as clustering and classification, can analyze the content of documents and assign appropriate categories or tags based on the similarity of their content.
Furthermore, advanced techniques like topic modeling and sentiment analysis can be used to identify the key themes and sentiments present in the knowledge repository. This allows users to explore and discover relevant information based on their specific interests or requirements.
Contextualizing Knowledge with Metadata
To enhance the retrieval and understandability of knowledge, it is crucial to contextualize it with relevant metadata. Metadata provides additional information about the knowledge, such as its source, author, creation date, and usage statistics. AI techniques can be utilized to automatically extract metadata from various sources or generate it based on the content of the knowledge itself.
Additionally, AI-powered techniques, such as sentiment analysis and emotion recognition, can provide insights into the emotional context surrounding the knowledge. This can help users to understand the sentiment or biases associated with specific pieces of knowledge, enabling them to make more informed decisions.
Recommender Systems for Knowledge Discovery
AI-powered recommender systems can be integrated within the knowledge repository to facilitate knowledge discovery and serendipitous learning. These systems leverage user behavior, preferences, and historical interactions to recommend relevant content or suggest related knowledge items. By analyzing patterns in the usage and access of knowledge, AI algorithms can personalize the recommendations, ensuring that users are presented with the most relevant and valuable information.
Furthermore, AI techniques like semantic search and entity recognition can be employed to improve the accuracy and specificity of search results within the knowledge repository. This enables users to find relevant knowledge quickly and efficiently.
Continuous Learning and Improvement
An AI-enabled knowledge repository should not be a stagnant system but should continuously learn and improve based on user feedback and interactions. AI algorithms, such as reinforcement learning, can be employed to train the system to make better recommendations, optimize search results, and adapt to changing user preferences.
Moreover, techniques like active learning can be used to involve users in the process of knowledge curation and refinement. By soliciting feedback and annotations from users, the repository can improve its accuracy and quality over time.
Final Thoughts
Designing an AI-enabled knowledge repository involves leveraging AI techniques to extract, represent, organize, and contextualize knowledge in a meaningful way. By combining the power of AI with the principles of knowledge management, organizations can create repositories that enable efficient knowledge discovery, collaboration, and decision-making in the AI era. However, it is essential to ensure ethical considerations, data privacy, and security when implementing AI technologies in knowledge management systems to maintain trust and protect sensitive information.
### 8.4 Knowledge Validation and Maintenance
In the era of Artificial Intelligence (AI), where vast amounts of data are being collected and processed, ensuring the quality and accuracy of knowledge is crucial for effective decision-making and problem-solving. Knowledge validation and maintenance play a significant role in AI-powered knowledge repositories, ensuring that the information stored is reliable, up-to-date, and relevant. In this section, we will explore the importance of knowledge validation and maintenance in AI systems and discuss various techniques and strategies used to ensure the integrity and reliability of knowledge.
The Importance of Knowledge Validation
Knowledge validation is the process of evaluating and verifying the accuracy, completeness, and reliability of the information stored in AI-powered knowledge repositories. It is a critical step to ensure that the knowledge available for AI systems and decision-makers is trustworthy and free from errors or biases. By validating knowledge, organizations can mitigate the risks of making flawed decisions or relying on inaccurate information.
In the AI era, where data is often obtained from various sources, knowledge validation becomes even more crucial. The sheer volume, velocity, and variety of data require rigorous validation processes to ensure the integrity of knowledge. Additionally, as AI systems continuously learn and adapt, it is necessary to validate knowledge regularly to address any inconsistencies or changes in the underlying data.
Techniques for Knowledge Validation
There are several techniques and methods used for knowledge validation in AI systems. Let's explore some of the most common approaches:
1. Expert Review
Expert review involves the involvement of domain experts or subject matter experts (SMEs) who evaluate the knowledge stored in AI systems. These experts possess deep knowledge and expertise in the relevant domain and can provide valuable insights to validate the accuracy, relevance, and completeness of the information. Expert review is particularly useful in complex and specialized domains where the expertise of humans is essential.
2. Statistical Analysis
Statistical analysis is often employed to validate knowledge by examining its consistency and patterns within the dataset. Techniques such as data profiling, outlier detection, and correlation analysis can help identify discrepancies or inconsistencies in the data. By analyzing the statistical properties of the knowledge, organizations can gain insights into the quality and reliability of the information.
3. Cross-Referencing and External Validation
Cross-referencing involves comparing the knowledge stored in AI systems with external sources such as reputable databases, scientific literature, or industry standards. By cross-checking the information, organizations can verify its accuracy and validate its reliability. Additionally, external validation provides an opportunity to incorporate the latest research or updates into the knowledge repository.
4. Data Cleansing and Filtering
Data cleansing and filtering techniques are used to eliminate noise, errors, or irrelevant information from the dataset. This process involves identifying and removing duplicate records, correcting data inconsistencies, and handling missing or incomplete data. By ensuring the data quality, organizations can improve the reliability and accuracy of the knowledge stored in AI systems.
5. Feedback from Users
Users play a vital role in knowledge validation and maintenance. By allowing users to provide feedback on the knowledge they interact with, organizations can identify potential errors, gaps, or outdated information. User feedback can be collected through various mechanisms such as surveys, ratings, comments, or direct notifications. This feedback loop helps in continuously improving and updating the knowledge repository.
Strategies for Knowledge Maintenance
Knowledge maintenance involves the ongoing management, updating, and refinement of knowledge stored in AI-powered systems. To ensure that the knowledge remains relevant and accurate, organizations need to establish effective strategies and processes. Here are some strategies for knowledge maintenance:
1. Continuous Monitoring
Continuous monitoring of the knowledge repository helps detect any changes, inconsistencies, or inaccuracies in the data. By employing automated monitoring systems, organizations can proactively identify and address issues before they impact decision-making or system performance. Monitoring can be done through automated alerts, anomaly detection techniques, or periodic audits.
2. Regular Updates
Regular updates are essential to keep the knowledge repository up-to-date and aligned with the latest research, industry standards, or best practices. Organizations should establish processes to review and incorporate new information or insights into the knowledge repository. This can be done through scheduled updates, versioning, or automated synchronization with external data sources.
3. Collaboration and Crowdsourcing
Leveraging the collective intelligence of users and experts can significantly contribute to knowledge maintenance. Organizations can encourage collaboration and crowdsourcing by providing platforms for users to contribute, verify, or update the knowledge repository. By integrating user-generated content and expert contributions, organizations can enhance the accuracy and relevance of the knowledge.
4. Governance and Documentation
Establishing governance mechanisms and documentation processes are crucial for knowledge maintenance. Clear ownership, roles, and responsibilities should be defined to ensure accountability. Documentation should include details on the validation processes, update cycles, and any modifications made to the knowledge repository. This documentation helps maintain transparency, traceability, and auditability.
5. Machine Learning and Automation
Machine learning techniques can be applied to automate the knowledge maintenance processes. AI algorithms can continuously analyze data patterns and identify inconsistencies or outdated information. By utilizing machine learning and automation, organizations can streamline knowledge maintenance and reduce the manual effort involved.
Conclusion
Knowledge validation and maintenance are critical aspects of AI-powered knowledge repositories. Ensuring the accuracy, reliability, and relevance of knowledge is essential for efficient decision-making and problem-solving in the AI era. By employing techniques such as expert review, statistical analysis, cross-referencing, and user feedback, organizations can validate the knowledge and identify any discrepancies. Implementing strategies like continuous monitoring, regular updates, collaboration, and automation further enhance the maintenance of knowledge repositories. By prioritizing knowledge validation and maintenance, organizations can unleash the full potential of AI in knowledge management and drive successful outcomes.
### 8.5 Real-World Examples of AI-Powered Knowledge Repositories
In the AI era, organizations are increasingly leveraging AI-powered knowledge repositories to enhance their knowledge management practices. These repositories serve as centralized platforms that collect, store, organize, and share vast amounts of information while using artificial intelligence techniques to extract valuable insights. In this section, we will explore some real-world examples of AI-powered knowledge repositories and how they contribute to effective knowledge management.
1. IBM Watson
One of the most well-known AI-powered knowledge repositories is IBM Watson. Watson is a cognitive computing system developed by IBM that combines artificial intelligence, natural language processing, and machine learning capabilities. Watson provides organizations with a comprehensive platform to manage and retrieve vast amounts of structured and unstructured data. By applying AI algorithms to categorize and analyze information, Watson enables users to extract valuable insights and make data-driven decisions. Watson's ability to understand and process natural language queries makes it particularly useful in knowledge management, as it allows users to access relevant information using plain language.
2. Google Knowledge Graph
Google Knowledge Graph is another example of an AI-powered knowledge repository that has revolutionized knowledge management on the internet. The Knowledge Graph is a vast database of interconnected information that powers Google's search engine and other Google services. By analyzing billions of web pages, structured data sources, and user behavior patterns, the Knowledge Graph provides users with accurate and relevant information through rich snippets, knowledge cards, and featured snippets. These AI-driven features enhance the user experience by presenting concise and contextual information directly on the search results page, reducing the need for users to visit multiple websites to find the information they need.
3. Microsoft Azure Cognitive Search
Microsoft Azure Cognitive Search is a cloud-based AI-powered knowledge repository that enables organizations to build scalable and intelligent search solutions. It combines AI technologies such as natural language processing, machine learning, and computer vision to index and analyze vast amounts of data across multiple sources, including documents, databases, and websites. With Azure Cognitive Search, organizations can create powerful search experiences that retrieve relevant information quickly and accurately. The platform's advanced AI capabilities allow for the extraction of insights from unstructured data, enabling organizations to gain a deeper understanding of their knowledge assets and make more informed decisions.
4. Amazon AWS Knowledge Center
Amazon AWS Knowledge Center is an AI-powered knowledge repository provided by Amazon Web Services, designed to help users find answers to their technical questions. The Knowledge Center uses natural language processing and machine learning algorithms to analyze and categorize a wide range of technical articles, FAQs, and troubleshooting guides. By understanding the context of user queries, the Knowledge Center provides accurate recommendations and suggests relevant articles to resolve specific issues. Through continuous learning and feedback mechanisms, the system improves its accuracy over time, ensuring that users can efficiently access the information they need.
5. Salesforce Einstein Knowledge
Salesforce Einstein Knowledge is an AI-powered knowledge repository integrated into the Salesforce platform. Einstein Knowledge enables organizations to capture, manage, and deliver knowledge across various channels, providing consistent and personalized experiences for customers and employees. By leveraging natural language processing and machine learning techniques, Einstein Knowledge understands user queries and suggests the most relevant information from a vast repository of articles, FAQs, and documentation. The platform also facilitates knowledge collaboration and feedback loops, allowing subject matter experts to contribute and update knowledge resources in real-time.
These real-world examples demonstrate the power of AI-powered knowledge repositories in enhancing knowledge management practices. By using advanced AI technologies, these platforms enable organizations to transform vast amounts of information into actionable insights, improving decision-making, productivity, and customer experiences. As AI continues to advance, we can expect the development of more sophisticated AI-powered knowledge repositories that will further revolutionize the way organizations manage and leverage knowledge in the AI era.
## 9. Fractal Visualization and Analysis in AI
### 9.1 Visualization Techniques for Fractal Data
In the era of artificial intelligence (AI), where massive amounts of data are generated and processed, the visualization of complex data sets becomes crucial for understanding and extracting meaningful insights. When dealing with fractal data, the challenge of visualizing intricate patterns and structures becomes even more pronounced. Visualization techniques for fractal data play a key role in unraveling the hidden complexities and uncovering the underlying relationships within these data sets.
Fractals, with their self-similar and infinitely detailed structures, have unique properties that require specialized visualization approaches. These techniques enable us to explore the intricate patterns and understand the complex properties of fractal data. By visualizing fractal data, researchers, scientists, and knowledge managers can gain valuable insights into the underlying dynamics and relationships within the data.
Fractal Visualization Methods
Fractal visualization techniques aim to represent the complex patterns and structures inherent in fractal data in a visually appealing and comprehensible manner. These methods leverage various visualization techniques and tools to present fractal data in an intuitive and informative way. Here are some commonly used visualization methods for fractal data:
2D Visualization: This technique is used to represent fractal data in a two-dimensional space. It enables the visualization of fractal patterns on a flat surface, such as a computer screen or a printed surface. Techniques such as color mapping, contour plots, and density plots are employed to highlight the details and visualize the variations within the fractal data.
3D Visualization: Three-dimensional visualization methods provide an immersive and interactive representation of fractal data. By adding an additional dimension, the visual depiction of fractal patterns becomes more tangible and easier to comprehend. Techniques like volume rendering, surface rendering, and isosurface extraction can be used to bring the intricate details of fractal data to life in a three-dimensional space.
Multidimensional Visualization: Fractal data often consists of multiple variables or dimensions. To visualize such complex data, multidimensional visualization techniques are employed. These techniques allow for the visualization of several dimensions simultaneously, enabling a comprehensive understanding of the relationships and dependencies within the fractal data. Methods like parallel coordinates, scatterplot matrices, and hypercube visualization are commonly used in this context.
Interactive Visualization: Interactive visualization techniques empower users to explore and interact with fractal data in real-time. These methods provide a dynamic and immersive environment for users to manipulate and navigate through the data, allowing for a more intuitive understanding of the underlying patterns and relationships. Techniques such as zooming, panning, filtering, and brushing enable users to focus on specific regions of interest and extract meaningful insights from the fractal data.
Animation: Animation techniques are utilized to visualize the dynamic aspects of fractal data. By representing the temporal evolution of fractal patterns through animation, researchers can observe how the patterns change over time and gain insights into the underlying processes. Animation techniques can reveal hidden dynamics, cyclical patterns, and emergent behaviors within fractal data, providing a deeper understanding of the phenomena being studied.
Virtual Reality (VR) Visualization: VR visualization offers an immersive and interactive experience that allows users to explore fractal data in a three-dimensional virtual environment. This technique provides a unique perspective on the fractal structures, enabling users to navigate through the data and gain a deeper understanding of the complex relationships within it. VR visualization of fractal data can provide a sense of scale, depth, and immersion, enhancing the comprehension and analysis of the data.
Application of Visualization Techniques in Knowledge Management
Visualization techniques for fractal data have significant implications for knowledge management in the AI era. They facilitate the exploration and analysis of complex data sets, enabling knowledge managers to uncover hidden patterns, identify trends, and extract valuable insights. Here are some ways visualization techniques can enhance knowledge management:
Decision Making: Visualizing fractal data aids decision-makers in understanding complex information and making informed decisions. By representing data visually, decision-makers can identify critical factors, detect anomalies, and assess the impact of various variables on outcomes. Visualization techniques enable knowledge managers to present data in a visually intuitive manner, enhancing the decision-making process and improving the quality of decisions.
Knowledge Discovery: Visualization techniques facilitate the exploration and discovery of new knowledge within fractal data. By visually analyzing the patterns, relationships, and trends present in the data, knowledge managers can identify valuable insights that were previously hidden or overlooked. Visualization helps knowledge managers identify patterns, correlations, and outliers, leading to the discovery of new knowledge and the identification of potential areas of innovation.
Collaboration and Communication: Visualizations provide a common language for knowledge managers to communicate and collaborate effectively. By visualizing complex data sets, knowledge managers can communicate ideas and insights more clearly, enabling better collaboration among team members. Visualization techniques promote shared understanding and facilitate knowledge sharing, leading to improved teamwork and more efficient knowledge management processes.
Knowledge Sharing and Dissemination: Visualization techniques can transform complex fractal data into easily understandable visual representations. This enables knowledge managers to share knowledge with a wider audience, including non-experts or decision-makers. By presenting information visually, knowledge managers can effectively convey complex ideas and findings to diverse stakeholders, promoting knowledge dissemination and driving organizational learning.
Pattern Recognition and Anomaly Detection: Visualization techniques can aid in the identification of patterns and anomalies within fractal data. By visually exploring the data, knowledge managers can detect unusual behaviors, outliers, or unexpected patterns that may require further investigation. Visualization enhances the ability to recognize and understand complex patterns, leading to improved anomaly detection and better-informed decision-making.
In the era of AI, where vast amounts of complex data are generated and processed, visualization techniques for fractal data play a vital role in knowledge management. These techniques empower knowledge managers to transform complex data into visually intuitive representations, enabling a deeper understanding of the underlying patterns and relationships. By leveraging visualization techniques, knowledge managers can unlock valuable insights, facilitate collaboration, and make informed decisions that drive organizational success in the AI era.
### 9.2 Analyzing Fractal Patterns using AI
In the era of artificial intelligence (AI), the analysis of fractal patterns has emerged as a powerful tool for understanding complex phenomena. Fractals are intricate geometric shapes that exhibit self-similarity at different scales, and they can be found in various natural and man-made structures. By harnessing the analytical capabilities of AI, we can delve deeper into the world of fractals and gain valuable insights into the underlying patterns and structures.
AI techniques such as machine learning and deep learning have the ability to process vast amounts of data and uncover hidden patterns that may not be apparent to the naked eye. When applied to fractal analysis, AI algorithms can enhance our understanding of complex systems and provide us with valuable information about their behavior and characteristics. Here, we explore how AI can be used to analyze fractal patterns and the potential benefits it offers to knowledge management in the AI era.
Analyzing Fractal Dimension
One of the key aspects of fractals is their dimensionality, which differs from traditional Euclidean geometry. Fractals often exhibit non-integer dimensions, known as fractal dimensions, which quantitatively represent their level of complexity or intricacy. By analyzing the fractal dimensions of a given dataset or system, AI algorithms can help us gain a deeper understanding of its underlying structure.
Machine learning algorithms can be trained to analyze and predict the fractal dimensions of different patterns. This can be done by feeding the algorithms with labeled training data consisting of known fractal patterns and their corresponding dimensions. Once trained, the AI model can then be applied to new datasets to determine their fractal dimensions and classify them accordingly. This analysis can aid in identifying hidden patterns, detecting anomalies, and characterizing the complexity of various phenomena.
Pattern Recognition and Classification
Fractals exhibit self-similarity, meaning that smaller components of a fractal resemble the larger whole. This property makes them excellent candidates for pattern recognition and classification tasks. By utilizing AI techniques such as convolutional neural networks (CNNs) in computer vision, we can train models to recognize and classify fractal patterns in images, videos, or other visual data.
Through the use of labeled training data, AI algorithms can learn to identify specific types of fractal patterns and classify them into different categories. For example, in medical imaging, AI-powered algorithms can detect and classify fractal patterns in X-rays, MRIs, or CT scans, aiding in the diagnosis of various diseases. In the field of finance, AI can analyze stock market data and identify fractal patterns that may indicate future price movements. By combining the power of AI and the inherent complexity of fractals, we can uncover valuable insights and make more accurate predictions.
Data Visualization and Interpretability
Fractals possess intricate and visually appealing structures that can be challenging to interpret without the aid of advanced visualization tools. AI techniques can contribute to the visualization and interpretation of fractal patterns by utilizing generative models, such as generative adversarial networks (GANs), to generate realistic fractal images or animations.
By training a GAN on a dataset of known fractal patterns, the AI model can learn the underlying rules that govern the generation of fractals. Once trained, the GAN can generate new fractal images that resemble the training data, allowing us to explore and visualize the vast space of possible fractal structures. This visualization not only aids in understanding the intricacies of fractal geometry but also provides a means for exploring new and unexplored areas of knowledge.
Predictive Modeling and Forecasting
AI algorithms are well-suited for predictive modeling and forecasting tasks, and when combined with the analysis of fractal patterns, they can provide valuable insights into the behavior and evolution of complex systems. By analyzing the temporal evolution of fractal patterns, AI models can learn the underlying dynamics and make predictions about future developments.
For instance, in environmental science, AI can analyze the fractal patterns of weather data to predict climate trends and identify potential extreme events such as hurricanes or heatwaves. In the realm of stock market prediction, fractal analysis coupled with AI algorithms can help forecast market trends and assist in making informed investment decisions. Through the integration of AI with the analysis of fractal patterns, we can gain a deeper understanding of the complex systems that surround us and make more accurate predictions about their future behavior.
Conclusion
The analysis of fractal patterns using AI techniques opens up new possibilities for knowledge management in the AI era. By leveraging the power of AI, we can delve deeper into the complexities of fractals and gain valuable insights that can be applied to various domains. From analyzing fractal dimension to pattern recognition and classification, data visualization and interpretability, and predictive modeling and forecasting, AI enhances our understanding of fractal systems and helps us make more informed decisions. As we continue to explore the synergies between AI and fractals, we can look forward to further advancements in knowledge management and the unlocking of new frontiers in the world of artificial intelligence.
### 9.3 Fractal-Based Anomaly Detection
Anomaly detection is a critical task in many domains, including finance, cybersecurity, and industrial monitoring. Traditional approaches to anomaly detection often rely on predetermined thresholds or statistical methods that may not capture complex and dynamic patterns in the data. In the era of artificial intelligence (AI), the integration of fractal analysis techniques with anomaly detection algorithms opens up new possibilities for detecting and understanding anomalies in complex datasets.
Fractals, with their self-similarity and recursive patterns, are particularly well-suited for capturing the intricate structures of real-world data. By leveraging the power of AI, fractal-based anomaly detection algorithms can automatically learn and adapt to the underlying patterns of the data, leading to more accurate and robust anomaly detection.
One of the key advantages of fractal-based anomaly detection is its ability to identify anomalies in both spatial and temporal dimensions. Fractal analysis can reveal hidden patterns and irregularities in the data that may not be apparent to traditional statistical methods. By encoding the self-similar characteristics of the data into the anomaly detection model, it becomes possible to detect anomalies at different scales and resolutions.
Fractal-based anomaly detection algorithms can be applied to a wide range of applications. In finance, for example, these algorithms can help identify fraudulent transactions, market manipulations, or abnormal trading patterns. By analyzing the fractal dimensions and patterns of financial time series data, anomalies such as sudden market crashes or speculative bubbles can be detected and flagged for further investigation.
In cybersecurity, fractal-based anomaly detection can play a crucial role in identifying malicious activities or abnormal network behaviors. By analyzing network traffic or system logs, these algorithms can detect patterns that deviate from normal behavior and raise alerts. This can help prevent cybersecurity breaches, detect intrusion attempts, and protect sensitive data.
In industrial monitoring and predictive maintenance, fractal-based anomaly detection can be used to identify equipment failures, abnormal sensor readings, or production deviations. By analyzing the fractal patterns in sensor data or process parameters, anomalies can be detected early, allowing for timely interventions and preventing costly downtime.
Fractal-based anomaly detection algorithms can also be applied to image or video analysis tasks in computer vision. By examining the fractal characteristics of images or video frames, anomalies such as object occlusions, unusual movements, or tampering can be detected. This has important applications in surveillance, video analytics, and forensic investigations.
To implement fractal-based anomaly detection, machine learning algorithms such as support vector machines (SVM), k-nearest neighbors (KNN), or neural networks can be used. These algorithms can be trained on a labeled dataset containing both normal and anomalous samples. During training, the algorithms learn the underlying patterns and structures of the data, allowing them to differentiate between normal and anomalous instances. Once trained, the models can be used to classify new, unseen data and identify potential anomalies.
It is worth noting that the success of fractal-based anomaly detection relies on the availability of quality data. To achieve accurate and reliable results, it is essential to preprocess the data, remove noise, and normalize it appropriately. Additionally, feature engineering techniques may be employed to extract relevant features that capture the intrinsic fractal characteristics of the data.
Fractal-based anomaly detection is not without its challenges. The complexity and computational demands of fractal analysis can be considerable, especially for large-scale or high-dimensional datasets. Efficient algorithms and computational techniques need to be developed to handle the computational burden and ensure real-time anomaly detection.
Furthermore, the interpretability of fractal-based anomaly detection models may pose a challenge. While these models can accurately detect anomalies, understanding the underlying reasons behind these deviations may require domain expertise or additional investigations. Therefore, combining the power of fractal-based anomaly detection with explainable AI techniques could enhance the transparency and trustworthiness of the results.
In conclusion, combining fractal analysis with AI-based anomaly detection algorithms provides a powerful approach to detecting anomalies in complex datasets. The self-similar and recursive nature of fractals enables the capture of intricate patterns and structures in the data, leading to accurate and robust anomaly detection. Fractal-based anomaly detection has applications in various domains, including finance, cybersecurity, industrial monitoring, and computer vision. However, addressing computational challenges and ensuring model interpretability are important areas of future research in this field. By harnessing the potential of fractals and AI, organizations can gain valuable insights into their data, detect anomalous behaviors, and make informed decisions to mitigate risks and enhance operational efficiency.
### 9.4 Predictive Analytics with Fractal Insights
Predictive analytics is a powerful approach that utilizes historical data and statistical algorithms to make predictions about future events or outcomes. In the era of artificial intelligence (AI), predictive analytics has gained significant attention and has become an essential component of knowledge management. Fractal insights, with their inherent self-similarity and complexity, have proven to be a valuable asset in enhancing the accuracy and effectiveness of predictive analytics in AI systems.
Fractals, as we have explored throughout this book, are intricate geometric patterns that exhibit self-similarity, meaning that they appear similar at different scales. This self-similarity property makes fractals an excellent tool for analyzing and understanding complex phenomena in various domains, including AI. Fractal insights provide a deeper understanding of intricate relationships and patterns within data, enabling more accurate prediction models.
One way in which fractal insights contribute to predictive analytics in AI is by providing a richer understanding of the underlying data distribution. Traditional statistical models often assume that the data follows a specific distribution, such as a normal distribution. However, real-world data often deviates from such assumptions, displaying complex patterns and irregularities. By incorporating fractal insights into predictive models, AI systems can capture the intricate nature of the data distribution, resulting in more accurate predictions.
Fractals can also be leveraged in feature engineering, a crucial step in predictive analytics where relevant features are extracted from raw data. Traditional feature engineering methods rely on manual selection or transformation of features based on domain expertise. However, this approach may miss out on important patterns and relationships buried within the data. By utilizing fractal insights, AI systems can reveal hidden features and relationships that may not be apparent through traditional approaches. This enables the model to capture more comprehensive and relevant information, leading to improved predictive performance.
Another area where fractal insights contribute to predictive analytics is in time series forecasting. Time series data, characterized by the sequential nature of observations, is prevalent in many domains, such as finance, healthcare, and weather forecasting. Fractal patterns exist in time series data, and understanding these patterns can lead to valuable insights for making accurate predictions. Fractal analysis techniques, such as fractal dimension estimation and detrended fluctuation analysis, can uncover hidden patterns and long-range dependencies within time series data, allowing AI systems to make more accurate predictions about future trends and fluctuations.
In addition, fractal insights can enhance the interpretability of predictive models in AI systems. Black-box models, such as deep neural networks, are known for their high predictive accuracy but lack transparency. Understanding the underlying reasoning behind their predictions can be challenging. By incorporating fractal insights into the model development process, AI systems can generate more interpretable models. Fractal analysis provides a visual representation of complex patterns, enabling human users to comprehend the decision-making process of the AI system and gain insights into the factors driving predictions.
Furthermore, fractal insights can be leveraged in anomaly detection, a critical task in predictive analytics that aims to identify abnormal or unusual events in a dataset. Anomalies often represent deviations from normal patterns and can be indicative of critical events, such as cybersecurity breaches or equipment failures. Fractal-based anomaly detection methods can identify subtle deviations from regular patterns, enhancing the system's ability to detect and predict anomalies with higher accuracy. By understanding the fractal structure of the underlying data, AI systems can distinguish between normal and abnormal patterns, enabling proactive action to mitigate potential risks.
In summary, predictive analytics plays a crucial role in AI-driven knowledge management. By incorporating fractal insights into predictive models, AI systems can enhance their accuracy, improve feature engineering, analyze complex time series data, increase interpretability, and improve anomaly detection. Fractals provide a powerful lens through which AI systems can gain a deeper understanding of the underlying data, enabling more accurate predictions and valuable insights. As AI continues to evolve, the integration of fractal-based predictive analytics will remain vital for knowledge management in the AI era.
## 10. Challenges and Future Directions
### 10.1 Ethical Challenges in AI-Driven Knowledge Management
As artificial intelligence (AI) continues to advance and transform various industries, including knowledge management, it brings with it a set of ethical challenges that need to be addressed. The integration of AI into knowledge management systems has the potential to revolutionize how organizations collect, store, analyze, and share knowledge. However, the ethical concerns associated with AI-driven knowledge management cannot be ignored.
AI Bias and Fairness
One of the main ethical challenges in AI-driven knowledge management is the issue of bias and fairness. AI systems are often trained on large amounts of data, which can inadvertently include biases from historical and societal patterns. These biases can manifest in the form of discriminatory or prejudiced outcomes, perpetuating existing inequalities and biases. For example, if an AI system is used to filter job applications, it may unknowingly favor certain demographic groups over others. This can lead to unfairness and discrimination in the hiring process.
To address this challenge, organizations must ensure that the datasets used to train AI models are diverse, representative, and free from biases. Additionally, continuous monitoring and auditing of AI systems can help detect and rectify any biased outcomes. Ethical guidelines and standards should also be developed to promote fairness and mitigate the risk of bias in AI-driven knowledge management.
Privacy and Security Concerns
With the integration of AI into knowledge management systems, privacy and security concerns become paramount. AI systems often rely on extensive data collection and analysis to make informed decisions. This can include personal and sensitive information, such as customer data, employee records, or intellectual property. If not properly secured, this data can be at risk of unauthorized access, misuse, or breach.
Organizations must prioritize data protection and implement robust security measures to safeguard sensitive information. This includes encryption, access controls, and regular vulnerability assessments. Furthermore, privacy regulations and compliance standards, such as the General Data Protection Regulation (GDPR), should be adhered to ensure the ethical handling of personal data within AI-driven knowledge management systems.
Transparency and Explainability
Another ethical challenge in AI-driven knowledge management is the lack of transparency and explainability. AI systems, particularly those based on deep learning and neural networks, can be highly complex and opaque in their decision-making processes. This lack of transparency can make it difficult for users and stakeholders to understand how a decision or recommendation was reached.
To address this challenge, organizations must ensure that AI systems are designed in a way that provides transparency and explainability. This can be achieved through the use of interpretable AI models, where the decision-making process is more easily understood. Additionally, organizations should invest in tools and technologies that enable post-hoc explanations for AI decisions, allowing users to understand and question the reasoning behind system outputs. Transparent and explainable AI-driven knowledge management systems can enhance trust and accountability.
Human-AI Collaboration and Autonomy
As AI becomes more integrated into knowledge management systems, the role of humans in decision-making processes may be diminished or altered. This raises ethical concerns related to the autonomy and agency of individuals. It is crucial to strike a balance between the capabilities of AI systems and the involvement of humans in decision-making, ensuring that the final decisions are accountable and aligned with human values.
Organizations must promote human-AI collaboration by involving human experts in the design, development, and evaluation of AI-driven knowledge management systems. Human oversight and intervention should be integrated into the decision-making process to prevent undue reliance on AI systems and mitigate potential risks. This collaborative approach upholds ethical principles and ensures that AI systems are used as tools to enhance human decision-making rather than replacing it.
Ensuring Ethical Use of AI
Finally, an overarching ethical challenge in AI-driven knowledge management is ensuring the ethical use of AI technologies. Organizations must establish clear policies, guidelines, and codes of conduct to govern the use of AI systems in knowledge management. This includes defining the boundaries of AI decision-making, establishing principles for responsible AI use, and establishing mechanisms for accountability and redress in case of ethical breaches.
Additionally, ongoing ethical training and education for employees involved in AI-driven knowledge management is crucial. Ensuring that individuals have a deep understanding of the ethical considerations and implications of AI can help foster a culture of responsible AI use and decision-making within organizations.
In summary, the integration of AI into knowledge management systems presents several ethical challenges that need to be addressed. From AI bias and fairness to privacy and security concerns, transparency and explainability to human-AI collaboration, organizations must navigate these challenges to ensure that AI-driven knowledge management is conducted ethically. By prioritizing fairness, privacy, transparency, collaboration, and responsible AI use, organizations can harness the full potential of AI while upholding ethical standards. The development of ethical guidelines, continuous monitoring, and regular ethical audits can help organizations navigate these challenges and ensure that AI-driven knowledge management operates in an ethical and responsible manner.
*[AI]: Artificial Intelligence *[GDPR]: General Data Protection Regulation
### 10.2 Privacy and Security Concerns
As the proliferation of Artificial Intelligence (AI) continues to shape the landscape of knowledge management (KM) in the era of AI, it is vital to address the privacy and security concerns that arise with the integration of these technologies. While AI-driven knowledge management has the potential to revolutionize how organizations create, organize, and share knowledge, it also raises significant ethical and practical challenges related to privacy and security.
10.2.1 Data Privacy in AI-Driven Knowledge Management
One of the primary concerns in AI-driven knowledge management is data privacy. With the increasing adoption of AI technologies, vast amounts of data are collected, stored, and analyzed to extract valuable insights. This data often encompasses sensitive and personal information, such as customer data, employee records, and intellectual property. It is crucial to ensure the privacy of this data, as any unauthorized access or misuse can have severe consequences.
To address privacy concerns, organizations must adopt robust data protection measures. This includes implementing strong access controls, encryption techniques, and anonymization methods to safeguard sensitive information. Additionally, organizations must comply with relevant data protection regulations, such as the General Data Protection Regulation (GDPR) in the European Union, to ensure that personal data is handled appropriately.
Furthermore, transparency is critical in AI-driven knowledge management. Organizations should provide clear and concise information about what data is being collected, how it is being used, and who has access to it. By being transparent with users and stakeholders, organizations can build trust and maintain a responsible approach to data privacy.
10.2.2 Security of AI Models and Algorithms
The security of AI models and algorithms is another significant concern in AI-driven knowledge management. As organizations heavily rely on AI-driven systems to analyze and interpret vast amounts of data, it becomes crucial to protect these models from malicious attacks or tampering.
Attackers may attempt to manipulate AI models with the intent to obtain unauthorized access to sensitive information or to introduce biased decision-making. Adversarial attacks, for example, involve subtly modifying inputs to mislead AI algorithms, leading to incorrect or biased outcomes. This poses a significant threat to the integrity and reliability of knowledge management systems.
To mitigate these risks, organizations should implement robust security measures at multiple levels. This includes securing the AI infrastructure, employing secure coding practices, and implementing intrusion detection and prevention systems. Regular auditing and testing of AI systems can help identify vulnerabilities and ensure their overall security.
10.2.3 Ensuring Ethical Use of AI in Knowledge Management
Apart from privacy and security concerns, the ethical use of AI in knowledge management plays a crucial role in building trust and fostering responsible AI deployment. Ethical considerations include the fair treatment of individuals through unbiased decision-making, avoiding AI-enabled discrimination, and maintaining transparency in the use of AI algorithms.
Bias in AI systems is a significant concern when it comes to knowledge management. AI models are trained on historical data, which may contain inherent biases or discriminatory patterns. When these biased models are used for knowledge management, it can perpetuate unfairness and exclusion, leading to incorrect recommendations or decisions. Therefore, organizations must strive to address bias in AI models and ensure fairness and inclusivity in their knowledge management practices.
Another ethical concern is the potential misuse of AI-driven knowledge management for surveillance or unethical purposes. Organizations should establish clear guidelines and frameworks that prevent the misuse of AI technology and protect individuals' rights to privacy and dignity. Regular audits and ethical assessments can help identify any potential misuse and ensure compliance with ethical standards.
10.2.4 Regulatory and Legal Considerations
In addition to ethical concerns, organizations must also navigate through various regulatory and legal considerations associated with AI-driven knowledge management. Compliance with data protection regulations is paramount to safeguarding data privacy. Organizations operating in different jurisdictions must adhere to relevant laws and regulations pertaining to data protection, such as GDPR, California Consumer Privacy Act (CCPA), or Personal Information Protection and Electronic Documents Act (PIPEDA) in Canada.
Moreover, organizations should stay updated with evolving legal frameworks related to AI and knowledge management. Legal implications, such as liability of AI systems, intellectual property rights, and the disclosure of AI-driven decision-making processes, need to be carefully considered and addressed.
Conclusion
As AI continues to reshape knowledge management practices, it is essential to address the privacy and security concerns that arise with its integration. Organizations must prioritize data privacy, secure their AI models and algorithms, ensure ethical use of AI, and navigate through regulatory and legal considerations. By adopting responsible and ethical AI-driven knowledge management practices, organizations can harness the full potential of AI while mitigating the associated risks.
### 10.3 Data Bias and Fairness in AI
As artificial intelligence (AI) continues to advance, it becomes increasingly important to address the issue of data bias and fairness in AI systems. Data bias refers to the existence of systematic errors in the data used to train machine learning models, which can result in unfair or discriminatory outcomes. Fairness, on the other hand, refers to the principle of treating all individuals or groups equitably, without favoring one over the other.
Data bias can occur in various stages of the AI lifecycle, including data collection, preprocessing, and model training. It can arise from a range of sources, such as biased data collection methods, inaccuracy or incompleteness of the data, or the influence of societal biases that manifest in the data. Data bias can have serious implications, as it can perpetuate and amplify existing prejudices and inequalities in society.
The repercussions of data bias in AI systems are extensive, affecting various domains such as employment, criminal justice, healthcare, and finance. For example, biased recruitment algorithms can perpetuate gender or racial discrimination by favoring certain groups over others. Similarly, biased criminal risk assessment algorithms can disproportionately target individuals from minority communities, leading to unjust outcomes in the justice system. Therefore, it is crucial to identify and mitigate data bias to ensure fairness and equity in AI applications.
Addressing data bias and achieving fairness in AI systems requires a combination of technical, ethical, and societal considerations. Here are some approaches and strategies that can help mitigate data bias and promote fairness:
1. Data Representation and Feature Selection
One way to combat data bias is to carefully analyze the dataset and examine the representation of different groups. It is important to ensure that the dataset is diverse and representative of the population being modeled. By consciously selecting features that are not discriminatory or biased, one can reduce the possibility of biased outcomes. Additionally, one can use techniques such as feature engineering or feature selection to minimize the impact of biased features in the dataset.
2. Regular Monitoring and Evaluation
Continuous monitoring and evaluation of AI systems are essential to identify and rectify any biases that may arise during system operation. Regular audits of the data and the models can help detect and correct biased patterns. By actively seeking out and addressing biases, organizations can ensure fairness in AI systems and avoid perpetuating discrimination.
3. Transparent and Explainable AI
To enhance fairness, it is crucial to develop AI models and systems that are transparent and explainable. By employing interpretable machine learning techniques, such as decision trees or rule-based models, it becomes easier to understand how the system arrives at its decisions. This not only enables better detection of biases but also provides a means for stakeholders to scrutinize the system and hold it accountable for any unfairness.
4. Diverse and Inclusive AI Development Teams
A diverse and inclusive development team can contribute to reducing data bias in AI systems. Including individuals from different backgrounds, ethnicities, genders, and perspectives can help identify and challenge biased assumptions and ensure that various viewpoints are considered during the development process. By fostering diversity, organizations can prevent the creation of biased AI systems and promote fairness and inclusivity.
5. Addressing Ethical Concerns
Data bias and fairness in AI also involve complex ethical considerations. Organizations and developers should actively engage in discussions and debates around ethical AI practices. Establishing ethical guidelines and frameworks for AI development, deployment, and usage can help identify and address potential biases. In addition, involving ethicists and experts in the development process can help navigate the ethical challenges arising from AI applications.
6. Regular Algorithmic Audits and Bias Testing
Algorithmic audits and bias testing are essential tools to assess the fairness of AI systems. These involve evaluating the performance of the system on different demographic groups to identify potential disparities and biases. By conducting regular tests and audits, organizations can rectify any biases and ensure that the AI system operates fairly for all individuals.
7. Data Governance and Data Responsibility
Safeguarding against data bias and ensuring fairness requires robust data governance practices. Organizations should establish clear guidelines and protocols for data collection, labeling, and preprocessing to minimize bias. Moreover, data responsibility involves considering the potential biases and consequences of using specific datasets and taking appropriate corrective actions to mitigate them.
8. Collaboration and Public Engagement
Promoting fairness in AI systems requires collaboration and engagement with various stakeholders, including policymakers, regulators, advocacy groups, and the general public. By involving these stakeholders, organizations can gain insights and perspectives on potential biases and ensure that AI systems are developed and deployed in a manner that aligns with societal values and expectations.
Despite the progress made in addressing data bias and promoting fairness in AI, challenges still remain. Achieving fairness requires an ongoing commitment from organizations, researchers, and policymakers to continuously evaluate and improve AI systems. Additionally, it is crucial to consider the intersectionality of various biases, as individuals may experience multiple forms of discrimination simultaneously.
By actively working towards reducing data bias and enhancing fairness in AI, we can ensure that AI systems are deployed responsibly and create positive impacts across diverse sectors of society. It is through such efforts that we can fully leverage the potential of AI and knowledge management while minimizing the risks of perpetuating bias and inequality.
### 10.4 Enhancing Human-AI Collaboration in Knowledge Management
In the era of Artificial Intelligence (AI), the collaboration between humans and AI systems has become increasingly important in various domains, including knowledge management. As AI technologies continue to advance, they have the potential to revolutionize the way organizations acquire, store, retrieve, share, transfer, and apply knowledge. However, to fully leverage this potential, it is crucial to strike a balance between the capabilities of AI and the unique skills and insights that humans bring to the table. This section explores the concept of enhancing human-AI collaboration in knowledge management and discusses the benefits and challenges associated with this collaboration.
The Power of Human-AI Collaboration
AI systems are adept at processing massive amounts of data, identifying patterns, and generating insights at a scale and speed beyond human capabilities. They can analyze complex information, extract knowledge, and provide recommendations based on the analysis. However, AI systems often lack the contextual understanding, creativity, empathy, and moral reasoning that humans possess. By combining the strengths of both humans and AI, organizations can achieve greater effectiveness and efficiency in knowledge management.
1. Complementary Abilities
Humans possess unique cognitive abilities such as critical thinking, intuition, and the ability to make moral judgments. These skills are vital for decision-making and problem-solving in knowledge management. AI systems, on the other hand, excel at processing large volumes of structured and unstructured data, detecting patterns, and automating routine tasks. By working together, humans and AI can complement each other's strengths, leading to more accurate and comprehensive knowledge management processes.
2. AI as a Knowledge Assistant
AI can act as a knowledge assistant, augmenting human decision-making processes by providing timely and relevant information. For example, AI-powered chatbots can assist employees in finding the right knowledge resources, answering common queries, and guiding them through a knowledge repository. This reduces the time and effort required for knowledge retrieval, allowing employees to focus on higher-value tasks.
3. Intelligent Knowledge Discovery
With the help of AI, human users can benefit from advanced knowledge discovery techniques. AI systems can analyze large datasets and identify valuable insights, trends, and correlations that humans may not have the capacity to discover manually. By leveraging AI algorithms, knowledge management professionals can uncover hidden patterns and relationships within the knowledge repository, leading to more informed decision-making and the discovery of new knowledge.
4. Collaborative Filtering and Recommendation Systems
AI-based recommendation systems can play a significant role in knowledge management by suggesting relevant knowledge resources based on individual preferences, expertise, and past interactions. These systems employ techniques such as collaborative filtering, content-based filtering, and hybrid approaches to curate personalized recommendations, enhancing the knowledge-seeking experience for users. By leveraging AI-driven recommendations, organizations can increase knowledge-sharing and collaboration among employees, ensuring the right knowledge reaches the right people at the right time.
Challenges in Human-AI Collaboration
While the collaboration between humans and AI systems in knowledge management holds immense potential, it is not without challenges. Addressing these challenges is crucial to ensuring effective collaboration and maximizing the benefits derived from AI technologies.
1. Trust and Acceptance
Building trust between humans and AI systems is essential for successful collaboration. Lack of trust can lead to resistance and reluctance in accepting AI-driven recommendations, resulting in underutilization of AI capabilities. Organizations must invest in building trust by ensuring transparency, explainability, and accountability in AI systems. Effective communication of the value AI brings to the knowledge management process is also crucial in gaining acceptance from human users.
2. Human-AI Interaction Design
Designing human-AI interfaces that are intuitive, user-friendly, and adaptive is critical for facilitating collaboration. AI systems should be designed to interact seamlessly with humans, providing explanations, clarifications, and feedback when necessary. The user experience should be personalized, taking into account user preferences and context. By focusing on intuitive design principles, organizations can ensure that humans can effectively work alongside AI systems without facing barriers or difficulties.
3. Ethical Considerations
Ethical considerations play a vital role in human-AI collaboration, especially in knowledge management. AI systems can inadvertently amplify biases present in the data or make decisions that are ethically questionable. Organizations must establish ethical guidelines for AI systems, ensuring fairness, transparency, and accountability in knowledge management practices. Human oversight and intervention should be in place to mitigate any potential ethical risks and ensure that AI systems serve the best interests of all stakeholders.
4. Continuous Learning and Adaptation
Both humans and AI systems need to continuously learn, adapt, and improve in the collaborative knowledge management process. Human experts must stay updated with the latest AI advancements and acquire the necessary skills to work effectively with AI. AI systems should be trained on the most recent data and continuously refined to enhance their performance and align with evolving human needs. Organizations need to invest in ongoing training and professional development programs to support the learning and collaboration between humans and AI.
The Future of Human-AI Collaboration in Knowledge Management
As AI technologies continue to evolve, the collaboration between humans and AI in knowledge management will become increasingly important. Future developments will focus on enhancing the symbiotic relationship between humans and AI, allowing for even deeper and more meaningful collaboration. This includes advancements in natural language processing, sentiment analysis, cognitive computing, and explainable AI, which will further enhance the capabilities of AI systems in supporting knowledge management processes.
Furthermore, the emergence of AI-powered virtual assistants and augmented reality technologies will create new opportunities for human-AI collaboration. Virtual assistants can provide real-time support, context-aware recommendations, and collaborative tools to enhance knowledge sharing and collaboration among distributed teams. Augmented reality interfaces can overlay AI-driven insights onto real-world scenarios, enabling humans to make more informed decisions based on valuable knowledge extracted by AI systems.
In conclusion, enhancing human-AI collaboration in knowledge management is crucial for leveraging the full potential of AI in the era of Artificial Intelligence. By combining the complementary abilities of humans and AI, organizations can achieve more effective and efficient knowledge management processes. However, addressing challenges related to trust, human-AI interaction design, ethical considerations, and continuous learning is essential for successful collaboration. Looking ahead, future advancements in AI technologies hold tremendous promise for further enhancing human-AI collaboration and revolutionizing knowledge management practices.
### 10.5 Emerging Trends and Future Directions
As the field of Artificial Intelligence (AI) continues to evolve, new trends and directions are emerging in the realm of fractals and knowledge management. These trends have the potential to shape the future of knowledge organization, discovery, and application in the AI era. In this section, we will explore some of these emerging trends and their implications for the field.
10.5.1 Fractal-Based AI Algorithms
One of the emerging trends in AI is the development and application of fractal-based algorithms. Fractal geometry offers a unique approach to data representation and analysis, which can enhance the performance of AI systems. By leveraging the self-similarity and complexity inherent in fractal patterns, these algorithms can capture intricate relationships and patterns in large datasets.
Fractal-based AI algorithms have shown promise in various domains, including image and speech recognition, anomaly detection, and predictive analytics. These algorithms enable AI systems to extract finer-grained features from complex data, leading to more accurate and robust models. As researchers continue to explore the potential of fractal-based AI algorithms, we can expect to see further advancements in data analysis and pattern recognition.
10.5.2 Explainable AI and Interpretability
As AI becomes increasingly integrated into various aspects of our lives, there is a growing need for transparency and interpretability. AI systems are often seen as "black boxes," making it difficult to understand how they arrive at their decisions or recommendations. This lack of transparency poses challenges, particularly in critical domains such as healthcare, finance, and law.
Emerging trends in AI focus on developing explainable AI models that can provide insights into the decision-making process of AI systems. Fractal patterns can play a crucial role in this endeavor. Fractals offer a visual and intuitive means of representing complex data structures, allowing users to interpret and understand AI system outputs more effectively.
By leveraging fractal visualization techniques, researchers can provide explanations for the decisions made by AI models. Moreover, fractal-based AI algorithms enable the identification of patterns and features that influence the decision-making process, enhancing the interpretability of AI systems. As the demand for explainable AI grows, incorporating fractal-based methods can help build trust and acceptance of AI technologies.
10.5.3 Human-AI Collaboration in Knowledge Management
The future of knowledge management in the AI era lies in the seamless integration of human and AI capabilities. While AI systems are capable of processing vast amounts of data and making intelligent decisions, human expertise and intuition remain valuable assets in knowledge creation and application.
Emerging trends in knowledge management focus on harnessing the complementary strengths of humans and AI. Fractal-based knowledge management systems allow for human-AI collaboration by providing intuitive interfaces for data visualization and analysis. These systems enable users to navigate through complex datasets and discover hidden patterns with ease.
Moreover, AI-powered recommendation systems can assist knowledge workers in finding relevant information and expertise, enhancing productivity and decision-making. By combining the power of AI algorithms with human insights, organizations can unlock the full potential of their knowledge assets and drive innovation.
10.5.4 Ethical Considerations and Bias Mitigation
As AI systems become progressively embedded in our daily lives, addressing ethical considerations and mitigating bias becomes paramount. The integration of fractal patterns in AI offers opportunities for addressing these challenges.
Fractals exhibit self-similarity and complexity, providing a contrast to biased and oversimplified representations often encountered in AI systems. By incorporating fractal-based approaches, AI models can capture diverse perspectives and complex relationships, reducing the risk of perpetuating biases.
Additionally, fractal visualization techniques allow for the identification and analysis of bias in datasets and AI algorithms. This enables researchers and developers to take proactive steps in minimizing and eliminating biases, promoting fairness, and ensuring equitable outcomes.
10.5.5 Enhancing AI-Augmented Decision Making
In the future, AI will play an integral role in decision-making processes across various domains. Whether it is in healthcare, finance, or strategic planning, AI algorithms can support human decision-makers by providing valuable insights and recommendations.
Emerging trends focus on enhancing the effectiveness of AI-augmented decision-making by incorporating fractal-based methods. Fractal patterns offer a holistic representation of data, capturing intricate relationships and dependencies. By integrating these patterns into decision-making algorithms, AI systems can provide more accurate and informed recommendations.
Furthermore, fractal-based AI algorithms can enable decision-makers to consider multiple possibilities and scenarios, facilitating risk assessment and scenario analysis. This can drive better strategic decisions and mitigate potential risks.
In conclusion, the emerging trends and future directions in fractals and knowledge management in the AI era hold significant promise for the field. Fractal-based AI algorithms, explainable AI, human-AI collaboration, ethical considerations, and enhancing AI-augmented decision-making are all areas that will shape the future of AI and knowledge management. By combining the power of fractals with AI technologies, organizations can unlock new levels of understanding, discovery, and innovation, ultimately leading to more effective and responsible use of AI in knowledge management.
## 11. Case Studies in Fractal-Based Knowledge Management
### 11.1 Fractal-Based Robotics and AI
The integration of fractals and knowledge management in the field of robotics and artificial intelligence (AI) has significantly transformed the way we perceive and interact with technology. With the ability to mimic the intricate patterns found in nature, fractals have opened new avenues for innovation and improvement in robotics and AI systems. This section explores the applications, benefits, and challenges associated with fractal-based robotics and AI.
Understanding Fractals in Robotics
Fractals, known for their self-similarity and complex geometric patterns, serve as a valuable tool in robotics. By utilizing fractal principles, robotic systems can mimic the behavior and structure of natural organisms more accurately. This enables robots to perform complex tasks efficiently and adapt to dynamic environments effectively.
One of the prominent applications of fractals in robotics is in designing robotic locomotion systems. By imitating the movement patterns found in nature, such as the motions of animals or the growth of plants, robotic engineers can create more efficient and adaptable locomotion mechanisms. The fractal-based approach allows robots to navigate uneven terrains, maneuver through obstacles, and optimize energy consumption, making them more versatile and capable of performing tasks in a variety of environments.
Enhancing AI in Robotics with Fractals
Incorporating fractal principles in AI algorithms has revolutionized the field of robotics. Fractal-based AI algorithms can improve robotic decision-making processes, enhance perception capabilities, and optimize task execution. The self-similarity and scalability of fractals enable AI systems to recognize patterns, learn from experience, and generate adaptive responses based on environmental changes.
Fractal-based AI algorithms excel in tasks such as object recognition, path planning, and decision-making. By leveraging the power of fractals, AI algorithms can identify complex patterns and extract meaningful information from the environment. This enables robots to make accurate decisions in real-time while adapting to changing conditions. For example, a fractal-based AI algorithm can help a robotic device in a warehouse efficiently navigate through a cluttered environment, optimizing its path to minimize time and energy consumption.
Benefits of Fractal-Based Robotics and AI
The integration of fractals in robotics and AI offers several benefits, ranging from improved performance to increased efficiency. Some key advantages include:
1. Adaptability and Robustness: Fractal-based robotic systems possess adaptability and robustness similar to those found in natural organisms. These systems can respond effectively to changing environments and overcome obstacles, leading to increased operational efficiency and improved outcomes.
2. Energy Efficiency: Fractal-based robots optimize energy consumption by mimicking natural growth patterns and minimizing unnecessary movements. This energy-efficient approach reduces both costs and environmental impact.
3. Enhanced Perception: By incorporating fractal-based algorithms in AI systems, robotic devices can enhance their perception capabilities. They can identify and interpret complex patterns and make informed decisions accordingly, leading to improved performance and more reliable outcomes.
4. Increased Autonomy: Fractal-based robotics and AI empower robots to act autonomously in dynamic and unstructured environments. The ability to learn and adapt from experience enables robotic systems to operate independently, reducing the need for constant human intervention.
Challenges and Considerations
Despite the significant potential, fractal-based robotics and AI also face certain challenges and considerations that need to be addressed for their successful implementation:
1. Complexity and Computational Cost: Implementing fractal-based algorithms in robotics and AI systems often involves complex mathematical models and high computational costs. This can pose challenges in terms of real-time decision-making and resource limitations.
2. Design and Engineering: Designing and engineering fractal-based robotic systems require interdisciplinary knowledge and expertise. It requires collaboration between mathematicians, robotic engineers, and AI specialists to create efficient and reliable systems.
3. Ethical Implications: As robotics and AI systems become more sophisticated, ethical considerations also arise. Ensuring proper regulations and guidelines to prevent misuse and address the concerns related to privacy, security, and bias becomes crucial.
4. Scalability and Generalization: While fractals offer scalability at various levels, ensuring the generalizability of fractal-based robotics and AI approaches remains a challenge. Achieving robust performance across different tasks and environments requires careful optimization and thorough testing.
Future Directions
The integration of fractals in robotics and AI is still in its early stages, and there are ample opportunities for future exploration and advancement. Some potential directions for further research and development include:
1. Bio-inspired Robotics: Fractals can serve as a valuable source of inspiration for the design and development of bio-inspired robotic systems. By studying and imitating the growth patterns and behaviors of living organisms, researchers can create more adaptable, efficient, and autonomous robots.
2. Human-Robot Collaboration: Fractal-based robotics and AI can enhance human-robot collaboration by enabling seamless interaction and communication. This can lead to the development of robotic systems that augment human capabilities and work alongside humans in various domains.
3. Multi-Robot Systems: Fractal-based approaches can facilitate coordination and collaboration among multiple robots, leading to the development of sophisticated swarm robotics systems. These systems can leverage fractal principles to achieve collective intelligence, adaptability, and scalability.
4. Fractal-Based Perception: Further research can explore the application of fractal geometry in improving perception capabilities of robotic systems. By utilizing fractals to analyze and interpret sensory data, robots can achieve more sophisticated and human-like perception.
In conclusion, the integration of fractals and knowledge management in the field of robotics and AI brings numerous opportunities and benefits. Fractal-based approaches enhance the adaptability, decision-making, and perception capabilities of robotic systems, resulting in improved performance, energy efficiency, and autonomy. Despite the challenges and considerations, the future looks promising, with potential advancements in bio-inspired robotics, human-robot collaboration, multi-robot systems, and perception capabilities. As research progresses, the field of fractal-based robotics and AI holds great promise in revolutionizing various industries and shaping the future of technology.
### 11.2 Fractal Analysis in Medical Knowledge Management
In the era of Artificial Intelligence (AI), the integration of fractal analysis with knowledge management has opened up new possibilities in various fields, including healthcare. Medical knowledge management involves the acquisition, storage, retrieval, sharing, and application of healthcare-related information. By incorporating fractal analysis into this process, healthcare organizations can gain deeper insights into complex medical data, leading to improved patient care, diagnosis, and treatment.
Fractal analysis is the study of patterns that repeat themselves at different scales or levels of detail. Fractals are self-similar structures that exhibit intricate and complex patterns regardless of the level of magnification. This characteristic makes fractals particularly useful in medical knowledge management, as medical data contains multilevel and multiscale information.
One of the key applications of fractal analysis in medical knowledge management is medical image analysis. Medical images such as X-rays, CT scans, MRI scans, and ultrasound images contain rich information that can be challenging to interpret and analyze. Fractal analysis offers a unique approach to analyzing these images by quantifying the complexity and self-similarity of the structures within the image.
By applying fractal analysis to medical images, healthcare professionals can extract valuable information that is often not visible to the naked eye. For example, fractal analysis can help identify subtle changes in tissue structures that may be indicative of abnormalities or diseases. It can also aid in the characterization of tumor growth patterns, the assessment of tissue texture, and the detection of microcalcifications in mammograms. These insights can significantly enhance the accuracy of medical diagnoses and improve patient outcomes.
Fractal analysis is also valuable in the field of medical signal processing. Signals such as electrocardiograms (ECGs), electroencephalograms (EEGs), and respiratory waveforms exhibit complex patterns that can vary across different scales. Fractal analysis of these signals can provide useful information about the underlying physiological processes and help in the diagnosis of various medical conditions.
For instance, in the analysis of ECG signals, fractal analysis can help detect abnormalities in heart rate variability and identify irregular patterns that may indicate cardiac arrhythmias. In the case of EEG signals, fractal analysis can be used to study brain wave patterns and detect anomalies associated with epilepsy, Alzheimer's disease, and other neurological disorders. By incorporating fractal analysis into medical signal processing, healthcare practitioners can gain valuable insights into the underlying mechanisms of various diseases, leading to more accurate diagnoses and personalized treatment options.
In addition to medical image and signal analysis, fractal analysis has proven to be beneficial in the field of genomics and molecular biology. The analysis of genomic data, such as DNA sequences and protein structures, often requires sophisticated mathematical and computational techniques. Fractal analysis can provide a unique perspective on the complexity and self-similarity present within these biological datasets, offering insights into the organization and functionality of genes and proteins.
By applying fractal analysis to genomic data, researchers can identify hidden patterns and structures that may be critical for understanding genetic mutations, protein folding, and the development of diseases. This knowledge can be invaluable in the design of targeted therapies and the development of personalized medicine.
Moreover, the integration of fractal analysis with electronic health records (EHRs) can enhance medical knowledge management by enabling the extraction of meaningful insights from large-scale patient data. Fractal-based analysis techniques can help identify patterns and correlations within EHRs, leading to improved clinical decision-making, disease surveillance, and public health management.
For example, by analyzing the temporal patterns of patient vital signs, such as heart rate and blood pressure, in EHRs, healthcare providers can detect early warning signs of deterioration and take preventive measures accordingly. Fractal analysis can also aid in the identification of disease outbreaks by analyzing patterns of disease incidence and transmission within large populations.
In summary, the application of fractal analysis in medical knowledge management has the potential to revolutionize healthcare. By leveraging the intricate patterns and self-similarity inherent in medical data, healthcare professionals can gain deeper insights into complex phenomena, leading to more accurate diagnoses, improved treatment plans, and better patient outcomes. The integration of fractal analysis with AI-driven knowledge management platforms holds tremendous promise for the future of healthcare, paving the way for smarter and more efficient medical decision-making.
### 11.3 Fractals and Financial Knowledge Management
Financial institutions generate vast amounts of data on a daily basis. These data streams include market data, customer information, transaction records, and more. Managing and extracting insights from this data is crucial for financial institutions to stay competitive and make informed decisions. With the advent of artificial intelligence (AI), financial knowledge management has been revolutionized, and fractal patterns play a significant role in this domain.
Fractals, with their self-similarity and intricate patterns, can be employed to extract meaningful information from complex financial datasets. Financial markets exhibit fractal properties, meaning that similar patterns can be observed at different scales within market data. These fractal patterns reflect market behaviors, such as price movements, volatility trends, and the relationships between different financial instruments.
One of the areas where fractals have proven to be invaluable is in financial forecasting and prediction. Traditional statistical models often struggle to capture complex non-linear relationships in financial data, whereas fractal analysis provides a more suitable approach. Fractals allow us to identify hidden patterns within the data, facilitating the accurate prediction of market trends and asset prices.
Fractal analysis techniques, such as the Hurst exponent, can measure the long-term memory or persistence of a financial time series. This metric helps in assessing market efficiency and identifying mean-reverting or trending behaviors. By analyzing the fractal nature of financial time series, financial knowledge management systems can better understand market dynamics and make more accurate predictions.
Another application of fractals in financial knowledge management is risk assessment and management. Fractals can capture the inherent complexity and volatility of financial markets, providing insights into potential risks and their magnitudes. By analyzing fractal patterns in market data, financial institutions can identify systemic risks, predict market crashes, and develop robust risk management strategies.
Fractals also have implications for portfolio optimization. Traditional techniques, such as modern portfolio theory, often assume linear relationships between asset returns. However, fractal analysis allows for the consideration of non-linear dependencies and the impact of global market behaviors. Incorporating fractal-based measures into portfolio management strategies can lead to more diversified and resilient portfolios, reducing exposure to systematic risks.
Furthermore, fractal analysis can assist in market microstructure analysis, which focuses on understanding the dynamics of order flow, trading volumes, and bid-ask spreads. Fractals enable the identification of hidden patterns in high-frequency trading data, providing insights into market liquidity, price impact, and trading strategies. By leveraging fractal patterns, financial knowledge management systems can optimize trading algorithms and enhance execution strategies.
Fractals also play a vital role in sentiment analysis and social media analytics in the financial domain. Sentiment analysis aims to gauge public opinion and emotional responses in real-time. By analyzing social media data using fractal techniques, financial institutions can gain insights into market sentiment, identify emerging trends, and anticipate changes in market sentiment that may affect trading decisions.
Moreover, the application of fractals in financial knowledge management extends beyond analyzing market data. Fractal-based techniques can also improve fraud detection and money laundering prevention. Fractal patterns can uncover deviations and anomalies within financial transaction datasets, helping identify suspicious activities and patterns associated with fraudulent behavior.
In summary, the incorporation of fractal analysis into financial knowledge management in the AI era offers numerous benefits for financial institutions. By leveraging the self-similarity and complex patterns found in financial data, fractals enable accurate prediction of market trends, better risk management, enhanced portfolio optimization strategies, and improved sentiment analysis. Fractal-based approaches also contribute to market microstructure analysis, fraud detection, and money laundering prevention. As the field of AI continues to evolve, fractal-based knowledge management systems will undoubtedly play a more prominent role in the financial sector, providing institutions with a competitive edge and facilitating informed decision-making.
### 11.4 Fractal Patterns in Social Media Analytics
Social media has become an integral part of our lives, providing a vast sea of data that contains valuable insights into human behavior, preferences, and trends. With the advent of Artificial Intelligence (AI) and knowledge management techniques, social media analytics has taken a leap forward in capturing, analyzing, and deriving actionable intelligence from this data.
In the era of AI, understanding the complex dynamics of social media requires innovative approaches that can handle the massive volume, variety, and velocity of data generated by these platforms. Fractal patterns offer a promising framework for capturing and analyzing the underlying structure and behavior of users on social media.
Fractals, with their self-similar and recursive nature, can reveal patterns at different scales, uncovering hidden relationships, and providing a deeper understanding of complex systems. When applied to social media analytics, fractal patterns can help in various areas, such as sentiment analysis, trend detection, user segmentation, influence mapping, and recommendation systems.
Applying Fractals for Sentiment Analysis
Sentiment analysis, also known as opinion mining, is a vital component of social media analytics. It involves identifying, extracting, and classifying subjective information from user-generated content to determine the sentiment or opinion expressed. By applying fractal analysis to sentiment data, it is possible to identify patterns in the emotional dynamics of users.
Fractal analysis can reveal the recurring patterns in sentiment shifts over time, highlighting the cyclic nature of emotional fluctuations on social media. This understanding can help businesses and marketers to craft appropriate responses or interventions to mitigate negative sentiment or leverage positive sentiment.
Detecting Trends with Fractal Patterns
Trend detection is essential for businesses to stay ahead of the curve and anticipate market demands. By analyzing social media data with fractal techniques, it becomes possible to identify emerging trends and predict their future trajectory with higher accuracy.
Fractal patterns can capture the self-similarity and repetition in social media data, allowing analysts to detect patterns at different scales. These patterns can then be used to predict the growth, decline, or saturation of trends, enabling organizations to make timely decisions and take advantage of new opportunities.
User Segmentation and Influence Mapping
Understanding the demographics, interests, and preferences of social media users is crucial for targeted marketing, personalized recommendations, and identifying influencers. Fractal analysis can play a significant role in user segmentation and influence mapping by uncovering hidden patterns and relationships within social media networks.
By analyzing the fractal structure of connections and interactions between users, it becomes possible to identify clusters of users with similar characteristics or interests. This information can be used to personalize marketing campaigns, tailor content, and identify potential brand advocates or influencers who can amplify a company's message to a wider audience.
Fractal-Based Recommendation Systems
Recommendation systems have become an integral part of social media platforms, providing users with personalized content, products, and services. Leveraging fractal patterns in recommendation systems can enhance their effectiveness and accuracy.
Fractal analysis can identify similarities and dissimilarities among users, considering not just their explicit preferences but also the underlying patterns and dynamics of their behavior. By incorporating fractal-based recommendations, social media platforms can provide more relevant and engaging content, leading to increased user satisfaction and loyalty.
The Power of Fractal Patterns in Social Media Analytics
Fractal patterns provide a powerful framework for extracting meaningful insights from social media data. They reveal intricate structures, dynamics, and relationships that are not immediately apparent.
By understanding and leveraging fractal patterns in social media analytics, organizations can gain a competitive edge in areas such as sentiment analysis, trend detection, user segmentation, influence mapping, and recommendation systems. These insights can help businesses make data-driven decisions, enhance customer experiences, and drive innovation.
However, it is essential to recognize that applying fractal analysis to social media data requires advanced technologies and expertise in AI and knowledge management. Robust data collection, preprocessing, and analysis pipelines, combined with scalable algorithms and computing infrastructure, are necessary to handle the enormous volume and complexity of social media data.
Moreover, ethical considerations and privacy concerns must be carefully addressed when using social media data for analytics. Respecting user privacy and ensuring data security are crucial to build trust and maintain the integrity of social media analytics.
In conclusion, by harnessing the power of fractal patterns, social media analytics can unlock valuable insights and actionable intelligence. The combination of AI techniques and knowledge management practices allows organizations to navigate the vast social media landscape, understand their customers better, and make informed decisions. Fractal-based social media analytics opens up new opportunities for businesses to thrive and succeed in the AI era.
### 11.5 Fractal-Based Knowledge Management in Manufacturing
In the era of Artificial Intelligence (AI), the application of knowledge management in various industries has become crucial for organizations to stay competitive and drive innovation. One such industry where knowledge management can play a significant role is manufacturing. With the adoption of AI and the integration of fractal-based approaches, knowledge management in manufacturing can be revolutionized, leading to enhanced productivity, increased efficiency, and improved decision-making.
The Role of Fractals in Knowledge Management
Fractals, with their self-similar patterns that repeat at different scales, offer a unique way to represent complex and interconnected information in a manufacturing environment. In the context of knowledge management, fractals can be applied to capture and organize vast amounts of data, creating a structured knowledge repository. This repository can then be utilized by AI algorithms to derive insights and make informed decisions, ultimately optimizing various manufacturing processes.
Knowledge Creation and Acquisition
One of the fundamental aspects of knowledge management in manufacturing is the creation and acquisition of knowledge. Fractal-based approaches can assist in capturing knowledge from different sources, including experts, research papers, technical documents, and operational data. By applying fractal algorithms, relevant information can be extracted and transformed into a cohesive knowledge base, allowing organizations to leverage this knowledge for continuous process improvement.
For example, in a manufacturing facility, production data, maintenance records, and quality assurance reports can be transformed into a fractal-based knowledge representation. This representation can capture the interdependencies between various factors, such as machine settings, raw material characteristics, and environmental conditions. By analyzing these fractal patterns, organizations can identify critical factors influencing product quality, detect potential bottlenecks in the production line, and develop strategies for optimization.
Knowledge Storage and Retrieval
Fractal-based knowledge management systems can provide efficient storage and retrieval mechanisms for manufacturing organizations. By utilizing fractal compression techniques, large datasets can be compressed and stored in a compact form, reducing storage requirements. Furthermore, the self-similarity property of fractals enables fast and accurate retrieval of information based on similarity metrics.
In the manufacturing context, this means that historical data, production plans, and other relevant information can be stored in a compressed manner, saving storage space while retaining critical details. When retrieving knowledge from this repository, AI algorithms can employ fractal-based similarity measures to recommend relevant information based on patterns and similarities, enabling manufacturers to access the necessary knowledge quickly and efficiently.
Knowledge Sharing and Collaboration
Knowledge sharing and collaboration are essential for fostering innovation and continuous improvement in manufacturing organizations. Fractal-based knowledge management systems can facilitate effective knowledge sharing by providing a structured and intuitive representation of knowledge. This representation allows users to navigate through complex information and identify relationships and connections effortlessly.
Manufacturing teams can collaborate on various projects by leveraging the knowledge repository and sharing their insights and experiences. Fractal-based visualization techniques can help teams understand complex concepts and patterns, leading to more effective decision-making and problem-solving. By combining AI algorithms with fractal-based knowledge management, manufacturers can promote cross-functional collaboration and leverage the collective intelligence of their workforce.
Knowledge Transfer and Application
The transfer and application of knowledge are vital for improving manufacturing processes and driving innovation. Fractal-based knowledge management can support knowledge transfer by organizing and codifying knowledge into reusable formats. Manufacturing organizations can leverage this organized knowledge to train AI systems, enabling them to learn from historical data, identify patterns, and make accurate predictions.
For instance, by analyzing historical production data using fractal-based techniques, AI algorithms can learn from past experiences and identify optimal settings and conditions for improved productivity. This knowledge can be transferred to new manufacturing lines or employees, ensuring that best practices and lessons learned are effectively utilized across the organization.
Benefits and Challenges
The application of fractal-based knowledge management in manufacturing offers several significant benefits. It enables organizations to capture, store, and access knowledge efficiently, leading to improved decision-making, enhanced process optimization, and increased innovation. By leveraging fractal-based visualization techniques, organizations can gain deeper insights into complex manufacturing processes, allowing for better identification and resolution of issues.
However, there are also challenges that need to be addressed when implementing fractal-based knowledge management in manufacturing. These challenges include the need for adequate data quality and integrity, the interpretation of complex fractal patterns, and the training of AI systems using fractal-encoded knowledge. Overcoming these challenges requires a multidisciplinary approach, involving experts in AI, knowledge management, and manufacturing domain knowledge.
In conclusion, the integration of fractal-based knowledge management in manufacturing holds tremendous potential for driving efficiency, innovation, and optimization. By leveraging fractal patterns, AI algorithms can extract and analyze knowledge from vast amounts of data, enabling organizations to make informed decisions and drive continuous improvement. With the proper implementation and addressing of associated challenges, manufacturers can unlock the full benefits of fractal-based knowledge management and propel their organizations into the future of AI-powered manufacturing.
## 12. Conclusion
### 12.1 Key Takeaways
In this chapter, we have explored the integration of fractals and knowledge management in the era of Artificial Intelligence (AI). Fractals, with their infinite complexity and self-similarity, have proven to be valuable tools in understanding and representing complex systems. Knowledge management, on the other hand, focuses on capturing, organizing, sharing, and applying knowledge within organizations. By combining these two fields, we can unlock the true potential of AI in managing knowledge and enhancing decision-making processes.
Here are the key takeaways from our exploration of fractals and knowledge management in the AI era:
1. Fractals Provide a Framework for Understanding Complex Systems
Fractals offer a powerful framework for understanding and modeling complex systems. Their self-similarity and infinite detail make them appropriate for representing intricate patterns found in many natural and artificial phenomena. By leveraging fractal geometry, researchers and practitioners can gain deeper insights into the underlying structure and dynamics of complex systems, including those encountered in AI algorithms and applications.
2. Knowledge Management Enhances AI Systems
Knowledge management plays a vital role in supporting AI systems by ensuring that relevant information is captured, organized, and made available for decision-making and learning processes. Effective knowledge management facilitates the creation, acquisition, storage, retrieval, sharing, collaboration, transfer, and application of knowledge across individuals, teams, and organizations. By integrating knowledge management principles and practices into AI systems, we can enhance their capabilities and improve their performance.
3. Fractals and Knowledge Management Synergize in AI
The integration of fractals and knowledge management in the AI era provides numerous opportunities for synergistic collaboration. Fractals can be leveraged in knowledge management processes to model and represent the intricacies and patterns found in knowledge structures, relationships, and dynamics. Furthermore, AI-driven techniques and algorithms can be used to uncover hidden patterns, extract meaningful insights, and make predictions based on the fractal nature of knowledge.
4. AI-Driven Knowledge Discovery Unlocks Insights
AI algorithms, such as machine learning and deep learning, can be applied to knowledge management processes to discover hidden patterns, trends, and relationships within large and complex knowledge repositories. By leveraging the fractal patterns inherent in knowledge, AI-driven knowledge discovery techniques can provide valuable insights that may not be apparent through traditional approaches. These insights can enable organizations to make better-informed decisions and drive innovation.
5. AI-Enabled Knowledge Capture and Organization Enhance Efficiency
With the help of AI, knowledge can be captured and organized more efficiently. Natural Language Processing (NLP) techniques can convert unstructured data, such as text documents, into structured and searchable knowledge. AI algorithms can automatically tag, categorize, and classify knowledge based on content, context, and user feedback. By integrating AI-enabled knowledge capture and organization techniques, organizations can improve the accessibility and usability of their knowledge repositories.
6. AI-Powered Knowledge Sharing and Collaboration Foster Innovation
AI-driven knowledge sharing and collaboration platforms empower individuals and teams to share and exchange knowledge more effectively. AI algorithms can recommend relevant knowledge to users based on their interests, expertise, and context. Collaborative filtering techniques can facilitate the discovery of experts and foster meaningful interactions. By harnessing the power of AI in knowledge sharing and collaboration, organizations can foster innovation, enhance problem-solving capabilities, and drive continuous learning.
7. AI-Supported Knowledge Transfer and Application Accelerate Learning
AI can accelerate the transfer and application of knowledge within organizations. By leveraging AI algorithms, organizations can automate the extraction of actionable knowledge from vast amounts of data. Machine learning models can be used to predict and recommend optimal actions based on historical data and knowledge. This accelerates the learning process and enables organizations to make informed decisions in a timely manner.
8. Ethical Considerations Shape AI-Driven Knowledge Management
As with any application of AI, ethical considerations are crucial in the context of knowledge management. AI algorithms must be designed and implemented with fairness, transparency, and accountability in mind. Bias in data and algorithms should be identified and mitigated to ensure equitable and unbiased access to knowledge. Organizations must also consider privacy and security concerns when implementing AI-driven knowledge management systems.
In conclusion, the integration of fractals and knowledge management in the era of AI opens up new possibilities for managing knowledge and enhancing decision-making processes. Fractals provide a powerful framework for understanding complex systems, while knowledge management principles and practices facilitate the capture, organization, sharing, and application of knowledge. By leveraging AI technology, organizations can unlock valuable insights, enhance efficiency, foster innovation, accelerate learning, and address ethical considerations in knowledge management. The future of AI and knowledge management holds immense potential for transforming organizations and society at large. It is imperative for organizations to embrace this synergy and harness the power of fractals and AI in knowledge management to stay ahead in the AI-driven world.
### 12.2 Implications of Fractal-Based Knowledge Management
Fractal-based knowledge management holds immense potential in the era of Artificial Intelligence (AI). By combining the principles of fractal geometry and the power of AI technologies, organizations can achieve more efficient and effective knowledge management practices. In this section, we will explore the implications of employing fractal-based knowledge management in the AI era.
Enhancing Knowledge Capture and Organization
One of the key implications of fractal-based knowledge management in the AI era is its ability to enhance knowledge capture and organization processes. Fractals, with their self-similar patterns at different scales, provide a unique framework for capturing complex and interconnected knowledge. AI algorithms can be employed to analyze and classify this knowledge, enabling organizations to organize and structure their knowledge repositories efficiently. By leveraging fractal-based approaches, organizations can ensure that knowledge is captured comprehensively and organized in a manner that preserves its intricate relationships.
Facilitating Knowledge Discovery and Exploration
Fractal-based knowledge management also facilitates knowledge discovery and exploration in the AI era. Fractals inherently possess fractal dimensions, allowing for the embedding of vast amounts of information within a reduced space. This property can be leveraged to create knowledge graphs or semantic networks, which provide a visual representation of the complex relationships between different knowledge elements. AI algorithms can then be utilized to analyze these structures and uncover hidden patterns, enhancing the process of knowledge exploration and enabling novel discoveries. By leveraging fractal-based techniques, organizations can gain deeper insights into their knowledge repositories, uncovering valuable information that may have been overlooked using traditional knowledge management approaches.
Enabling Adaptive and Self-Organizing Knowledge Systems
Fractal-based knowledge management in the AI era also enables the creation of adaptive and self-organizing knowledge systems. Fractals exhibit self-similarity and self-organization, which can be harnessed to create dynamic and adaptive knowledge structures. AI technologies can be employed to continuously analyze and update these structures, ensuring that knowledge representations remain relevant and up-to-date. With fractal-based knowledge management, organizations can create intelligent systems that automatically adapt to changes in the knowledge landscape, enabling more effective decision-making and problem-solving.
Supporting Knowledge Transfer and Application
Effective knowledge transfer and application are crucial for organizations to fully leverage their intellectual capital. Fractal-based knowledge management in the AI era offers innovative solutions to support these processes. Fractal patterns can be used to identify similarities between different domains or areas of knowledge, facilitating cross-disciplinary knowledge transfer. AI-driven recommendation systems can utilize fractal-based algorithms to match the knowledge needs of individuals with appropriate knowledge sources, enhancing knowledge application. By leveraging these techniques, organizations can foster a culture of continuous learning and knowledge sharing, driving innovation and improving organizational performance.
Optimizing Knowledge Integration and Collaboration
Fractal-based knowledge management also optimizes knowledge integration and collaboration in the AI era. Fractal patterns can be used to identify potential areas of knowledge integration, where different domains or disciplines converge. By understanding these areas of convergence, organizations can foster interdisciplinary collaboration and innovation. AI technologies can facilitate this collaboration by leveraging fractal-based techniques to identify suitable collaborators, based on their expertise and knowledge profiles. Fractal-based knowledge management, thus, enables organizations to harness the collective intelligence of their workforce, resulting in richer and more comprehensive knowledge integration.
Overcoming Information Overload and Extracting Insights
In the AI era, organizations are inundated with vast amounts of information. Fractal-based knowledge management techniques offer a way to overcome information overload and extract valuable insights from this abundance of data. Fractals enable the compression and representation of complex information in a compact and meaningful manner. AI algorithms can then be employed to analyze these representations, identifying relevant patterns and extracting actionable insights. By leveraging fractal-based knowledge management, organizations can make sense of the overwhelming data landscape, enabling more informed decision-making and strategic planning.
Improving AI Performance and Robustness
Fractal-based knowledge management also has implications for improving the performance and robustness of AI systems. Fractal patterns, with their self-similarity and self-organization properties, can be used to create intelligent algorithms that exhibit similar characteristics. By incorporating fractal-inspired principles into AI algorithms, organizations can develop more robust and scalable systems that can adapt to new challenges and environments. This can enhance the performance of AI models, enabling them to handle complex and unpredictable scenarios more effectively.
### 12.3 The Future of AI and Knowledge Management
As we delve into the future of AI and knowledge management, it is evident that the integration of these two domains holds immense potential for transformative advancements. The AI era is marked by the exponential growth of data and the increasing need for efficient knowledge management strategies. In this section, we will explore the future possibilities and implications of AI-driven knowledge management, highlighting the key trends and potential challenges that lie ahead.
AI-Powered Knowledge Discovery and Augmentation
In the future, AI will play a crucial role in enhancing the process of knowledge discovery. As organizations accumulate vast amounts of data, extracting valuable insights and knowledge becomes a significant challenge. AI-driven algorithms can be employed to analyze and extract patterns and trends from these large datasets, enabling organizations to uncover hidden knowledge and gain a competitive edge.
Moreover, AI has the potential to augment human intelligence by automating routine tasks involved in knowledge discovery. Machine learning algorithms can assist in mining information from disparate sources, identifying correlations, and providing valuable recommendations. This integration of AI and knowledge management will empower organizations to make more informed decisions and drive innovation.
Intelligent Knowledge Capture and Organization
AI techniques such as natural language processing and machine learning are enabling the development of intelligent knowledge capture and organization systems. These systems can automatically categorize and tag information, making it easier to retrieve and access relevant knowledge. Through advanced semantic technologies, AI can understand the meaning and context of textual data, enabling more accurate knowledge organization and retrieval.
In the future, we can expect the integration of AI in knowledge management systems to further enhance the capture and organization of tacit knowledge. With the help of deep learning algorithms, organizations can capture and leverage the expertise and experiences of their employees, ensuring that valuable knowledge is not lost when individuals leave the organization.
Collaborative Knowledge Sharing with AI
AI technologies will revolutionize knowledge sharing and collaboration, enabling seamless access to insights and expertise across organizational boundaries. Organizations can leverage AI-driven chatbots or virtual assistants to facilitate knowledge sharing among employees, enabling them to quickly access relevant information and expertise.
Furthermore, AI can assist in creating virtual collaboration spaces where employees from different locations can collaborate in real-time, leveraging their collective knowledge and expertise. Advanced natural language processing techniques can bridge the gap between different languages and enable effective communication and collaboration in a multilingual environment.
AI-Enhanced Knowledge Transfer and Application
The integration of AI in knowledge management will also revolutionize the process of knowledge transfer and application. AI-powered systems can help identify knowledge gaps within an organization, and recommend relevant training materials or experts to address those gaps. Through personalized learning algorithms, employees can receive tailored knowledge transfer experiences, maximizing their learning potential.
Moreover, AI algorithms can provide context-aware recommendations, suggesting relevant knowledge during the decision-making process. This integration of AI and knowledge management ensures that organizations can make informed decisions based on accurate and up-to-date information.
Ethical Considerations and Responsible AI
As AI becomes more prevalent in knowledge management, organizations must address the ethical considerations associated with its use. The responsible and ethical use of AI technologies must be prioritized to ensure fairness, transparency, and accountability. Bias in data, algorithms, and decision-making processes must be actively monitored and mitigated to avoid perpetuating societal biases or discrimination.
Additionally, privacy and security concerns must be addressed to protect sensitive knowledge and personal information. Organizations need to develop robust data governance frameworks and adhere to regulatory requirements to safeguard the confidentiality of knowledge assets.
Challenges and Future Directions
While the future of AI-driven knowledge management is promising, there are several challenges that must be addressed. The first is the need for continued investment in AI research and development to further enhance the capabilities of knowledge management systems. Advances in machine learning, natural language processing, and cognitive computing are essential to realizing the full potential of AI in knowledge management.
Furthermore, the complexity of integrating AI technologies with existing knowledge management systems and workflows poses a challenge. Organizations need to ensure seamless integration, user adoption, and training to maximize the benefits of AI-driven knowledge management.
Another challenge lies in the continuous updating and maintenance of knowledge repositories. As new knowledge is acquired and existing knowledge becomes outdated, organizations must implement effective mechanisms for knowledge validation, verification, and curation.
Looking ahead, the future of AI and knowledge management seems bright. With the advent of advanced AI technologies, organizations can harness the power of big data, unlock hidden knowledge, and enhance decision-making processes. However, it is crucial to approach the integration of AI and knowledge management with a responsible and ethical mindset, ensuring that these technologies are used to benefit individuals and society as a whole.
In conclusion, as we explore the fractals of AI and the potential of knowledge management in the AI era, it becomes evident that the future holds immense opportunities for synergistic growth. The integration of AI and knowledge management will reshape how organizations discover, capture, share, transfer, and apply knowledge. By leveraging AI technologies responsibly and addressing the associated challenges, organizations can unlock the full potential of their knowledge assets and drive innovation in the increasingly complex and interconnected world of artificial intelligence.
### 12.4 Final Thoughts
In this book, we explored the fascinating relationship between fractals and knowledge management in the era of artificial intelligence (AI). We delved into the concept of fractals and its significance in various fields, including AI and knowledge management. From the foundations of AI to the applications of fractals in AI systems, we examined the ways in which these interconnected disciplines can revolutionize the management and utilization of knowledge.
Throughout our exploration, we discovered that the fractal nature of AI and knowledge management is more than just a metaphor. Fractals, with their self-similarity and complexity at different scales, provide a profound framework for understanding and harnessing the power of AI in knowledge management.
At the heart of AI and knowledge management lies the extraction, organization, and application of knowledge. Knowledge is the lifeblood of any organization, and effective knowledge management is essential for innovation, decision-making, and problem-solving. However, the sheer volume and complexity of data make it increasingly challenging to extract valuable insights and transform them into actionable knowledge.
Fractals offer a unique lens through which we can approach this challenge. By recognizing the inherent patterns, structures, and relationships in data, we can leverage AI algorithms and techniques to efficiently collect, analyze, and aggregate knowledge. Fractal-based AI algorithms allow us to uncover hidden patterns, hierarchies, and emergent behaviors that traditional methods might overlook.
By applying fractal-based approaches, we can enhance the capabilities of knowledge management systems in several key areas. First, these approaches enable AI algorithms to better capture and represent knowledge. Fractals provide a means to encode complex and interconnected concepts in more scalable and efficient ways. They help in organizing knowledge hierarchically, facilitating better search and retrieval of information.
Additionally, fractal-based algorithms can generate AI-powered knowledge repositories that adapt and evolve over time. Just as fractals exhibit self-similarity, these repositories can grow and expand while maintaining coherence and relevance. This adaptability allows organizations to stay up-to-date with changing trends and dynamics in their domain.
Fractal visualization and analysis techniques further enhance our understanding and utilization of knowledge. Through AI-driven visualization techniques, we can transform complex data into visually comprehensible representations. Fractal patterns and structures can be visually explored, revealing insights and aiding decision-making.
Moreover, AI-based analysis of fractal patterns enables the identification of anomalies or outliers within datasets. Fractals provide a powerful tool for anomaly detection, allowing organizations to identify irregular patterns that may indicate fraud, errors, or other critical issues. By incorporating fractal-based predictive analytics, AI systems can also generate forecasts and predictions based on fractal insights, providing valuable insights for strategic decision-making.
While the potential of applying fractals to AI and knowledge management is immense, it is crucial to address the ethical considerations that arise in this context. As AI systems become more integrated into knowledge management processes, concerns around data privacy, security, and bias become paramount. Organizations must ensure that the use of AI in knowledge management respects individual rights, promotes fairness, and safeguards sensitive information.
To enhance human-AI collaboration in knowledge management, it is essential to consider the role of human expertise and judgment alongside AI-generated insights. AI algorithms can assist in the acquisition, organization, and application of knowledge, but human judgment and critical thinking are still invaluable. By fostering collaboration between AI and human experts, organizations can fully leverage the power of AI in knowledge management while capitalizing on human creativity and intuition.
Looking to the future, the convergence of AI and knowledge management holds great promise. As AI continues to advance and become more embedded in our daily lives, the need for effective knowledge management becomes even more crucial. By embracing fractal-inspired approaches, organizations can unlock the full potential of AI in harnessing knowledge, driving innovation, and gaining a competitive edge.
In conclusion, the exploration of fractals and their interplay with knowledge management in the era of AI reveals a vast landscape of possibilities. Fractals offer a captivating lens through which we can understand the complexity and self-similarity embedded within AI systems and knowledge management processes. By harnessing the power of fractals in AI, we can unleash the full potential of knowledge management, ultimately leading to a more insightful, collaborative, and innovative future.

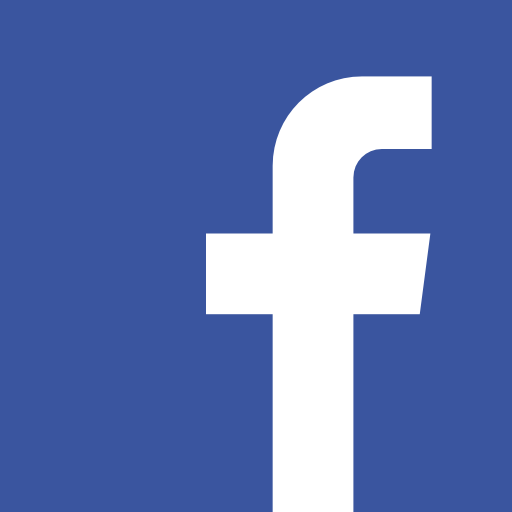
